کد مقاله | کد نشریه | سال انتشار | مقاله انگلیسی | نسخه تمام متن |
---|---|---|---|---|
537005 | 870664 | 2012 | 11 صفحه PDF | دانلود رایگان |
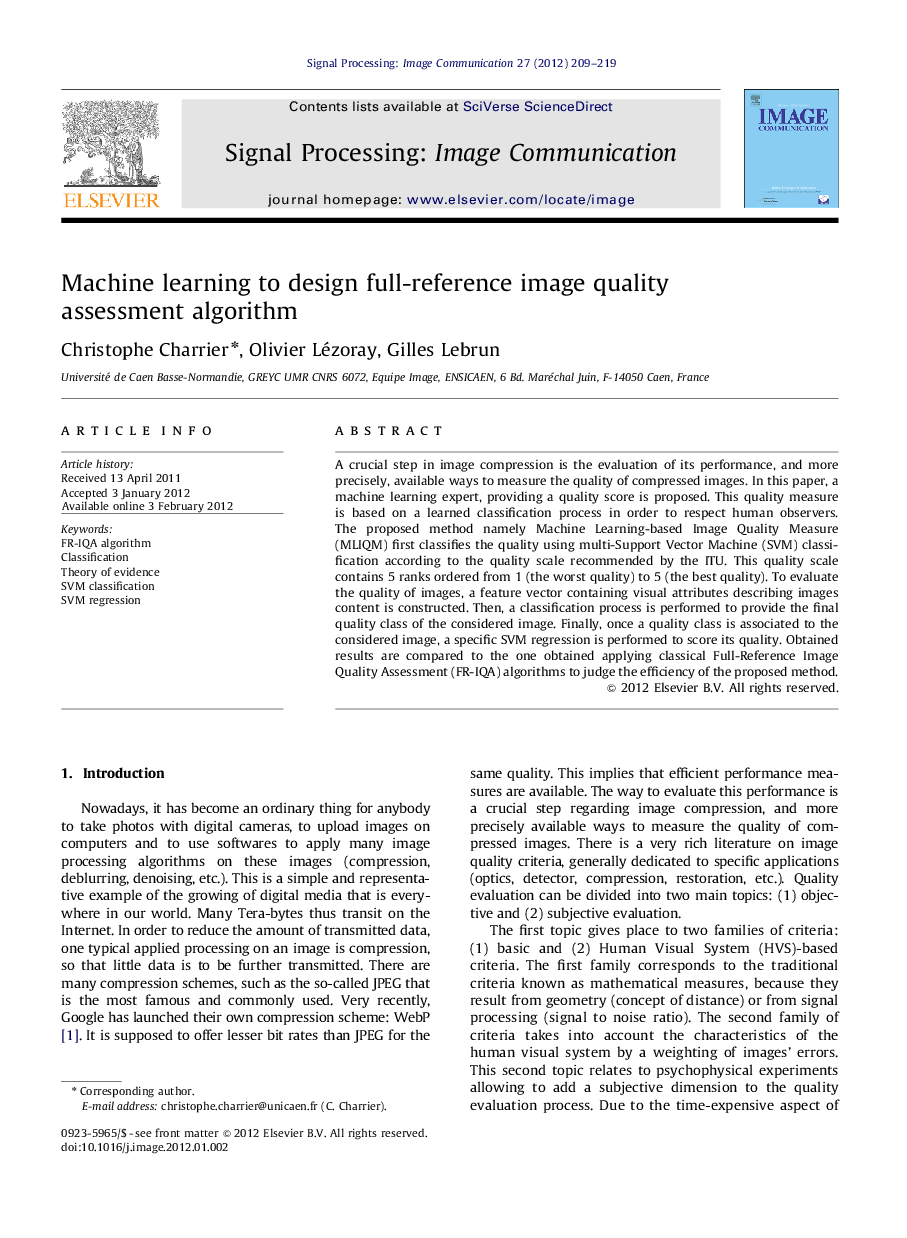
A crucial step in image compression is the evaluation of its performance, and more precisely, available ways to measure the quality of compressed images. In this paper, a machine learning expert, providing a quality score is proposed. This quality measure is based on a learned classification process in order to respect human observers. The proposed method namely Machine Learning-based Image Quality Measure (MLIQM) first classifies the quality using multi-Support Vector Machine (SVM) classification according to the quality scale recommended by the ITU. This quality scale contains 5 ranks ordered from 1 (the worst quality) to 5 (the best quality). To evaluate the quality of images, a feature vector containing visual attributes describing images content is constructed. Then, a classification process is performed to provide the final quality class of the considered image. Finally, once a quality class is associated to the considered image, a specific SVM regression is performed to score its quality. Obtained results are compared to the one obtained applying classical Full-Reference Image Quality Assessment (FR-IQA) algorithms to judge the efficiency of the proposed method.
► A machine learning expert providing a quality score is proposed.
► We examine changes in the level of information about hospital quality.
► SVM classification and SVM regression yields to design efficient full-reference image quality assessment algorithm.
► Correlation with human ratings is consistent and outperforms state-of-the-art techniques.
Journal: Signal Processing: Image Communication - Volume 27, Issue 3, March 2012, Pages 209–219