کد مقاله | کد نشریه | سال انتشار | مقاله انگلیسی | نسخه تمام متن |
---|---|---|---|---|
564694 | 1451749 | 2014 | 9 صفحه PDF | دانلود رایگان |
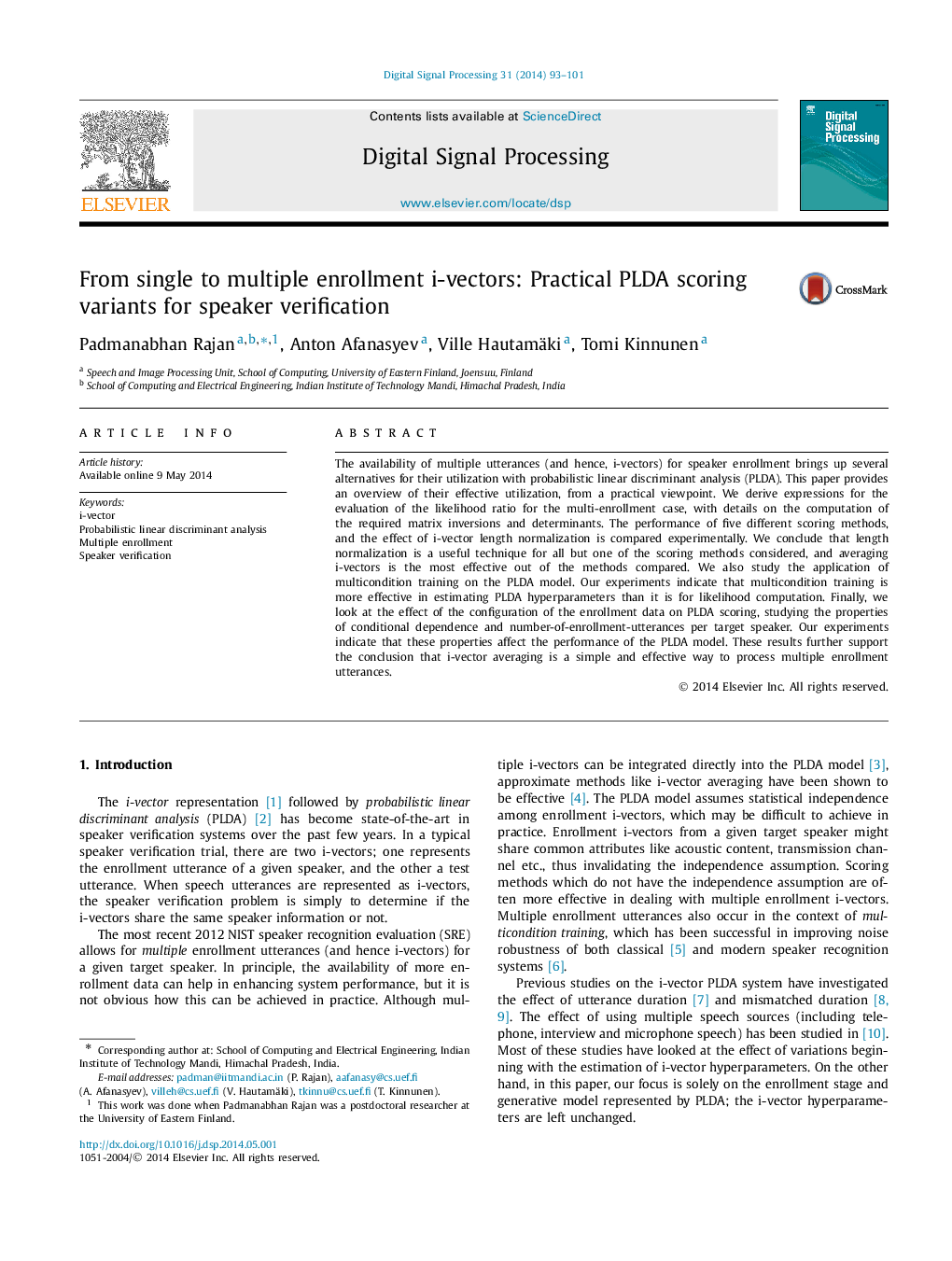
The availability of multiple utterances (and hence, i-vectors) for speaker enrollment brings up several alternatives for their utilization with probabilistic linear discriminant analysis (PLDA). This paper provides an overview of their effective utilization, from a practical viewpoint. We derive expressions for the evaluation of the likelihood ratio for the multi-enrollment case, with details on the computation of the required matrix inversions and determinants. The performance of five different scoring methods, and the effect of i-vector length normalization is compared experimentally. We conclude that length normalization is a useful technique for all but one of the scoring methods considered, and averaging i-vectors is the most effective out of the methods compared. We also study the application of multicondition training on the PLDA model. Our experiments indicate that multicondition training is more effective in estimating PLDA hyperparameters than it is for likelihood computation. Finally, we look at the effect of the configuration of the enrollment data on PLDA scoring, studying the properties of conditional dependence and number-of-enrollment-utterances per target speaker. Our experiments indicate that these properties affect the performance of the PLDA model. These results further support the conclusion that i-vector averaging is a simple and effective way to process multiple enrollment utterances.
Journal: Digital Signal Processing - Volume 31, August 2014, Pages 93–101