کد مقاله | کد نشریه | سال انتشار | مقاله انگلیسی | نسخه تمام متن |
---|---|---|---|---|
558328 | 874902 | 2013 | 20 صفحه PDF | دانلود رایگان |
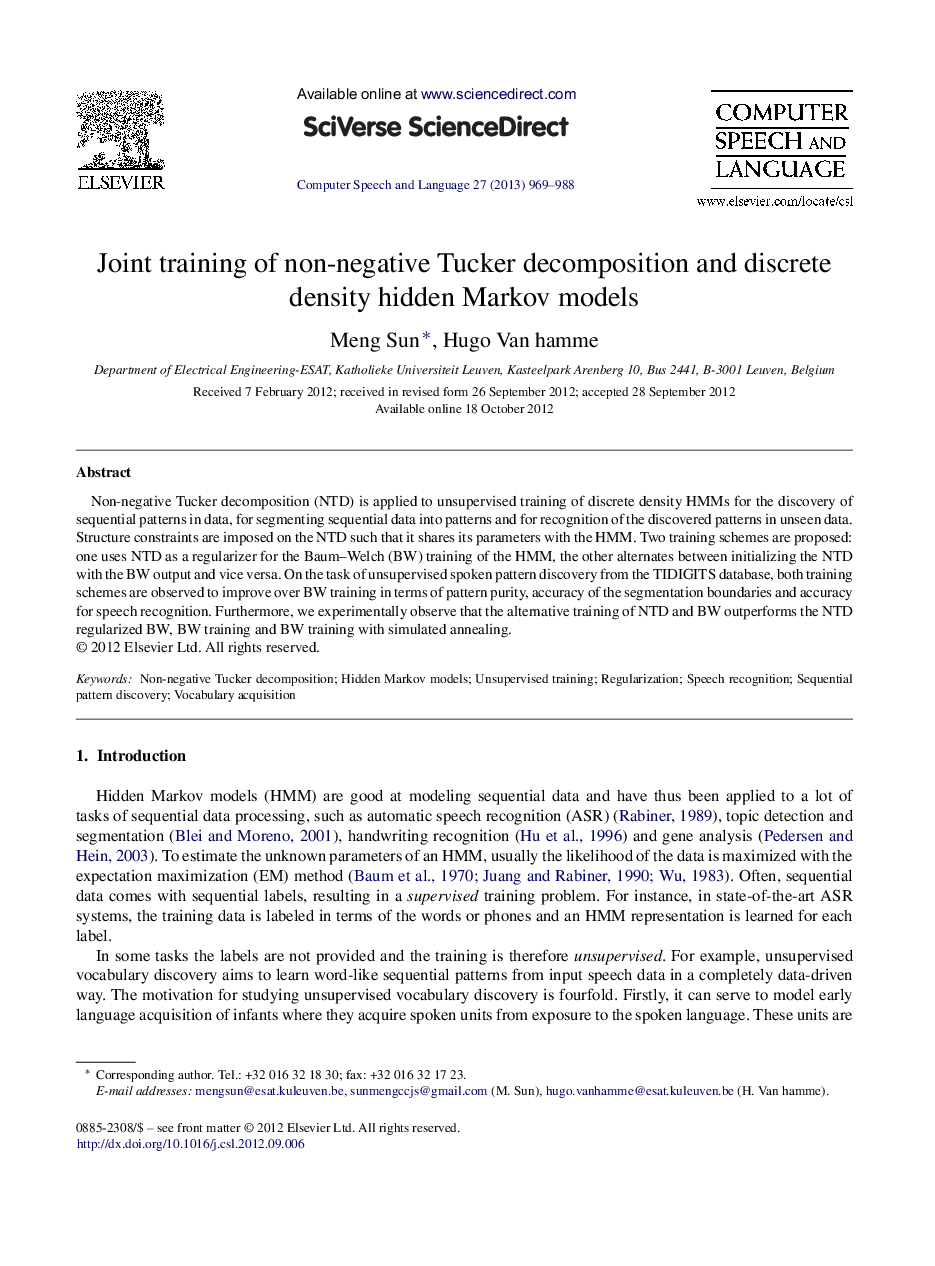
Non-negative Tucker decomposition (NTD) is applied to unsupervised training of discrete density HMMs for the discovery of sequential patterns in data, for segmenting sequential data into patterns and for recognition of the discovered patterns in unseen data. Structure constraints are imposed on the NTD such that it shares its parameters with the HMM. Two training schemes are proposed: one uses NTD as a regularizer for the Baum–Welch (BW) training of the HMM, the other alternates between initializing the NTD with the BW output and vice versa. On the task of unsupervised spoken pattern discovery from the TIDIGITS database, both training schemes are observed to improve over BW training in terms of pattern purity, accuracy of the segmentation boundaries and accuracy for speech recognition. Furthermore, we experimentally observe that the alternative training of NTD and BW outperforms the NTD regularized BW, BW training and BW training with simulated annealing.
► New methods of unsupervised training of discrete density HMMs.
► An unified framework to discover sequential patterns from unlabeled data.
► Good performance on unsupervised spoken pattern discovery.
Journal: Computer Speech & Language - Volume 27, Issue 4, June 2013, Pages 969–988