کد مقاله | کد نشریه | سال انتشار | مقاله انگلیسی | نسخه تمام متن |
---|---|---|---|---|
763488 | 1462850 | 2016 | 10 صفحه PDF | دانلود رایگان |
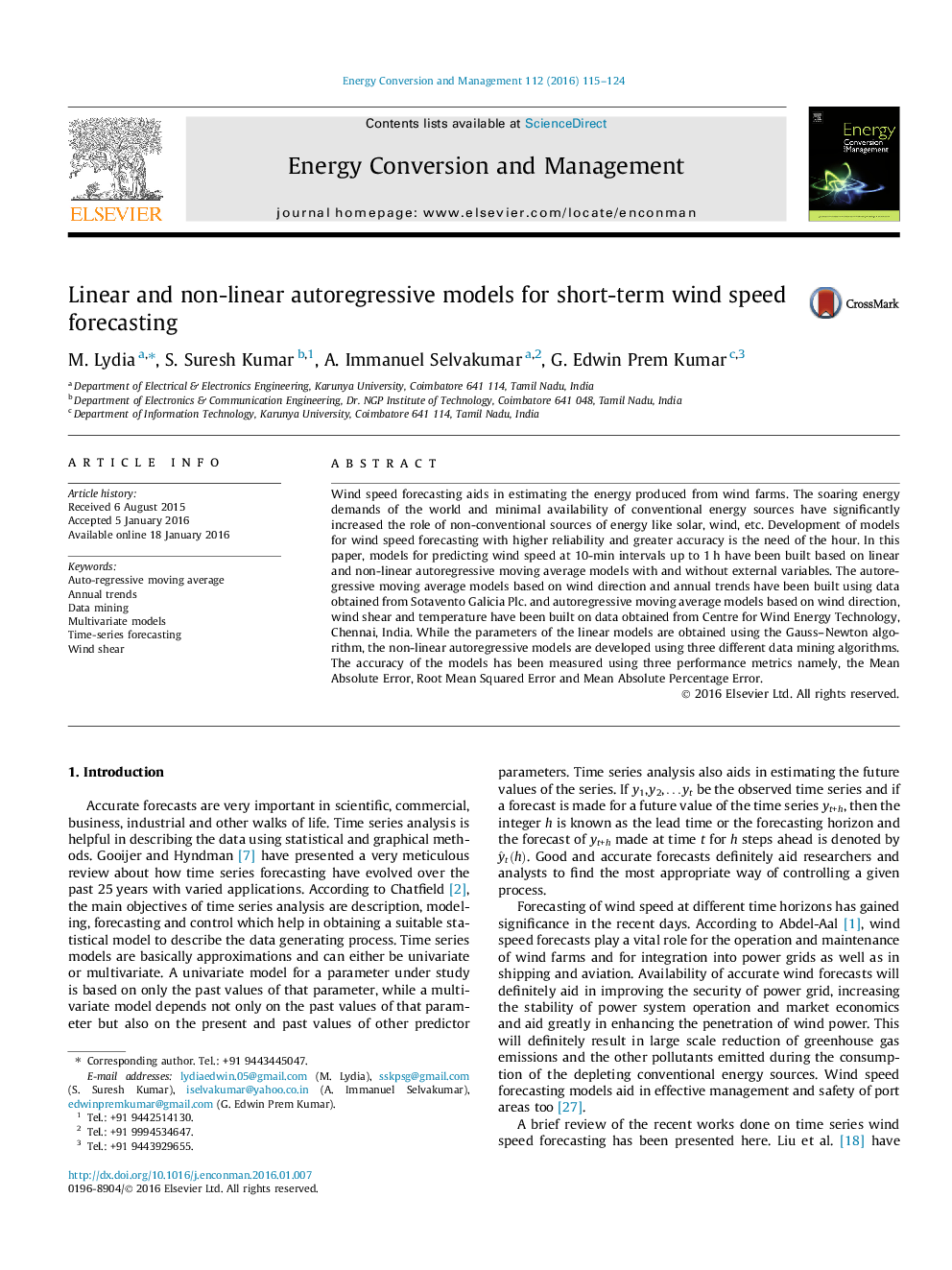
• Models for wind speed prediction at 10-min intervals up to 1 h built on time-series wind speed data.
• Four different multivariate models for wind speed built based on exogenous variables.
• Non-linear models built using three data mining algorithms outperform the linear models.
• Autoregressive models based on wind direction perform better than other models.
Wind speed forecasting aids in estimating the energy produced from wind farms. The soaring energy demands of the world and minimal availability of conventional energy sources have significantly increased the role of non-conventional sources of energy like solar, wind, etc. Development of models for wind speed forecasting with higher reliability and greater accuracy is the need of the hour. In this paper, models for predicting wind speed at 10-min intervals up to 1 h have been built based on linear and non-linear autoregressive moving average models with and without external variables. The autoregressive moving average models based on wind direction and annual trends have been built using data obtained from Sotavento Galicia Plc. and autoregressive moving average models based on wind direction, wind shear and temperature have been built on data obtained from Centre for Wind Energy Technology, Chennai, India. While the parameters of the linear models are obtained using the Gauss–Newton algorithm, the non-linear autoregressive models are developed using three different data mining algorithms. The accuracy of the models has been measured using three performance metrics namely, the Mean Absolute Error, Root Mean Squared Error and Mean Absolute Percentage Error.
Journal: Energy Conversion and Management - Volume 112, 15 March 2016, Pages 115–124