کد مقاله | کد نشریه | سال انتشار | مقاله انگلیسی | نسخه تمام متن |
---|---|---|---|---|
381009 | 1437457 | 2013 | 12 صفحه PDF | دانلود رایگان |
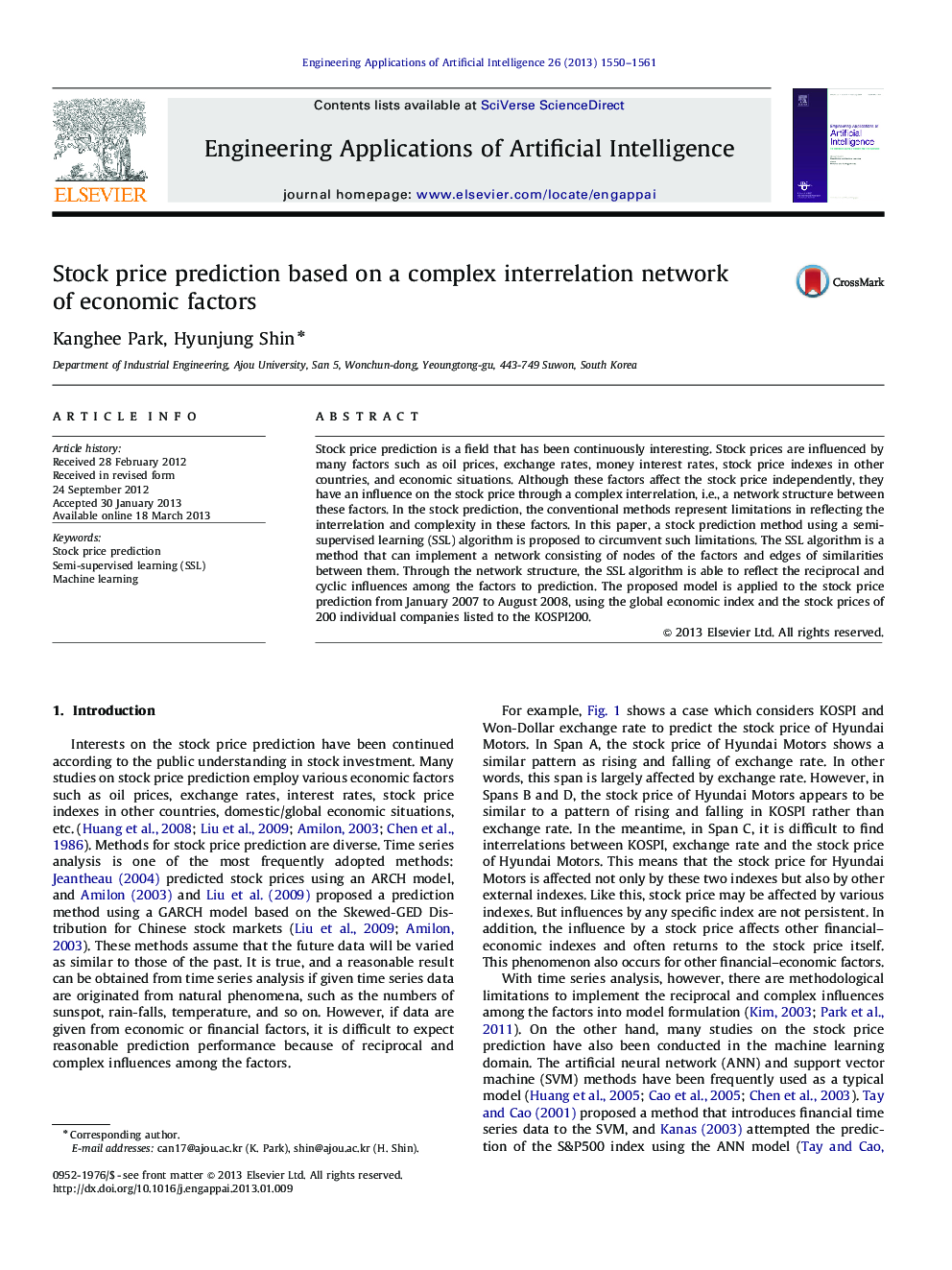
Stock price prediction is a field that has been continuously interesting. Stock prices are influenced by many factors such as oil prices, exchange rates, money interest rates, stock price indexes in other countries, and economic situations. Although these factors affect the stock price independently, they have an influence on the stock price through a complex interrelation, i.e., a network structure between these factors. In the stock prediction, the conventional methods represent limitations in reflecting the interrelation and complexity in these factors. In this paper, a stock prediction method using a semi-supervised learning (SSL) algorithm is proposed to circumvent such limitations. The SSL algorithm is a method that can implement a network consisting of nodes of the factors and edges of similarities between them. Through the network structure, the SSL algorithm is able to reflect the reciprocal and cyclic influences among the factors to prediction. The proposed model is applied to the stock price prediction from January 2007 to August 2008, using the global economic index and the stock prices of 200 individual companies listed to the KOSPI200.
► The movement of stock prices is predicted based on the network of diverse financial and economic factors.
► The semi-supervised learning (SSL) is employed to represent the complex interrelation of the network.
► SSL outperformed other competing models in terms of accuracy and earning rate as well.
Journal: Engineering Applications of Artificial Intelligence - Volume 26, Issues 5–6, May–June 2013, Pages 1550–1561