کد مقاله | کد نشریه | سال انتشار | مقاله انگلیسی | نسخه تمام متن |
---|---|---|---|---|
5532881 | 1402084 | 2017 | 8 صفحه PDF | دانلود رایگان |
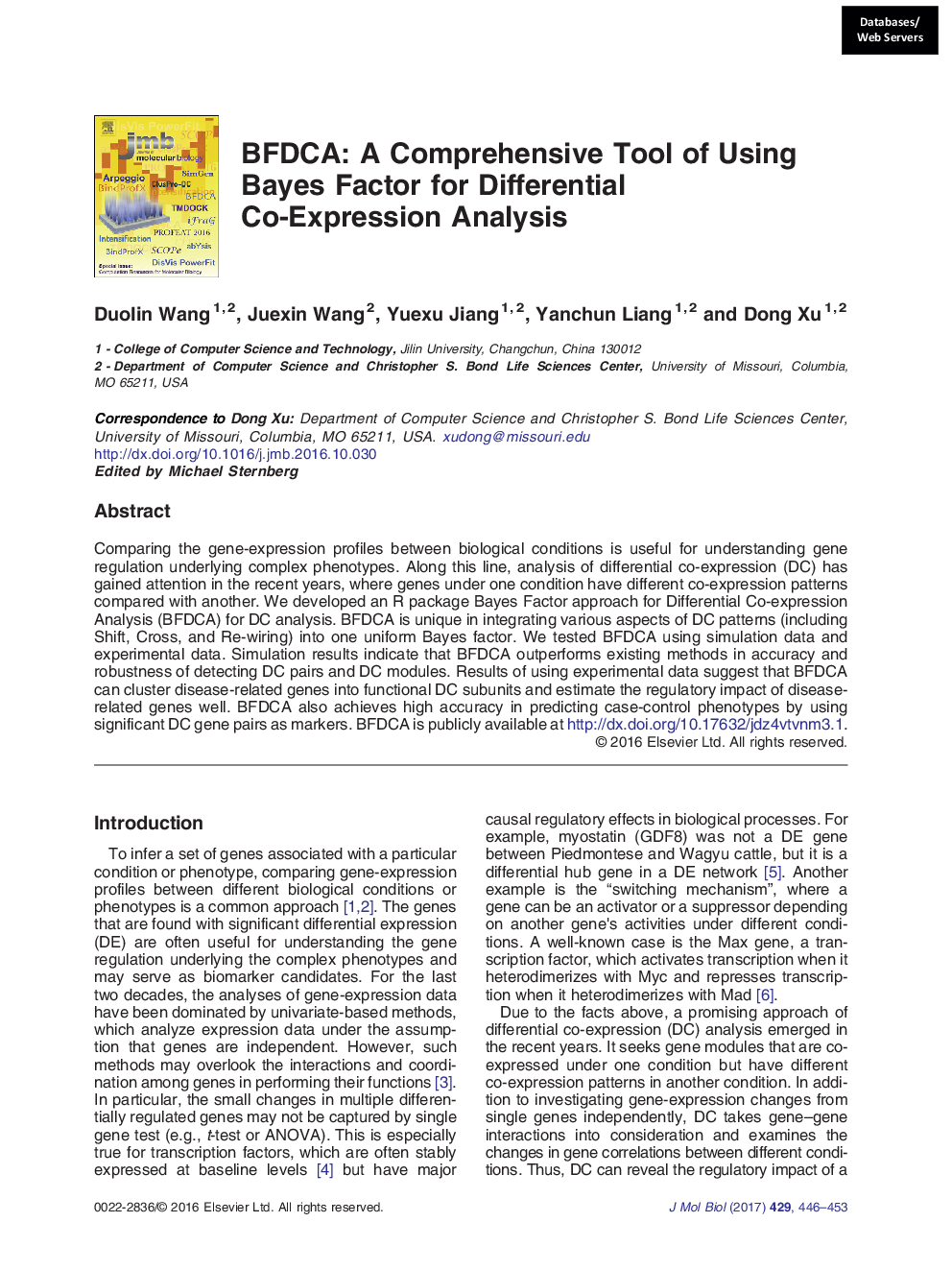
- Differential co-expression (DC) analysis is useful in understanding functional and regulatory relationships among genes, but few tools are available for effective DC analysis.
- By integrating different aspects of DC patterns into a uniform Bayes factor, Bayes Factor approach for Differential Co-expression Analysis (BFDCA) can estimate DC between two conditions with high sensitivity.
- BFDCA clusters condition-specific genes into functional DC subunits and quantitatively characterizes their regulatory impact on genes.
- BFDCA identifies significant DC gene pairs and achieves high accuracy in predicting case/control phenotypes by using these gene pairs as markers.
- BFDCA is implemented in a general R package, which can be easily used by biological researchers and generate biologically meaningful hypotheses.
Comparing the gene-expression profiles between biological conditions is useful for understanding gene regulation underlying complex phenotypes. Along this line, analysis of differential co-expression (DC) has gained attention in the recent years, where genes under one condition have different co-expression patterns compared with another. We developed an R package Bayes Factor approach for Differential Co-expression Analysis (BFDCA) for DC analysis. BFDCA is unique in integrating various aspects of DC patterns (including Shift, Cross, and Re-wiring) into one uniform Bayes factor. We tested BFDCA using simulation data and experimental data. Simulation results indicate that BFDCA outperforms existing methods in accuracy and robustness of detecting DC pairs and DC modules. Results of using experimental data suggest that BFDCA can cluster disease-related genes into functional DC subunits and estimate the regulatory impact of disease-related genes well. BFDCA also achieves high accuracy in predicting case-control phenotypes by using significant DC gene pairs as markers. BFDCA is publicly available at http://dx.doi.org/10.17632/jdz4vtvnm3.1.
Graphical Abstract335
Journal: Journal of Molecular Biology - Volume 429, Issue 3, 3 February 2017, Pages 446-453