کد مقاله | کد نشریه | سال انتشار | مقاله انگلیسی | نسخه تمام متن |
---|---|---|---|---|
5769958 | 1629202 | 2017 | 8 صفحه PDF | دانلود رایگان |
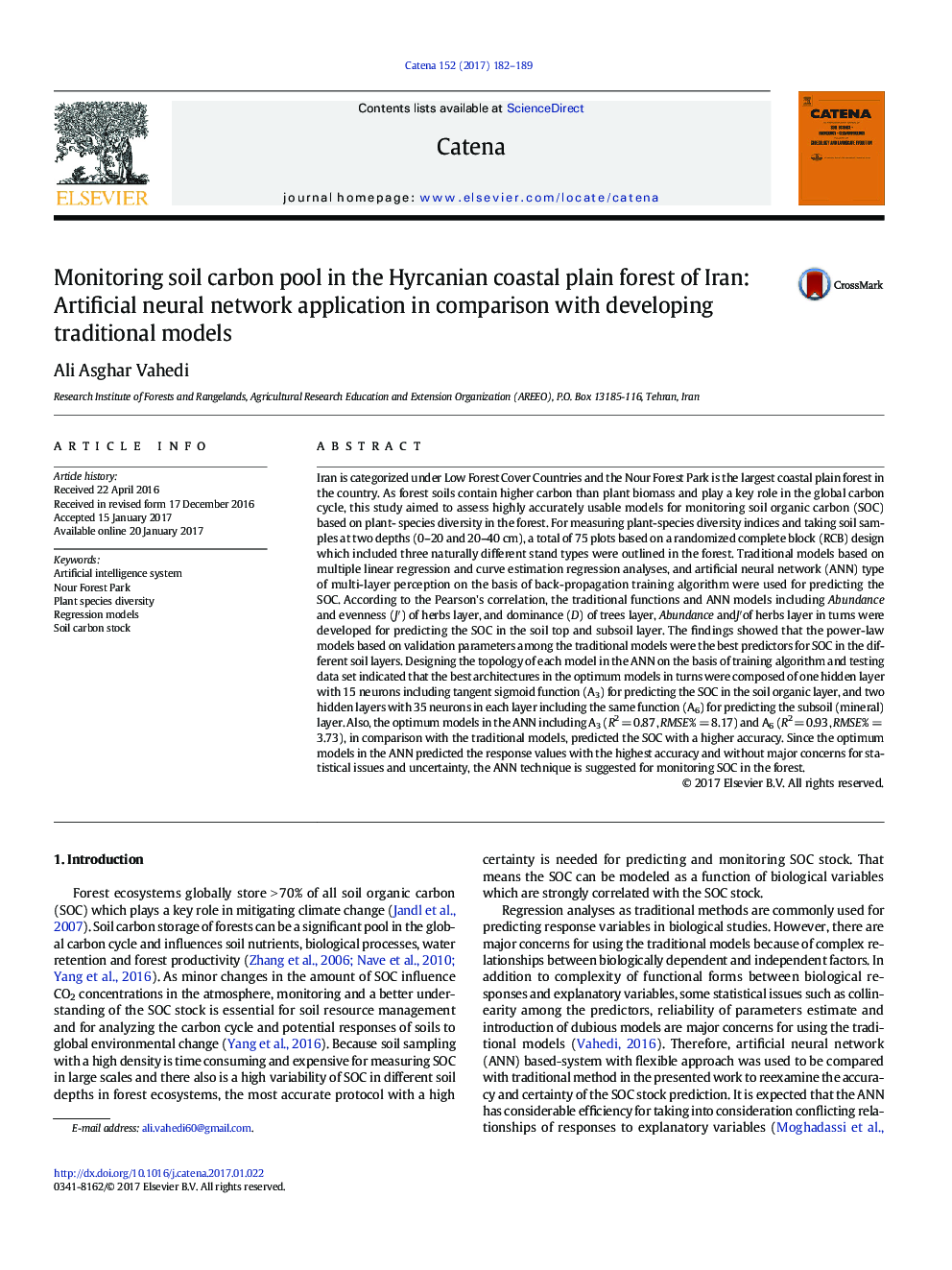
- This study developed a new protocol for monitoring SOC stock in a natural forest.
- Regression analyses compared to artificial neural network (ANN) technique were used for monitoring SOC stock.
- The SOC stock could serve as function of plant-species diversity indices in the models.
- The power-law models were the best traditional predictors for monitoring SOC stock.
- The ANN technique led to most accurate predictions for monitoring the SOC stock.
Iran is categorized under Low Forest Cover Countries and the Nour Forest Park is the largest coastal plain forest in the country. As forest soils contain higher carbon than plant biomass and play a key role in the global carbon cycle, this study aimed to assess highly accurately usable models for monitoring soil organic carbon (SOC) based on plant- species diversity in the forest. For measuring plant-species diversity indices and taking soil samples at two depths (0-20 and 20-40 cm), a total of 75 plots based on a randomized complete block (RCB) design which included three naturally different stand types were outlined in the forest. Traditional models based on multiple linear regression and curve estimation regression analyses, and artificial neural network (ANN) type of multi-layer perception on the basis of back-propagation training algorithm were used for predicting the SOC. According to the Pearson's correlation, the traditional functions and ANN models including Abundance and evenness (Jâ²) of herbs layer, and dominance (D) of trees layer, Abundance andJâ²of herbs layer in turns were developed for predicting the SOC in the soil top and subsoil layer. The findings showed that the power-law models based on validation parameters among the traditional models were the best predictors for SOC in the different soil layers. Designing the topology of each model in the ANN on the basis of training algorithm and testing data set indicated that the best architectures in the optimum models in turns were composed of one hidden layer with 15 neurons including tangent sigmoid function (A3) for predicting the SOC in the soil organic layer, and two hidden layers with 35 neurons in each layer including the same function (A6) for predicting the subsoil (mineral) layer. Also, the optimum models in the ANN including A3 (R2 = 0.87 , RMSE % = 8.17) and A6 (R2 = 0.93 , RMSE % = 3.73), in comparison with the traditional models, predicted the SOC with a higher accuracy. Since the optimum models in the ANN predicted the response values with the highest accuracy and without major concerns for statistical issues and uncertainty, the ANN technique is suggested for monitoring SOC in the forest.
84
Journal: CATENA - Volume 152, May 2017, Pages 182-189