کد مقاله | کد نشریه | سال انتشار | مقاله انگلیسی | نسخه تمام متن |
---|---|---|---|---|
6854939 | 1437600 | 2018 | 32 صفحه PDF | دانلود رایگان |
عنوان انگلیسی مقاله ISI
EEG signal classification using universum support vector machine
دانلود مقاله + سفارش ترجمه
دانلود مقاله ISI انگلیسی
رایگان برای ایرانیان
کلمات کلیدی
موضوعات مرتبط
مهندسی و علوم پایه
مهندسی کامپیوتر
هوش مصنوعی
پیش نمایش صفحه اول مقاله
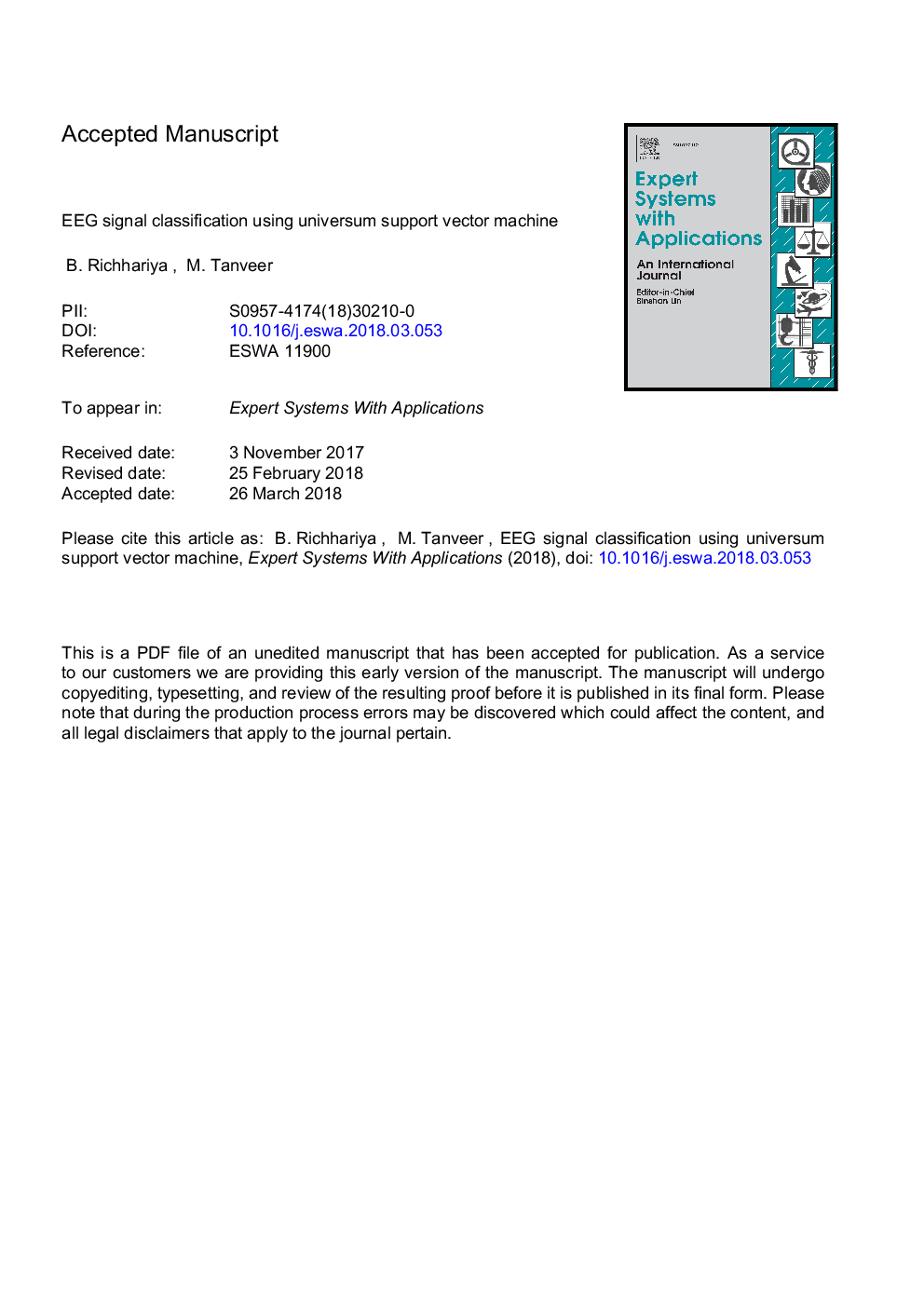
چکیده انگلیسی
Support vector machine (SVM) has been used widely for classification of electroencephalogram (EEG) signals for the diagnosis of neurological disorders such as epilepsy and sleep disorders. SVM shows good generalization performance for high dimensional data due to its convex optimization problem. The incorporation of prior knowledge about the data leads to a better optimized classifier. Different types of EEG signals provide information about the distribution of EEG data. To include prior information in the classification of EEG signals, we propose a novel machine learning approach based on universum support vector machine (USVM) for classification. In our approach, the universum data points are generated by selecting universum from the EEG dataset itself which are the interictal EEG signals. This removes the effect of outliers on the generation of universum data. Further, to reduce the computation time, we use our approach of universum selection with universum twin support vector machine (UTSVM) which has less computational cost in comparison to traditional SVM. For checking the validity of our proposed methods, we use various feature extraction techniques for different datasets consisting of healthy and seizure signals. Several numerical experiments are performed on the generated datasets and the results of our proposed approach are compared with other baseline methods. Our proposed USVM and proposed UTSVM show better generalization performance compared to SVM, USVM, Twin SVM (TWSVM) and UTSVM. The proposed UTSVM has achieved highest classification accuracy of 99% for the healthy and seizure EEG signals.
ناشر
Database: Elsevier - ScienceDirect (ساینس دایرکت)
Journal: Expert Systems with Applications - Volume 106, 15 September 2018, Pages 169-182
Journal: Expert Systems with Applications - Volume 106, 15 September 2018, Pages 169-182
نویسندگان
B. Richhariya, M. Tanveer,