کد مقاله | کد نشریه | سال انتشار | مقاله انگلیسی | نسخه تمام متن |
---|---|---|---|---|
559094 | 875048 | 2011 | 18 صفحه PDF | دانلود رایگان |
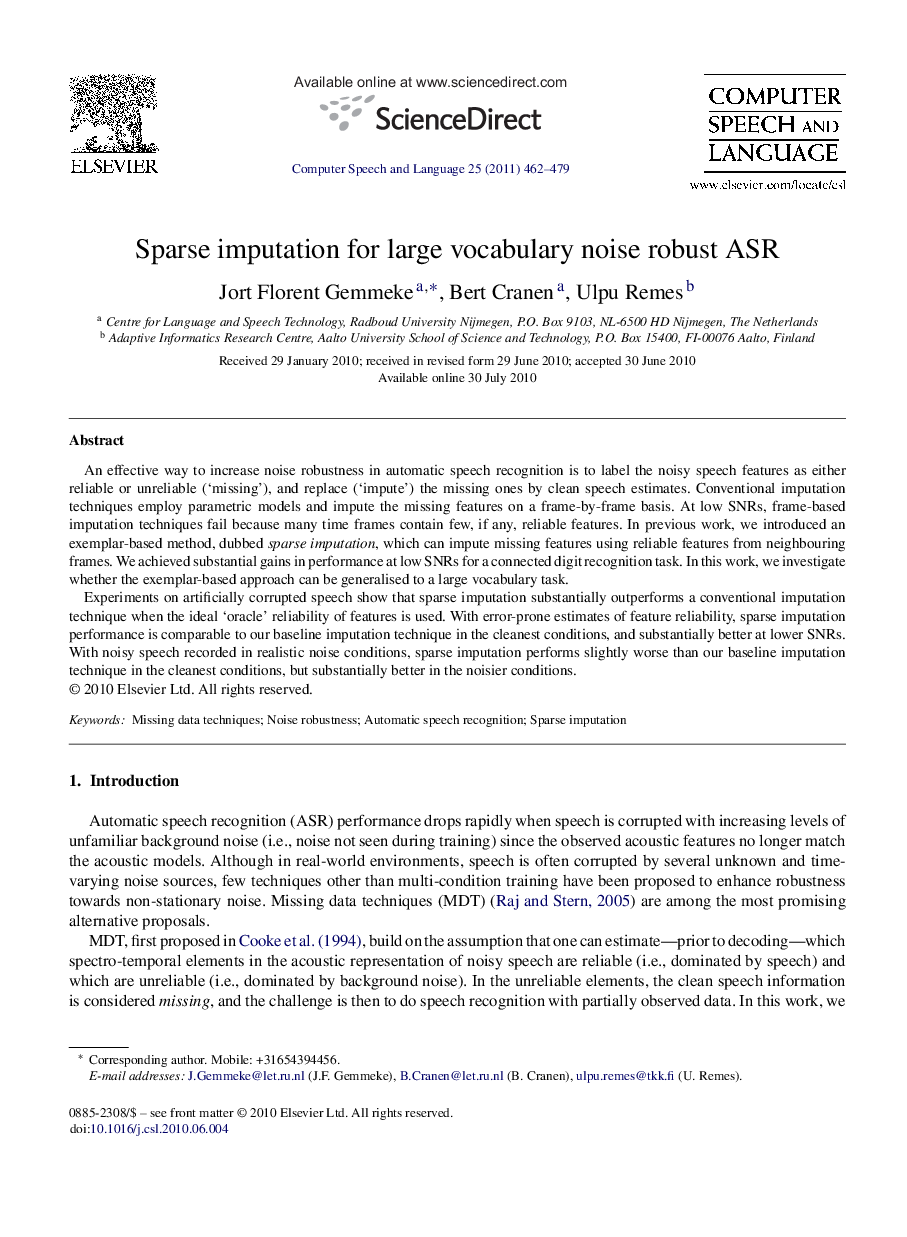
An effective way to increase noise robustness in automatic speech recognition is to label the noisy speech features as either reliable or unreliable (‘missing’), and replace (‘impute’) the missing ones by clean speech estimates. Conventional imputation techniques employ parametric models and impute the missing features on a frame-by-frame basis. At low SNRs, frame-based imputation techniques fail because many time frames contain few, if any, reliable features. In previous work, we introduced an exemplar-based method, dubbed sparse imputation, which can impute missing features using reliable features from neighbouring frames. We achieved substantial gains in performance at low SNRs for a connected digit recognition task. In this work, we investigate whether the exemplar-based approach can be generalised to a large vocabulary task.Experiments on artificially corrupted speech show that sparse imputation substantially outperforms a conventional imputation technique when the ideal ‘oracle’ reliability of features is used. With error-prone estimates of feature reliability, sparse imputation performance is comparable to our baseline imputation technique in the cleanest conditions, and substantially better at lower SNRs. With noisy speech recorded in realistic noise conditions, sparse imputation performs slightly worse than our baseline imputation technique in the cleanest conditions, but substantially better in the noisier conditions.
Journal: Computer Speech & Language - Volume 25, Issue 2, April 2011, Pages 462–479