کد مقاله | کد نشریه | سال انتشار | مقاله انگلیسی | نسخه تمام متن |
---|---|---|---|---|
6929679 | 867528 | 2016 | 23 صفحه PDF | دانلود رایگان |
عنوان انگلیسی مقاله ISI
Regularized kernel PCA for the efficient parameterization of complex geological models
دانلود مقاله + سفارش ترجمه
دانلود مقاله ISI انگلیسی
رایگان برای ایرانیان
کلمات کلیدی
موضوعات مرتبط
مهندسی و علوم پایه
مهندسی کامپیوتر
نرم افزارهای علوم کامپیوتر
پیش نمایش صفحه اول مقاله
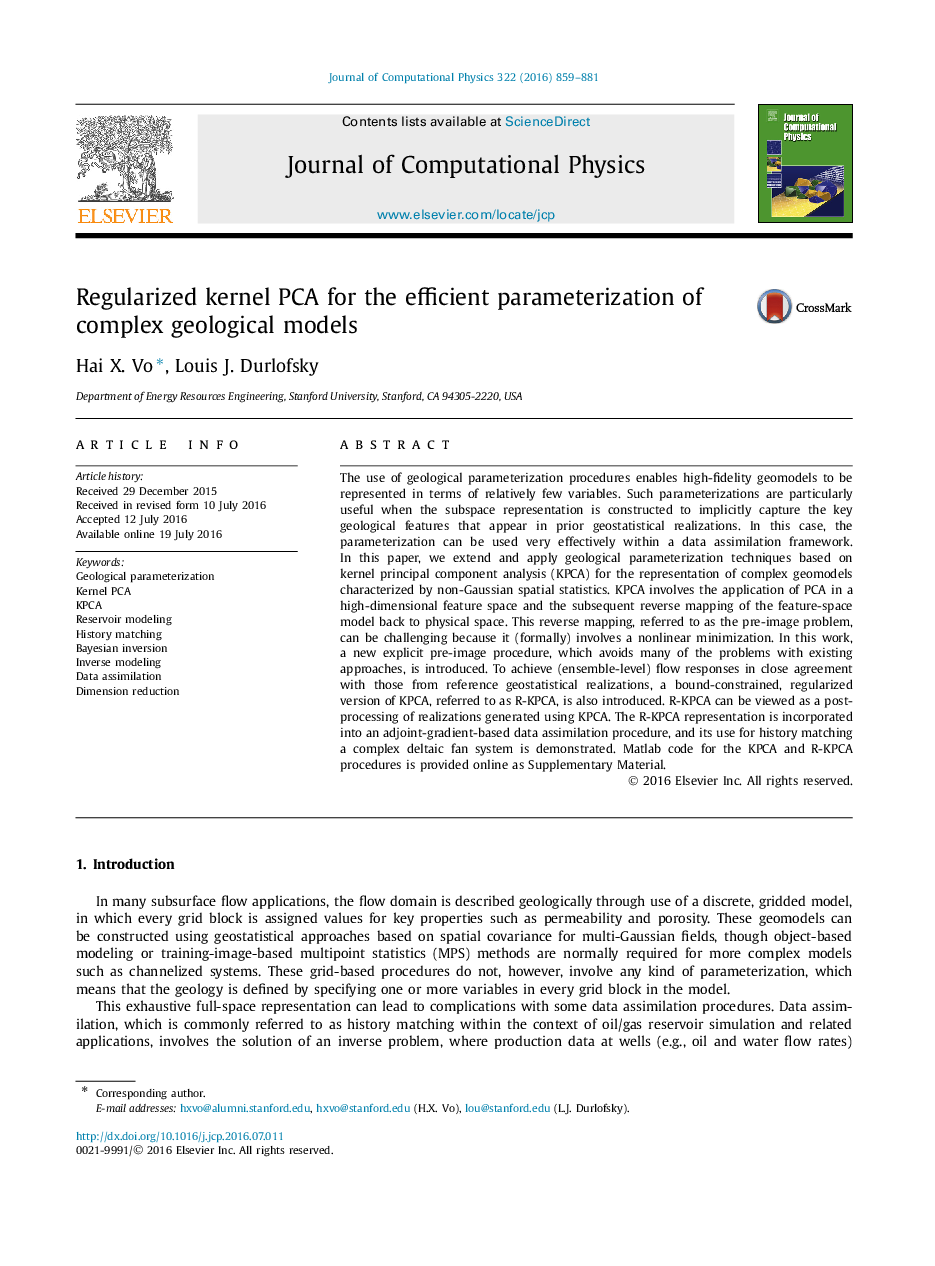
چکیده انگلیسی
The use of geological parameterization procedures enables high-fidelity geomodels to be represented in terms of relatively few variables. Such parameterizations are particularly useful when the subspace representation is constructed to implicitly capture the key geological features that appear in prior geostatistical realizations. In this case, the parameterization can be used very effectively within a data assimilation framework. In this paper, we extend and apply geological parameterization techniques based on kernel principal component analysis (KPCA) for the representation of complex geomodels characterized by non-Gaussian spatial statistics. KPCA involves the application of PCA in a high-dimensional feature space and the subsequent reverse mapping of the feature-space model back to physical space. This reverse mapping, referred to as the pre-image problem, can be challenging because it (formally) involves a nonlinear minimization. In this work, a new explicit pre-image procedure, which avoids many of the problems with existing approaches, is introduced. To achieve (ensemble-level) flow responses in close agreement with those from reference geostatistical realizations, a bound-constrained, regularized version of KPCA, referred to as R-KPCA, is also introduced. R-KPCA can be viewed as a post-processing of realizations generated using KPCA. The R-KPCA representation is incorporated into an adjoint-gradient-based data assimilation procedure, and its use for history matching a complex deltaic fan system is demonstrated. Matlab code for the KPCA and R-KPCA procedures is provided online as Supplementary Material.
ناشر
Database: Elsevier - ScienceDirect (ساینس دایرکت)
Journal: Journal of Computational Physics - Volume 322, 1 October 2016, Pages 859-881
Journal: Journal of Computational Physics - Volume 322, 1 October 2016, Pages 859-881
نویسندگان
Hai X. Vo, Louis J. Durlofsky,