کد مقاله | کد نشریه | سال انتشار | مقاله انگلیسی | نسخه تمام متن |
---|---|---|---|---|
532745 | 869989 | 2009 | 10 صفحه PDF | دانلود رایگان |
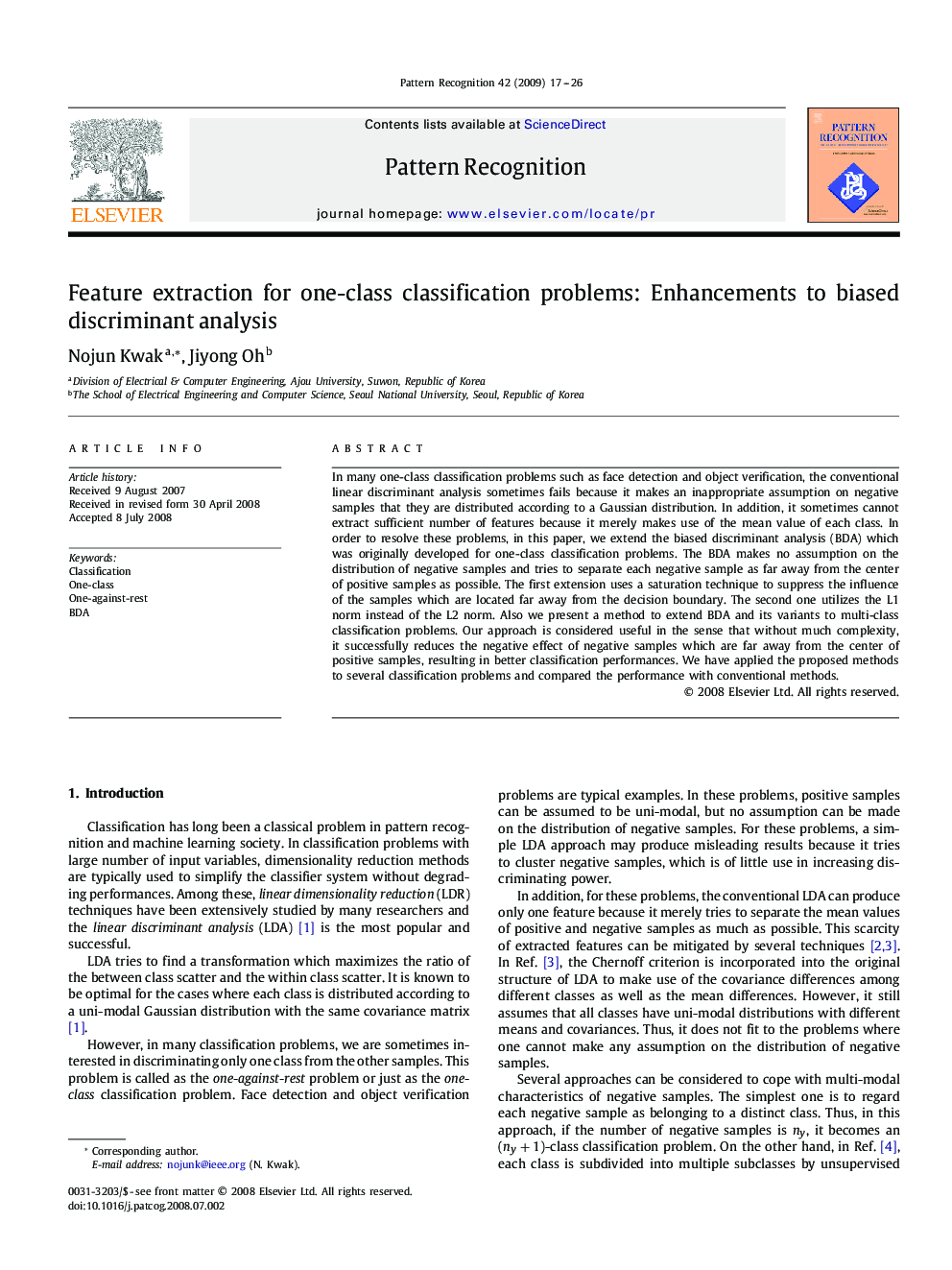
In many one-class classification problems such as face detection and object verification, the conventional linear discriminant analysis sometimes fails because it makes an inappropriate assumption on negative samples that they are distributed according to a Gaussian distribution. In addition, it sometimes cannot extract sufficient number of features because it merely makes use of the mean value of each class. In order to resolve these problems, in this paper, we extend the biased discriminant analysis (BDA) which was originally developed for one-class classification problems. The BDA makes no assumption on the distribution of negative samples and tries to separate each negative sample as far away from the center of positive samples as possible. The first extension uses a saturation technique to suppress the influence of the samples which are located far away from the decision boundary. The second one utilizes the L1 norm instead of the L2 norm. Also we present a method to extend BDA and its variants to multi-class classification problems. Our approach is considered useful in the sense that without much complexity, it successfully reduces the negative effect of negative samples which are far away from the center of positive samples, resulting in better classification performances. We have applied the proposed methods to several classification problems and compared the performance with conventional methods.
Journal: Pattern Recognition - Volume 42, Issue 1, January 2009, Pages 17–26