کد مقاله | کد نشریه | سال انتشار | مقاله انگلیسی | نسخه تمام متن |
---|---|---|---|---|
531578 | 869856 | 2008 | 7 صفحه PDF | دانلود رایگان |
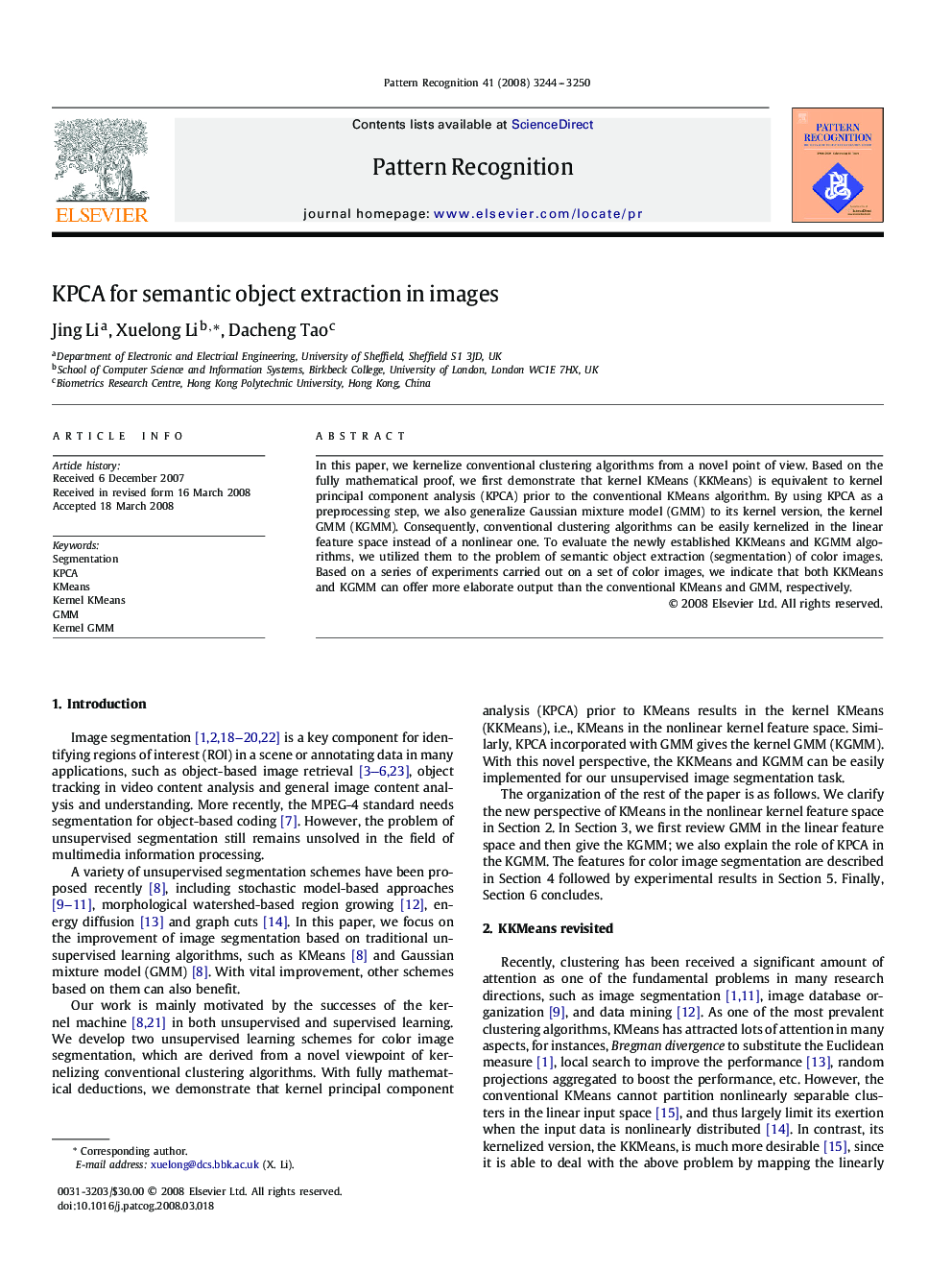
In this paper, we kernelize conventional clustering algorithms from a novel point of view. Based on the fully mathematical proof, we first demonstrate that kernel KMeans (KKMeans) is equivalent to kernel principal component analysis (KPCA) prior to the conventional KMeans algorithm. By using KPCA as a preprocessing step, we also generalize Gaussian mixture model (GMM) to its kernel version, the kernel GMM (KGMM). Consequently, conventional clustering algorithms can be easily kernelized in the linear feature space instead of a nonlinear one. To evaluate the newly established KKMeans and KGMM algorithms, we utilized them to the problem of semantic object extraction (segmentation) of color images. Based on a series of experiments carried out on a set of color images, we indicate that both KKMeans and KGMM can offer more elaborate output than the conventional KMeans and GMM, respectively.
Journal: Pattern Recognition - Volume 41, Issue 10, October 2008, Pages 3244–3250