کد مقاله | کد نشریه | سال انتشار | مقاله انگلیسی | نسخه تمام متن |
---|---|---|---|---|
792683 | 1466402 | 2016 | 12 صفحه PDF | دانلود رایگان |
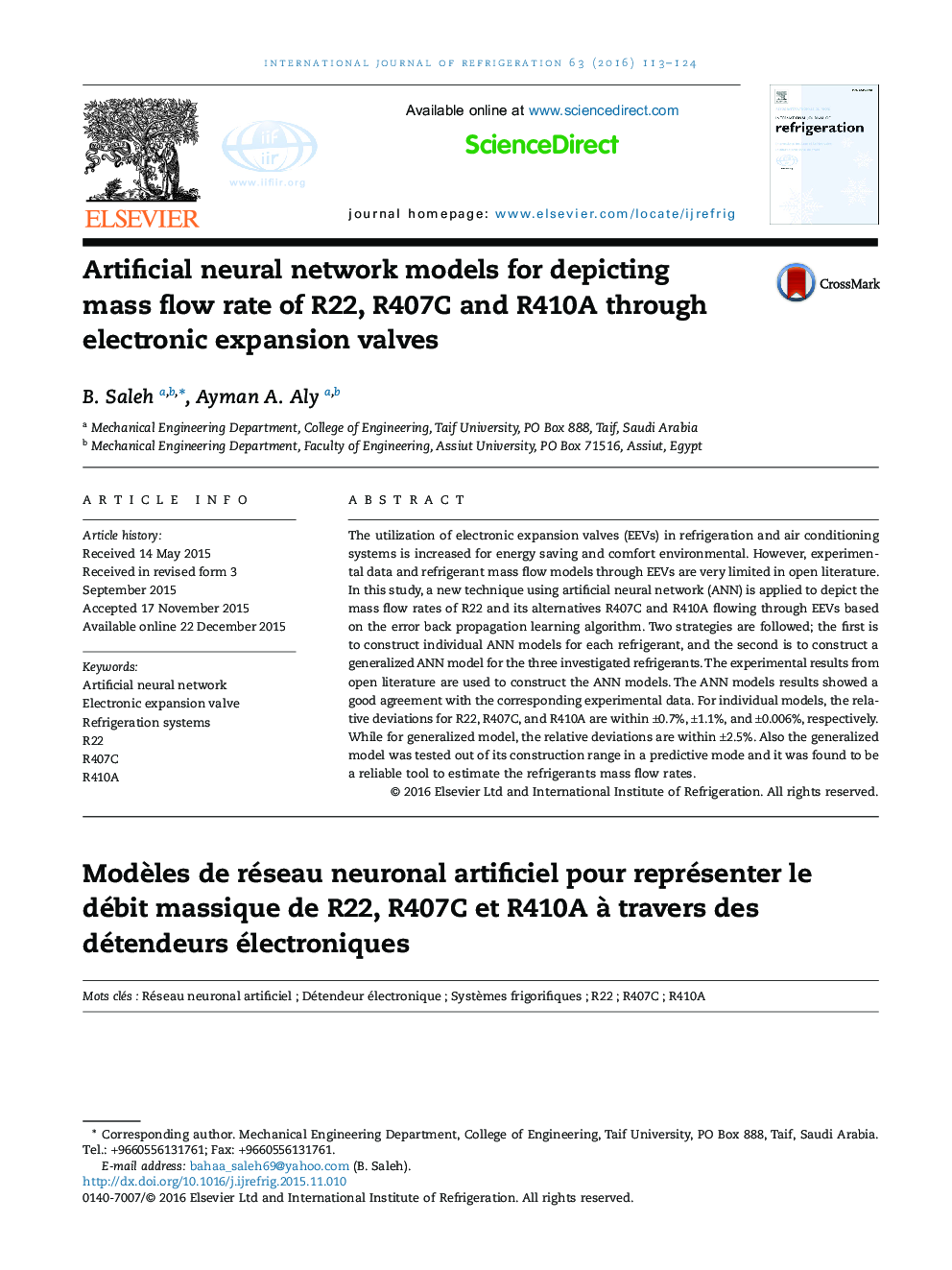
• We developed ANN models to depict refrigerant mass flow through expansion valve.
• The studied refrigerants are R22, R407C and R410A.
• Experimental results from open literature are used to construct the ANN models.
• Nine major parameters are selected as input of the models to deduce the flow rate.
• The models results showed good agreement with the corresponding experimental data.
The utilization of electronic expansion valves (EEVs) in refrigeration and air conditioning systems is increased for energy saving and comfort environmental. However, experimental data and refrigerant mass flow models through EEVs are very limited in open literature. In this study, a new technique using artificial neural network (ANN) is applied to depict the mass flow rates of R22 and its alternatives R407C and R410A flowing through EEVs based on the error back propagation learning algorithm. Two strategies are followed; the first is to construct individual ANN models for each refrigerant, and the second is to construct a generalized ANN model for the three investigated refrigerants. The experimental results from open literature are used to construct the ANN models. The ANN models results showed a good agreement with the corresponding experimental data. For individual models, the relative deviations for R22, R407C, and R410A are within ±0.7%, ±1.1%, and ±0.006%, respectively. While for generalized model, the relative deviations are within ±2.5%. Also the generalized model was tested out of its construction range in a predictive mode and it was found to be a reliable tool to estimate the refrigerants mass flow rates.
Journal: International Journal of Refrigeration - Volume 63, March 2016, Pages 113–124