کد مقاله | کد نشریه | سال انتشار | مقاله انگلیسی | نسخه تمام متن |
---|---|---|---|---|
6855232 | 1437610 | 2018 | 24 صفحه PDF | دانلود رایگان |
عنوان انگلیسی مقاله ISI
Robust EMG pattern recognition in the presence of confounding factors: features, classifiers and adaptive learning
دانلود مقاله + سفارش ترجمه
دانلود مقاله ISI انگلیسی
رایگان برای ایرانیان
کلمات کلیدی
موضوعات مرتبط
مهندسی و علوم پایه
مهندسی کامپیوتر
هوش مصنوعی
پیش نمایش صفحه اول مقاله
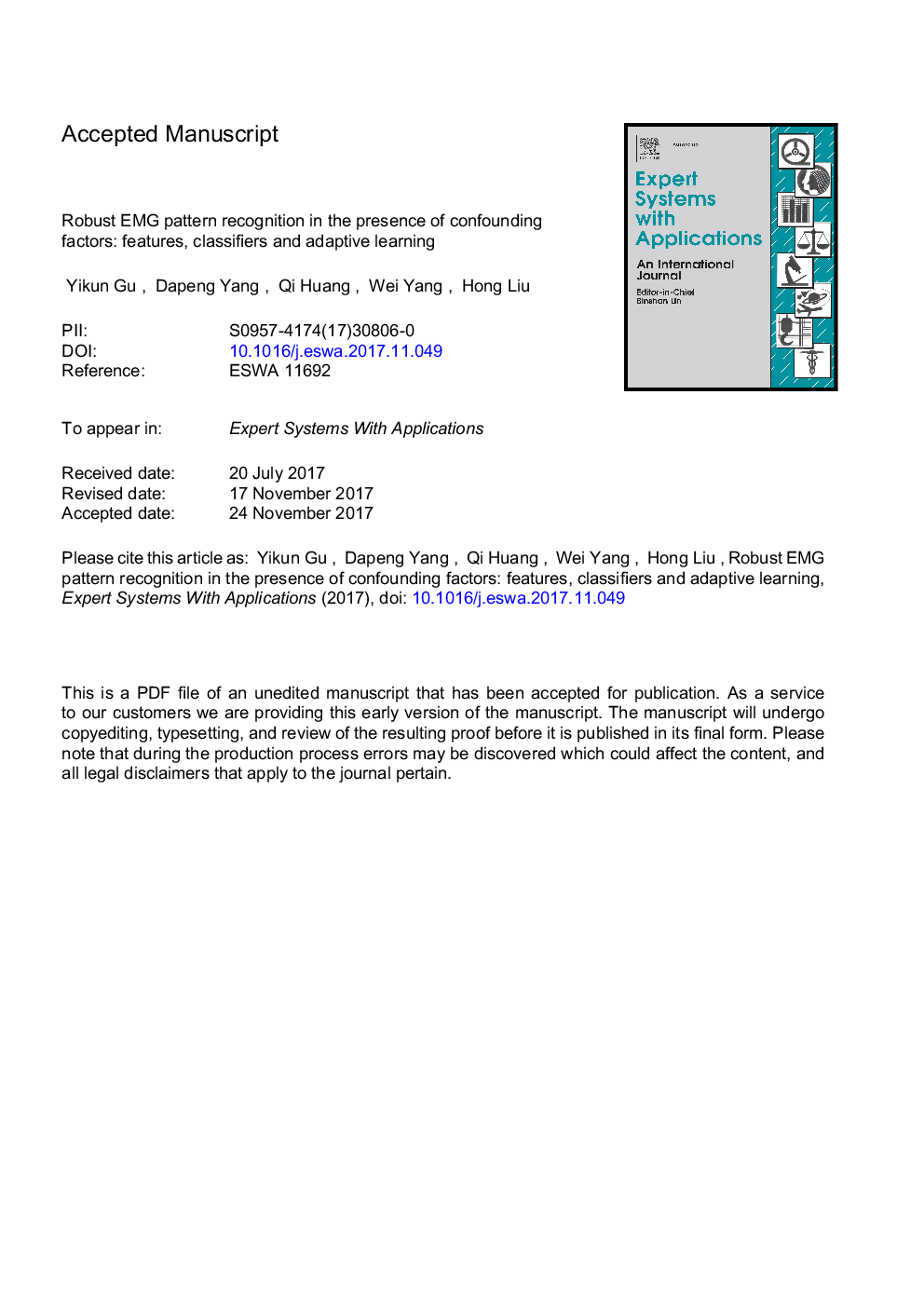
چکیده انگلیسی
Traditional electromyogram (EMG) pattern recognition does not take into account the effect of confounding factors, preventing its effective clinical application. In this paper, we investigated EMG pattern recognition of compound confounding factors including electrode shift, force variation, limb posture and temporary drift. We started with feature extraction, to identify suitable representations to resolve classification degradation caused by electrode doffing. Then, we proceeded to examine a variety of classifiers, to achieve a favorable classification accuracy (CA) when switching the control arms (dominant and nondominant) without additional calibration. Lastly, we investigated adaptive learning strategies, to mitigate the decline in CA over a relatively long duration (one day) of use. Two data-collection protocols (electrode doffing and donning, and one-day-long collection) were applied, and EMG datasets collected in these two protocols were arranged into three scenarios for progressive validation of the features, classifiers, and adaptive learning methods. Our results showed that the features extracted from the frequency domain improved the CA by 30% in comparison with their original time-domain representations (14 motions, 67.5â¯Â±â¯9.8%, pâ¯<â¯.001). When alternating the control arms, the performance achieved by the classifiers all declined and no method appeared to be more reliable than the others (14 motions, the best 45.5â¯Â±â¯8.4%, probabilistic neural network; 7 motions, the best 70.4â¯Â±â¯10.6%, linear discriminant analysis). At last, the new adaptive learning method utilizing representative samples could effectively mitigate classification degradation by updating the classifier's training samples in real-time.
ناشر
Database: Elsevier - ScienceDirect (ساینس دایرکت)
Journal: Expert Systems with Applications - Volume 96, 15 April 2018, Pages 208-217
Journal: Expert Systems with Applications - Volume 96, 15 April 2018, Pages 208-217
نویسندگان
Yikun Gu, Dapeng Yang, Qi Huang, Wei Yang, Hong Liu,