کد مقاله | کد نشریه | سال انتشار | مقاله انگلیسی | نسخه تمام متن |
---|---|---|---|---|
530075 | 869740 | 2013 | 12 صفحه PDF | دانلود رایگان |
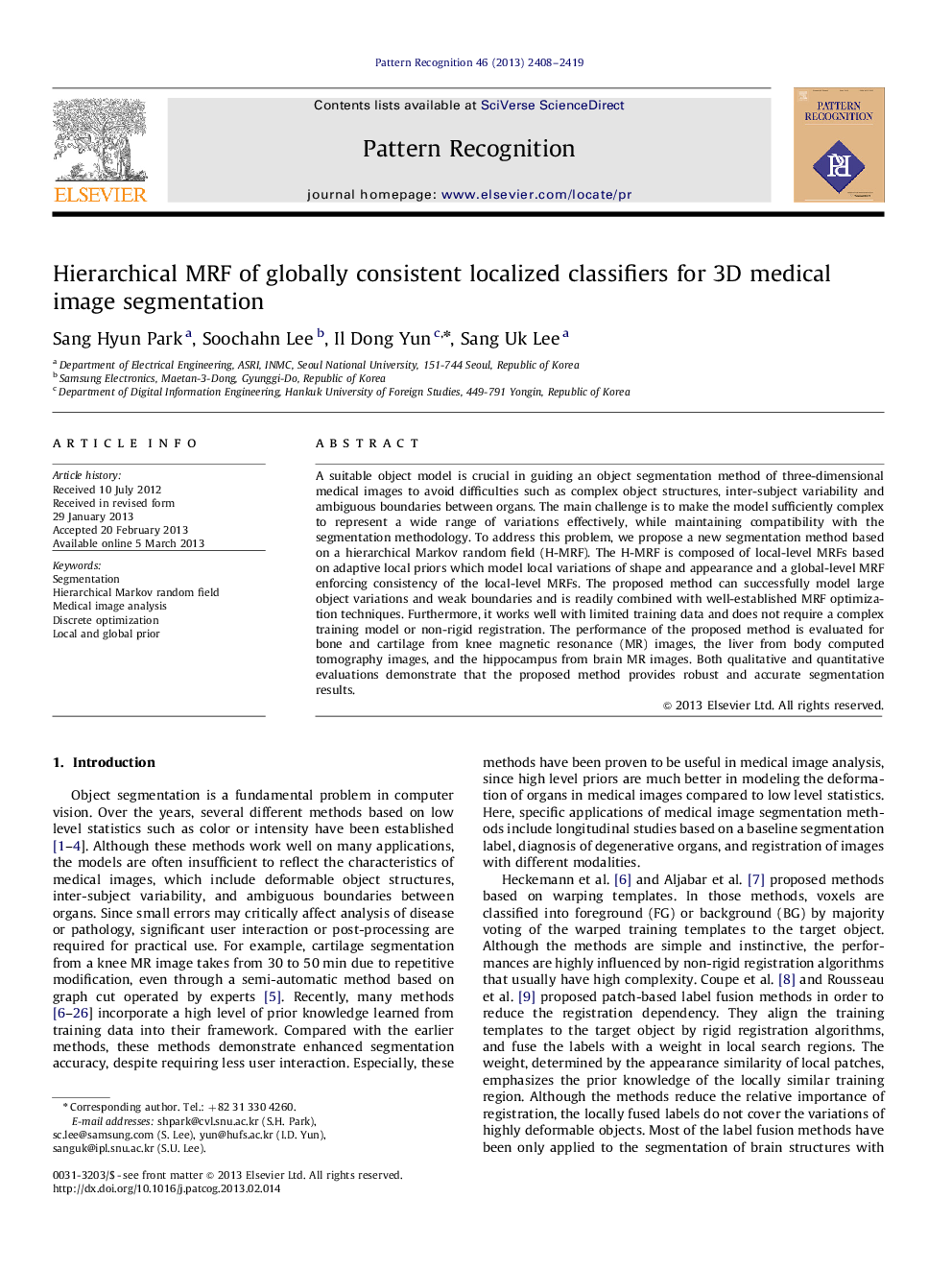
A suitable object model is crucial in guiding an object segmentation method of three-dimensional medical images to avoid difficulties such as complex object structures, inter-subject variability and ambiguous boundaries between organs. The main challenge is to make the model sufficiently complex to represent a wide range of variations effectively, while maintaining compatibility with the segmentation methodology. To address this problem, we propose a new segmentation method based on a hierarchical Markov random field (H-MRF). The H-MRF is composed of local-level MRFs based on adaptive local priors which model local variations of shape and appearance and a global-level MRF enforcing consistency of the local-level MRFs. The proposed method can successfully model large object variations and weak boundaries and is readily combined with well-established MRF optimization techniques. Furthermore, it works well with limited training data and does not require a complex training model or non-rigid registration. The performance of the proposed method is evaluated for bone and cartilage from knee magnetic resonance (MR) images, the liver from body computed tomography images, and the hippocampus from brain MR images. Both qualitative and quantitative evaluations demonstrate that the proposed method provides robust and accurate segmentation results.
► We present a new segmentation method based on a MRF from three-dimensional medical images.
► Hierarchical MRF, that simultaneously uses local and global prior, is proposed.
► Local prior covers variations by adaptively emphasizing shape and appearance priors.
► Global prior constrains overall smooth shape by relationships of local parts.
► The proposed method outperforms other MRF based methods for various organs.
Journal: Pattern Recognition - Volume 46, Issue 9, September 2013, Pages 2408–2419