کد مقاله | کد نشریه | سال انتشار | مقاله انگلیسی | نسخه تمام متن |
---|---|---|---|---|
377980 | 658859 | 2008 | 19 صفحه PDF | دانلود رایگان |
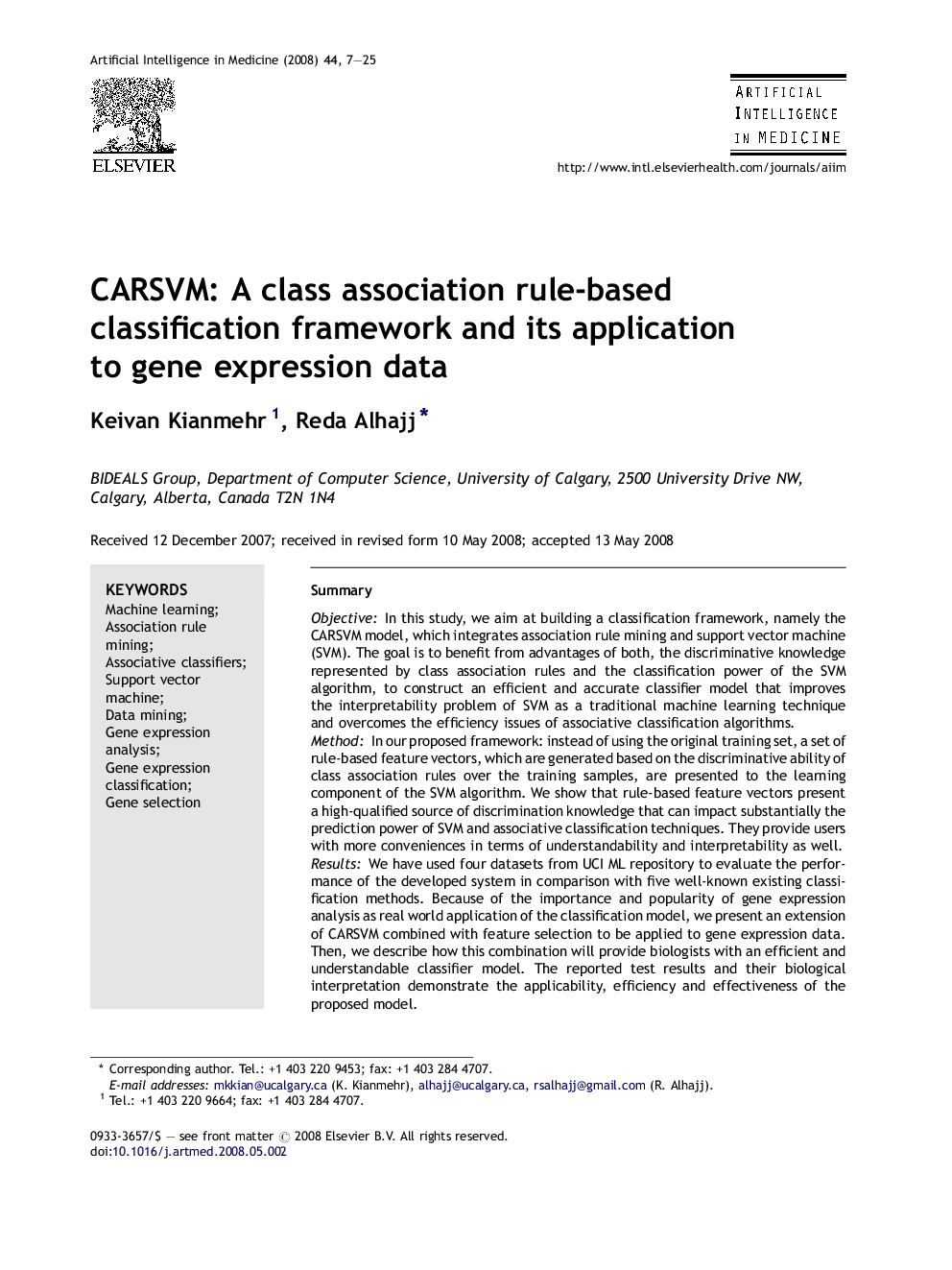
SummaryObjectiveIn this study, we aim at building a classification framework, namely the CARSVM model, which integrates association rule mining and support vector machine (SVM). The goal is to benefit from advantages of both, the discriminative knowledge represented by class association rules and the classification power of the SVM algorithm, to construct an efficient and accurate classifier model that improves the interpretability problem of SVM as a traditional machine learning technique and overcomes the efficiency issues of associative classification algorithms.MethodIn our proposed framework: instead of using the original training set, a set of rule-based feature vectors, which are generated based on the discriminative ability of class association rules over the training samples, are presented to the learning component of the SVM algorithm. We show that rule-based feature vectors present a high-qualified source of discrimination knowledge that can impact substantially the prediction power of SVM and associative classification techniques. They provide users with more conveniences in terms of understandability and interpretability as well.ResultsWe have used four datasets from UCI ML repository to evaluate the performance of the developed system in comparison with five well-known existing classification methods. Because of the importance and popularity of gene expression analysis as real world application of the classification model, we present an extension of CARSVM combined with feature selection to be applied to gene expression data. Then, we describe how this combination will provide biologists with an efficient and understandable classifier model. The reported test results and their biological interpretation demonstrate the applicability, efficiency and effectiveness of the proposed model.ConclusionFrom the results, it can be concluded that a considerable increase in classification accuracy can be obtained when the rule-based feature vectors are integrated in the learning process of the SVM algorithm. In the context of applicability, according to the results obtained from gene expression analysis, we can conclude that the CARSVM system can be utilized in a variety of real world applications with some adjustments.
Journal: Artificial Intelligence in Medicine - Volume 44, Issue 1, September 2008, Pages 7–25