کد مقاله | کد نشریه | سال انتشار | مقاله انگلیسی | نسخه تمام متن |
---|---|---|---|---|
564697 | 1451749 | 2014 | 12 صفحه PDF | دانلود رایگان |
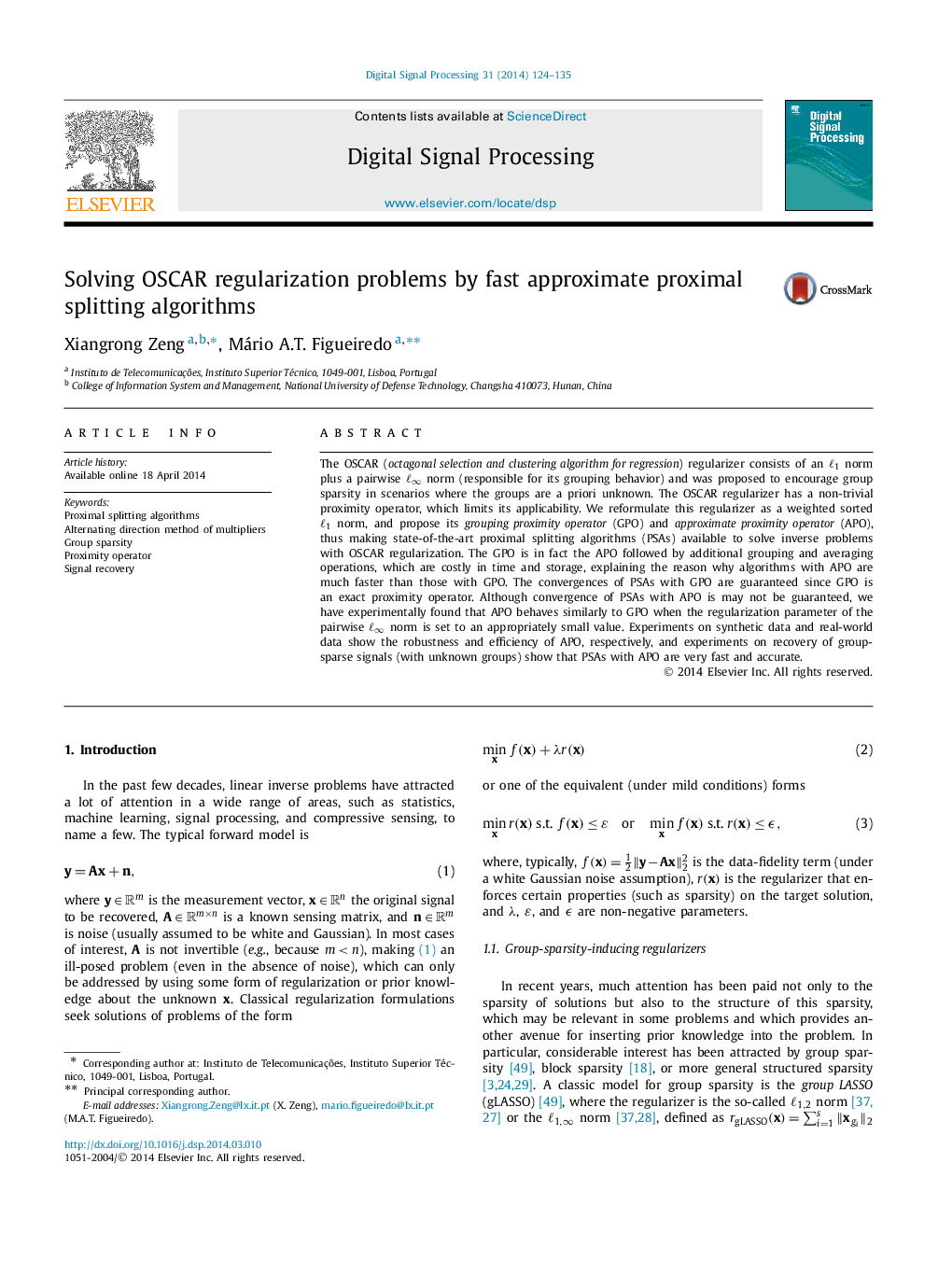
The OSCAR (octagonal selection and clustering algorithm for regression ) regularizer consists of an ℓ1ℓ1 norm plus a pairwise ℓ∞ℓ∞ norm (responsible for its grouping behavior) and was proposed to encourage group sparsity in scenarios where the groups are a priori unknown. The OSCAR regularizer has a non-trivial proximity operator, which limits its applicability. We reformulate this regularizer as a weighted sorted ℓ1ℓ1 norm, and propose its grouping proximity operator (GPO) and approximate proximity operator (APO), thus making state-of-the-art proximal splitting algorithms (PSAs) available to solve inverse problems with OSCAR regularization. The GPO is in fact the APO followed by additional grouping and averaging operations, which are costly in time and storage, explaining the reason why algorithms with APO are much faster than those with GPO. The convergences of PSAs with GPO are guaranteed since GPO is an exact proximity operator. Although convergence of PSAs with APO is may not be guaranteed, we have experimentally found that APO behaves similarly to GPO when the regularization parameter of the pairwise ℓ∞ℓ∞ norm is set to an appropriately small value. Experiments on synthetic data and real-world data show the robustness and efficiency of APO, respectively, and experiments on recovery of group-sparse signals (with unknown groups) show that PSAs with APO are very fast and accurate.
Journal: Digital Signal Processing - Volume 31, August 2014, Pages 124–135