کد مقاله | کد نشریه | سال انتشار | مقاله انگلیسی | نسخه تمام متن |
---|---|---|---|---|
5754729 | 1621200 | 2017 | 13 صفحه PDF | دانلود رایگان |
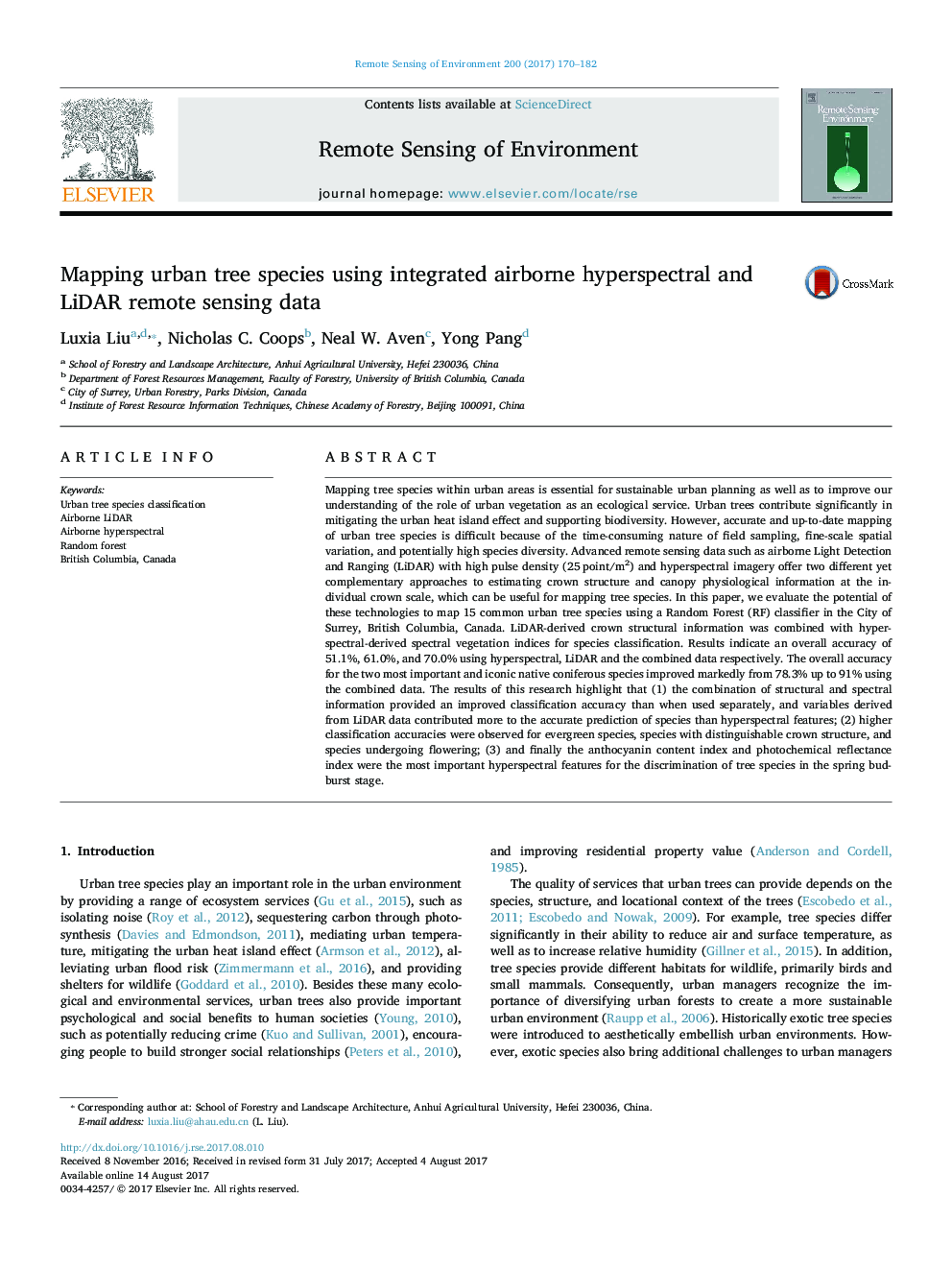
- Fused LiDAR and hyperspectral data are used to map fifteen urban tree species.
- Overall accuracy for each species ranged from 51 to 70%.
- Overall accuracy for two iconic native coniferous species was 78-91%.
- LiDAR contributed more to the accurate prediction of species than hyperspectral features.
- Two leaf pigment indices were the most important hyperspectral features.
Mapping tree species within urban areas is essential for sustainable urban planning as well as to improve our understanding of the role of urban vegetation as an ecological service. Urban trees contribute significantly in mitigating the urban heat island effect and supporting biodiversity. However, accurate and up-to-date mapping of urban tree species is difficult because of the time-consuming nature of field sampling, fine-scale spatial variation, and potentially high species diversity. Advanced remote sensing data such as airborne Light Detection and Ranging (LiDAR) with high pulse density (25Â point/m2) and hyperspectral imagery offer two different yet complementary approaches to estimating crown structure and canopy physiological information at the individual crown scale, which can be useful for mapping tree species. In this paper, we evaluate the potential of these technologies to map 15 common urban tree species using a Random Forest (RF) classifier in the City of Surrey, British Columbia, Canada. LiDAR-derived crown structural information was combined with hyperspectral-derived spectral vegetation indices for species classification. Results indicate an overall accuracy of 51.1%, 61.0%, and 70.0% using hyperspectral, LiDAR and the combined data respectively. The overall accuracy for the two most important and iconic native coniferous species improved markedly from 78.3% up to 91% using the combined data. The results of this research highlight that (1) the combination of structural and spectral information provided an improved classification accuracy than when used separately, and variables derived from LiDAR data contributed more to the accurate prediction of species than hyperspectral features; (2) higher classification accuracies were observed for evergreen species, species with distinguishable crown structure, and species undergoing flowering; (3) and finally the anthocyanin content index and photochemical reflectance index were the most important hyperspectral features for the discrimination of tree species in the spring budburst stage.
Journal: Remote Sensing of Environment - Volume 200, October 2017, Pages 170-182