کد مقاله | کد نشریه | سال انتشار | مقاله انگلیسی | نسخه تمام متن |
---|---|---|---|---|
737491 | 1461897 | 2013 | 11 صفحه PDF | دانلود رایگان |
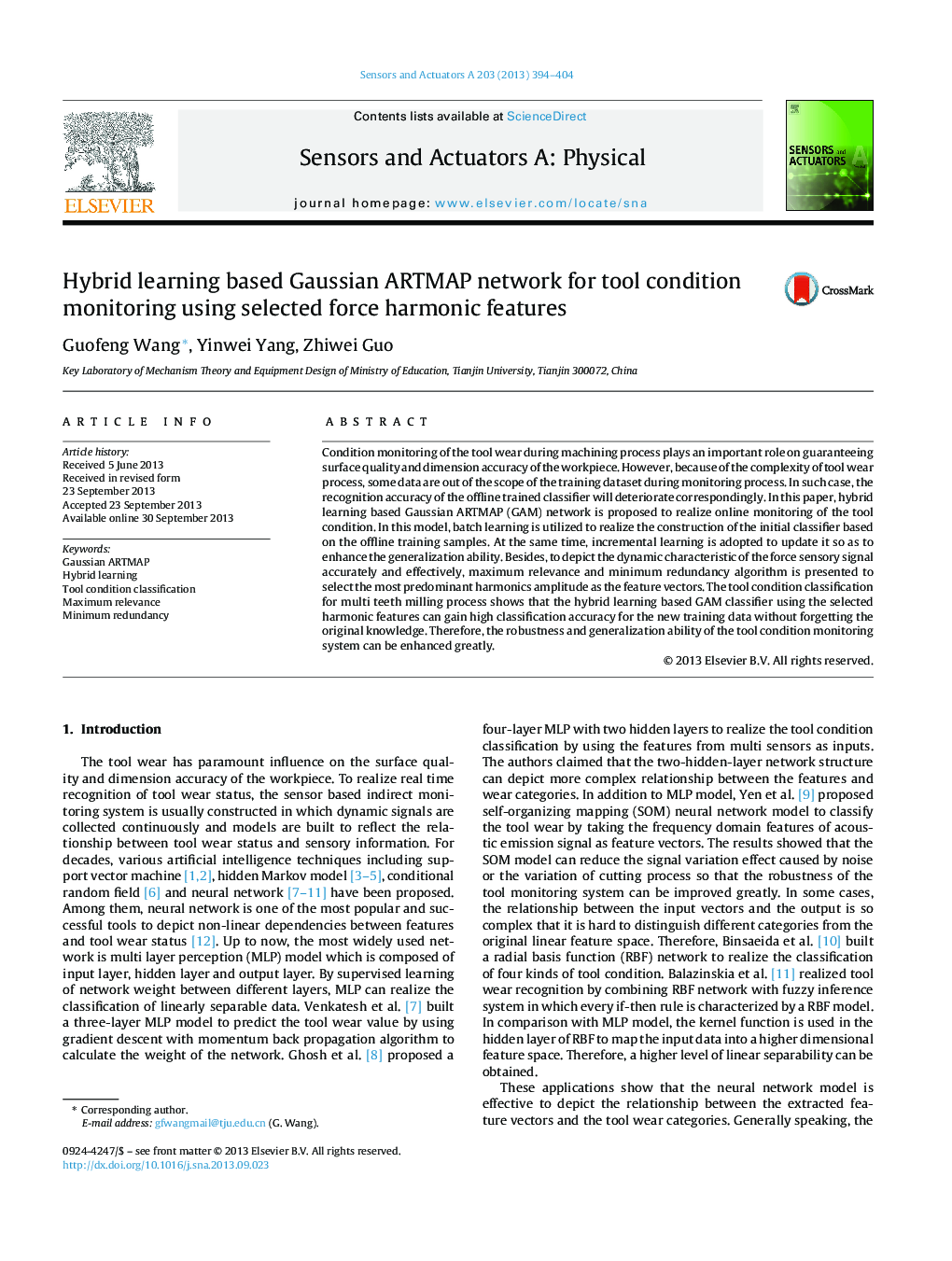
• Hybrid learning based GAM classifier is proposed to recognize tool wear states.
• mRMR algorithm is used to realize the selection of force harmonic features.
• GAM can learn new training data without forgetting the original knowledge.
• Selection ofγ is a tradeoff between training accuracy and generalization ability.
Condition monitoring of the tool wear during machining process plays an important role on guaranteeing surface quality and dimension accuracy of the workpiece. However, because of the complexity of tool wear process, some data are out of the scope of the training dataset during monitoring process. In such case, the recognition accuracy of the offline trained classifier will deteriorate correspondingly. In this paper, hybrid learning based Gaussian ARTMAP (GAM) network is proposed to realize online monitoring of the tool condition. In this model, batch learning is utilized to realize the construction of the initial classifier based on the offline training samples. At the same time, incremental learning is adopted to update it so as to enhance the generalization ability. Besides, to depict the dynamic characteristic of the force sensory signal accurately and effectively, maximum relevance and minimum redundancy algorithm is presented to select the most predominant harmonics amplitude as the feature vectors. The tool condition classification for multi teeth milling process shows that the hybrid learning based GAM classifier using the selected harmonic features can gain high classification accuracy for the new training data without forgetting the original knowledge. Therefore, the robustness and generalization ability of the tool condition monitoring system can be enhanced greatly.
Journal: Sensors and Actuators A: Physical - Volume 203, 1 December 2013, Pages 394–404