کد مقاله | کد نشریه | سال انتشار | مقاله انگلیسی | نسخه تمام متن |
---|---|---|---|---|
562439 | 1451953 | 2015 | 14 صفحه PDF | دانلود رایگان |
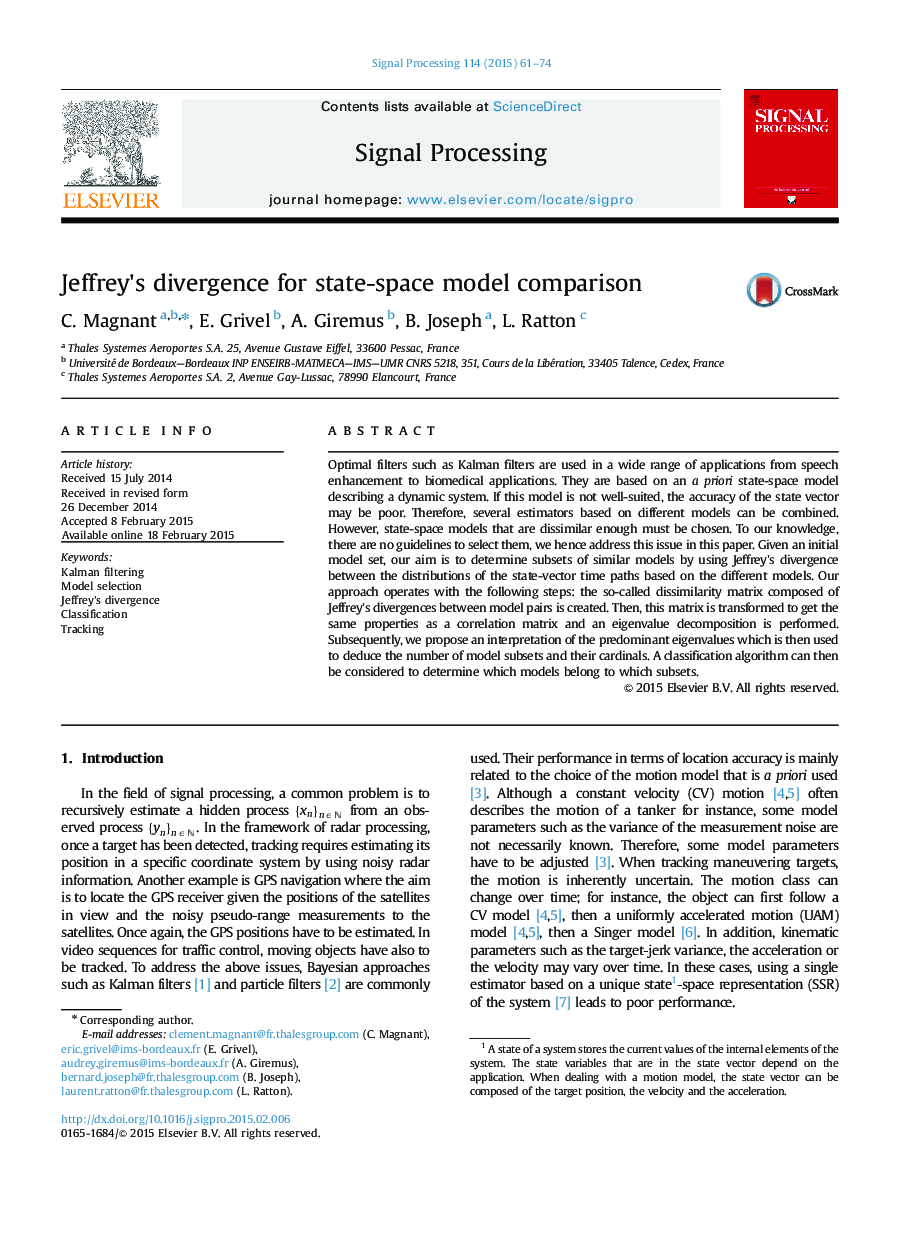
• We propose to compare two state models by using the Jeffrey's divergence (JD).
• We generalize the above approach to determine subsets in an initial model set.
• The eigenvalues decomposition is then interpreted to deduce the number of model subsets.
• Then, a classification algorithm can be used to determine which model belongs to which subset.
• The method is more particularly applied to a toy example and to tracking.
Optimal filters such as Kalman filters are used in a wide range of applications from speech enhancement to biomedical applications. They are based on an a priori state-space model describing a dynamic system. If this model is not well-suited, the accuracy of the state vector may be poor. Therefore, several estimators based on different models can be combined. However, state-space models that are dissimilar enough must be chosen. To our knowledge, there are no guidelines to select them, we hence address this issue in this paper. Given an initial model set, our aim is to determine subsets of similar models by using Jeffrey׳s divergence between the distributions of the state-vector time paths based on the different models. Our approach operates with the following steps: the so-called dissimilarity matrix composed of Jeffrey׳s divergences between model pairs is created. Then, this matrix is transformed to get the same properties as a correlation matrix and an eigenvalue decomposition is performed. Subsequently, we propose an interpretation of the predominant eigenvalues which is then used to deduce the number of model subsets and their cardinals. A classification algorithm can then be considered to determine which models belong to which subsets.
Journal: Signal Processing - Volume 114, September 2015, Pages 61–74