کد مقاله | کد نشریه | سال انتشار | مقاله انگلیسی | نسخه تمام متن |
---|---|---|---|---|
561472 | 1451886 | 2012 | 15 صفحه PDF | دانلود رایگان |
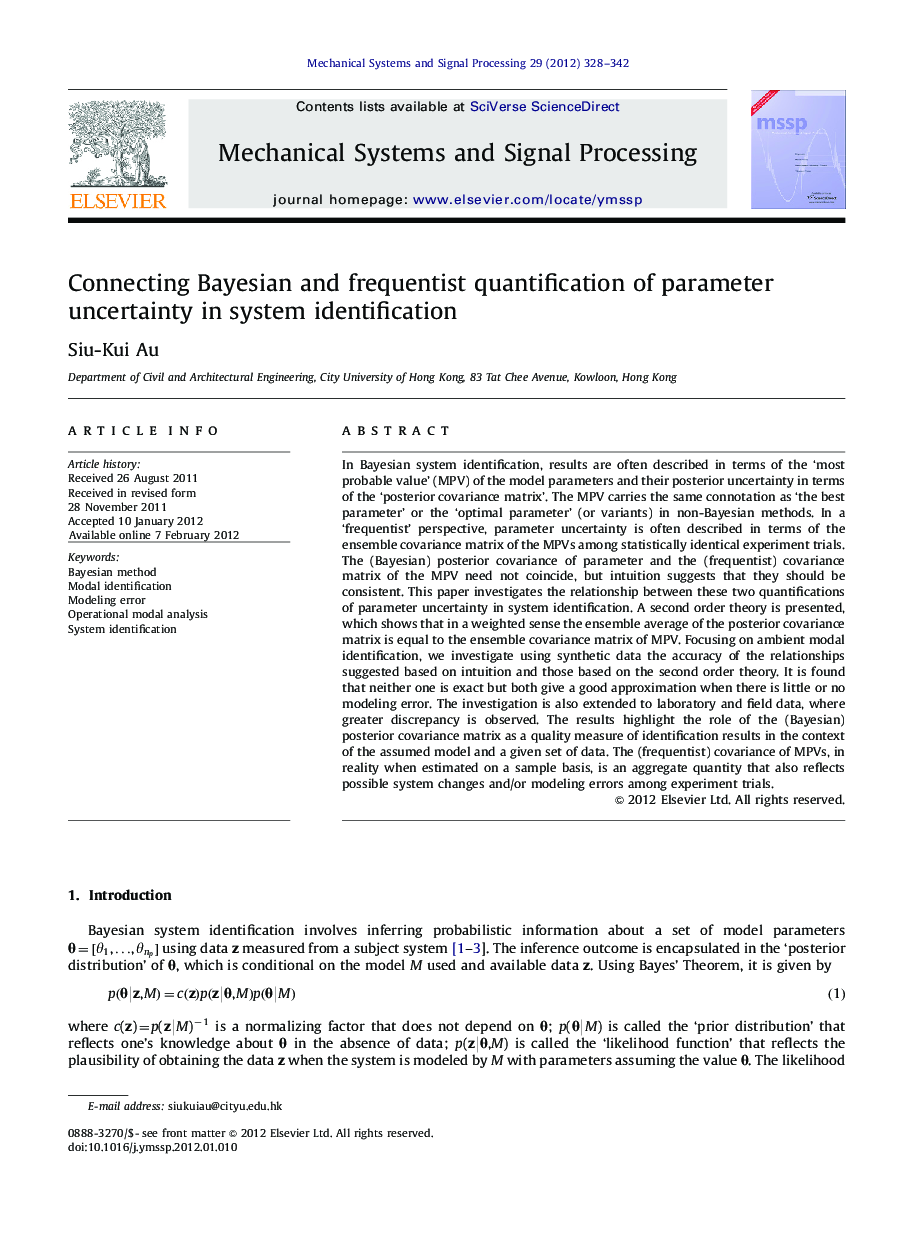
In Bayesian system identification, results are often described in terms of the ‘most probable value’ (MPV) of the model parameters and their posterior uncertainty in terms of the ‘posterior covariance matrix’. The MPV carries the same connotation as ‘the best parameter’ or the ‘optimal parameter’ (or variants) in non-Bayesian methods. In a ‘frequentist’ perspective, parameter uncertainty is often described in terms of the ensemble covariance matrix of the MPVs among statistically identical experiment trials. The (Bayesian) posterior covariance of parameter and the (frequentist) covariance matrix of the MPV need not coincide, but intuition suggests that they should be consistent. This paper investigates the relationship between these two quantifications of parameter uncertainty in system identification. A second order theory is presented, which shows that in a weighted sense the ensemble average of the posterior covariance matrix is equal to the ensemble covariance matrix of MPV. Focusing on ambient modal identification, we investigate using synthetic data the accuracy of the relationships suggested based on intuition and those based on the second order theory. It is found that neither one is exact but both give a good approximation when there is little or no modeling error. The investigation is also extended to laboratory and field data, where greater discrepancy is observed. The results highlight the role of the (Bayesian) posterior covariance matrix as a quality measure of identification results in the context of the assumed model and a given set of data. The (frequentist) covariance of MPVs, in reality when estimated on a sample basis, is an aggregate quantity that also reflects possible system changes and/or modeling errors among experiment trials.
► A second order theory shows the Bayesian and frequentist measure of parameter uncertainty are equal in a weighted sense.
► The theory is further investigated using synthetic, laboratory and field data.
► The total variability across experiments is shown as a sum of Bayesian and frequentist measure.
► This work gives a unifying perspective on the meaning and use of Bayesian and frequentist measure.
Journal: Mechanical Systems and Signal Processing - Volume 29, May 2012, Pages 328–342