کد مقاله | کد نشریه | سال انتشار | مقاله انگلیسی | نسخه تمام متن |
---|---|---|---|---|
6866061 | 679096 | 2015 | 7 صفحه PDF | دانلود رایگان |
عنوان انگلیسی مقاله ISI
Evolving RBF neural networks for rainfall prediction using hybrid particle swarm optimization and genetic algorithm
دانلود مقاله + سفارش ترجمه
دانلود مقاله ISI انگلیسی
رایگان برای ایرانیان
کلمات کلیدی
موضوعات مرتبط
مهندسی و علوم پایه
مهندسی کامپیوتر
هوش مصنوعی
پیش نمایش صفحه اول مقاله
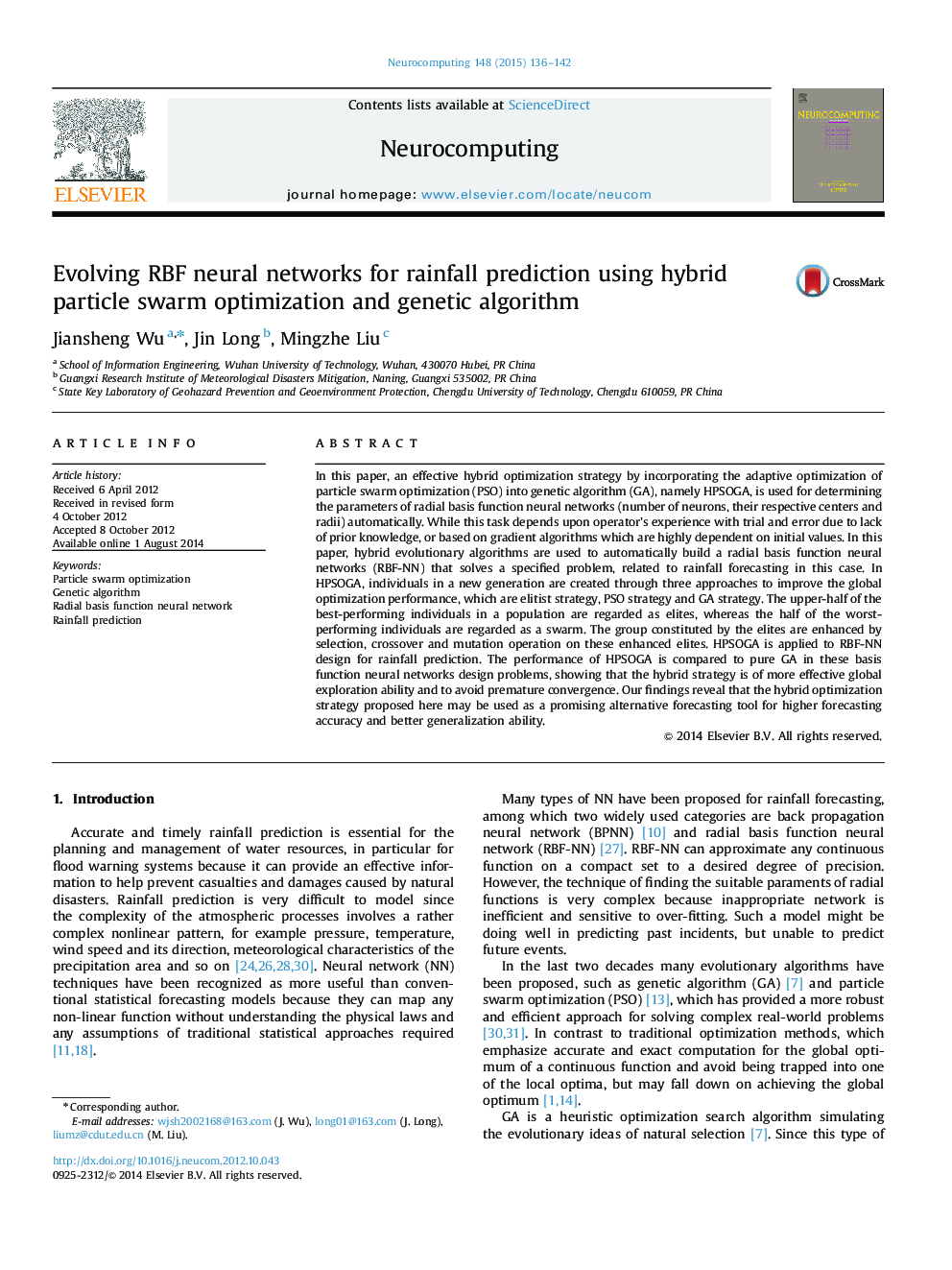
چکیده انگلیسی
In this paper, an effective hybrid optimization strategy by incorporating the adaptive optimization of particle swarm optimization (PSO) into genetic algorithm (GA), namely HPSOGA, is used for determining the parameters of radial basis function neural networks (number of neurons, their respective centers and radii) automatically. While this task depends upon operator׳s experience with trial and error due to lack of prior knowledge, or based on gradient algorithms which are highly dependent on initial values. In this paper, hybrid evolutionary algorithms are used to automatically build a radial basis function neural networks (RBF-NN) that solves a specified problem, related to rainfall forecasting in this case. In HPSOGA, individuals in a new generation are created through three approaches to improve the global optimization performance, which are elitist strategy, PSO strategy and GA strategy. The upper-half of the best-performing individuals in a population are regarded as elites, whereas the half of the worst-performing individuals are regarded as a swarm. The group constituted by the elites are enhanced by selection, crossover and mutation operation on these enhanced elites. HPSOGA is applied to RBF-NN design for rainfall prediction. The performance of HPSOGA is compared to pure GA in these basis function neural networks design problems, showing that the hybrid strategy is of more effective global exploration ability and to avoid premature convergence. Our findings reveal that the hybrid optimization strategy proposed here may be used as a promising alternative forecasting tool for higher forecasting accuracy and better generalization ability.
ناشر
Database: Elsevier - ScienceDirect (ساینس دایرکت)
Journal: Neurocomputing - Volume 148, 19 January 2015, Pages 136-142
Journal: Neurocomputing - Volume 148, 19 January 2015, Pages 136-142
نویسندگان
Jiansheng Wu, Jin Long, Mingzhe Liu,