کد مقاله | کد نشریه | سال انتشار | مقاله انگلیسی | نسخه تمام متن |
---|---|---|---|---|
561478 | 1451886 | 2012 | 13 صفحه PDF | دانلود رایگان |
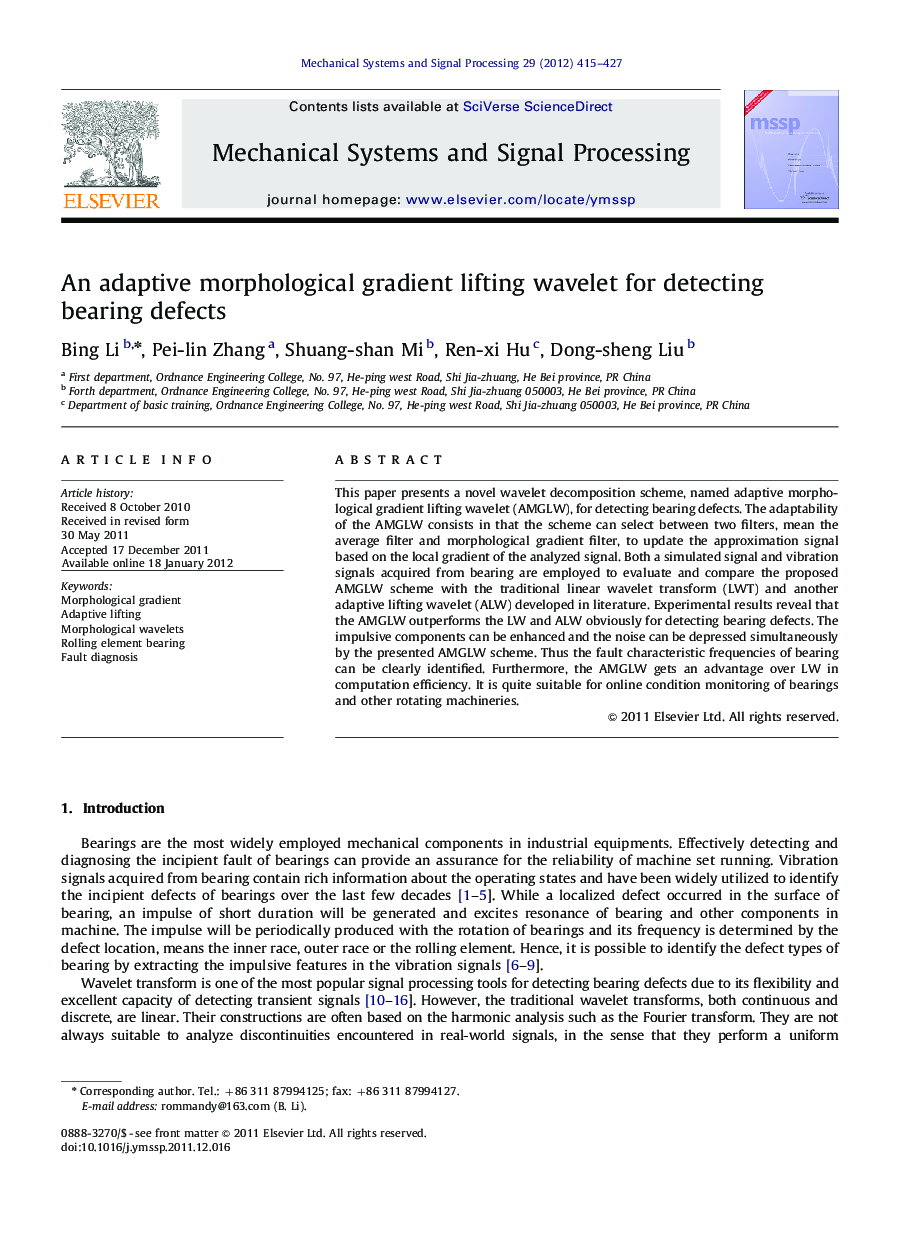
This paper presents a novel wavelet decomposition scheme, named adaptive morphological gradient lifting wavelet (AMGLW), for detecting bearing defects. The adaptability of the AMGLW consists in that the scheme can select between two filters, mean the average filter and morphological gradient filter, to update the approximation signal based on the local gradient of the analyzed signal. Both a simulated signal and vibration signals acquired from bearing are employed to evaluate and compare the proposed AMGLW scheme with the traditional linear wavelet transform (LWT) and another adaptive lifting wavelet (ALW) developed in literature. Experimental results reveal that the AMGLW outperforms the LW and ALW obviously for detecting bearing defects. The impulsive components can be enhanced and the noise can be depressed simultaneously by the presented AMGLW scheme. Thus the fault characteristic frequencies of bearing can be clearly identified. Furthermore, the AMGLW gets an advantage over LW in computation efficiency. It is quite suitable for online condition monitoring of bearings and other rotating machineries.
► A novel adaptive morphological gradient lifting wavelet (AMGLW) is proposed.
► The impulsive components can be enhanced and the noise can be depressed simultaneously by AMGLW.
► The AMGLW gets an advantage over the tradition linear wavelet transforms in computation efficiency.
► Experimental results show the superiority of AMGLW over the tradition linear wavelet transforms.
Journal: Mechanical Systems and Signal Processing - Volume 29, May 2012, Pages 415–427