کد مقاله | کد نشریه | سال انتشار | مقاله انگلیسی | نسخه تمام متن |
---|---|---|---|---|
7156531 | 1462650 | 2018 | 14 صفحه PDF | دانلود رایگان |
عنوان انگلیسی مقاله ISI
An efficient Bayesian uncertainty quantification approach with application to k-Ï-γ transition modeling
دانلود مقاله + سفارش ترجمه
دانلود مقاله ISI انگلیسی
رایگان برای ایرانیان
کلمات کلیدی
موضوعات مرتبط
مهندسی و علوم پایه
سایر رشته های مهندسی
مکانیک محاسباتی
پیش نمایش صفحه اول مقاله
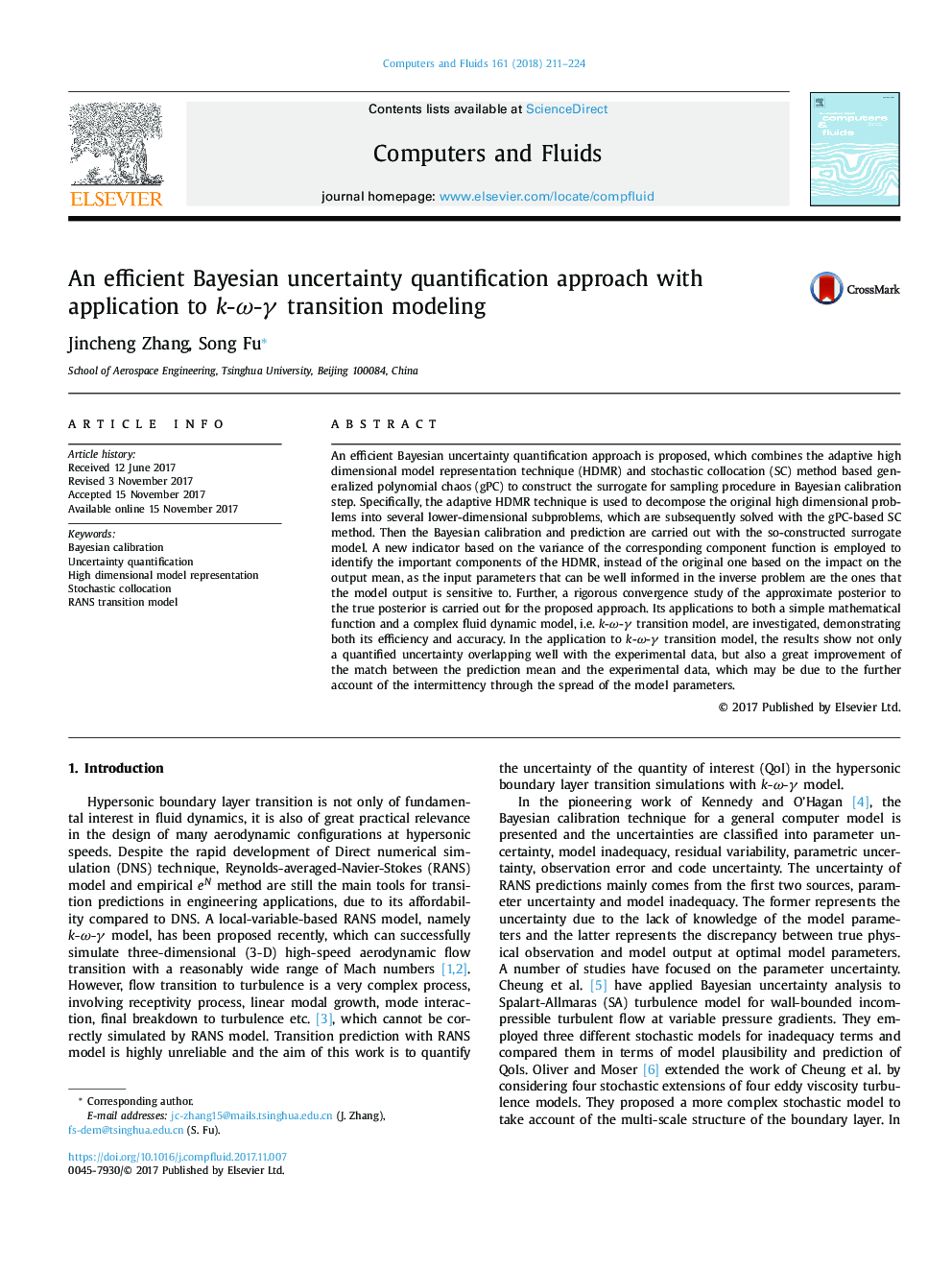
چکیده انگلیسی
An efficient Bayesian uncertainty quantification approach is proposed, which combines the adaptive high dimensional model representation technique (HDMR) and stochastic collocation (SC) method based generalized polynomial chaos (gPC) to construct the surrogate for sampling procedure in Bayesian calibration step. Specifically, the adaptive HDMR technique is used to decompose the original high dimensional problems into several lower-dimensional subproblems, which are subsequently solved with the gPC-based SC method. Then the Bayesian calibration and prediction are carried out with the so-constructed surrogate model. A new indicator based on the variance of the corresponding component function is employed to identify the important components of the HDMR, instead of the original one based on the impact on the output mean, as the input parameters that can be well informed in the inverse problem are the ones that the model output is sensitive to. Further, a rigorous convergence study of the approximate posterior to the true posterior is carried out for the proposed approach. Its applications to both a simple mathematical function and a complex fluid dynamic model, i.e. k-Ï-γ transition model, are investigated, demonstrating both its efficiency and accuracy. In the application to k-Ï-γ transition model, the results show not only a quantified uncertainty overlapping well with the experimental data, but also a great improvement of the match between the prediction mean and the experimental data, which may be due to the further account of the intermittency through the spread of the model parameters.
ناشر
Database: Elsevier - ScienceDirect (ساینس دایرکت)
Journal: Computers & Fluids - Volume 161, 15 January 2018, Pages 211-224
Journal: Computers & Fluids - Volume 161, 15 January 2018, Pages 211-224
نویسندگان
Jincheng Zhang, Song Fu,