کد مقاله | کد نشریه | سال انتشار | مقاله انگلیسی | نسخه تمام متن |
---|---|---|---|---|
383961 | 660837 | 2013 | 10 صفحه PDF | دانلود رایگان |
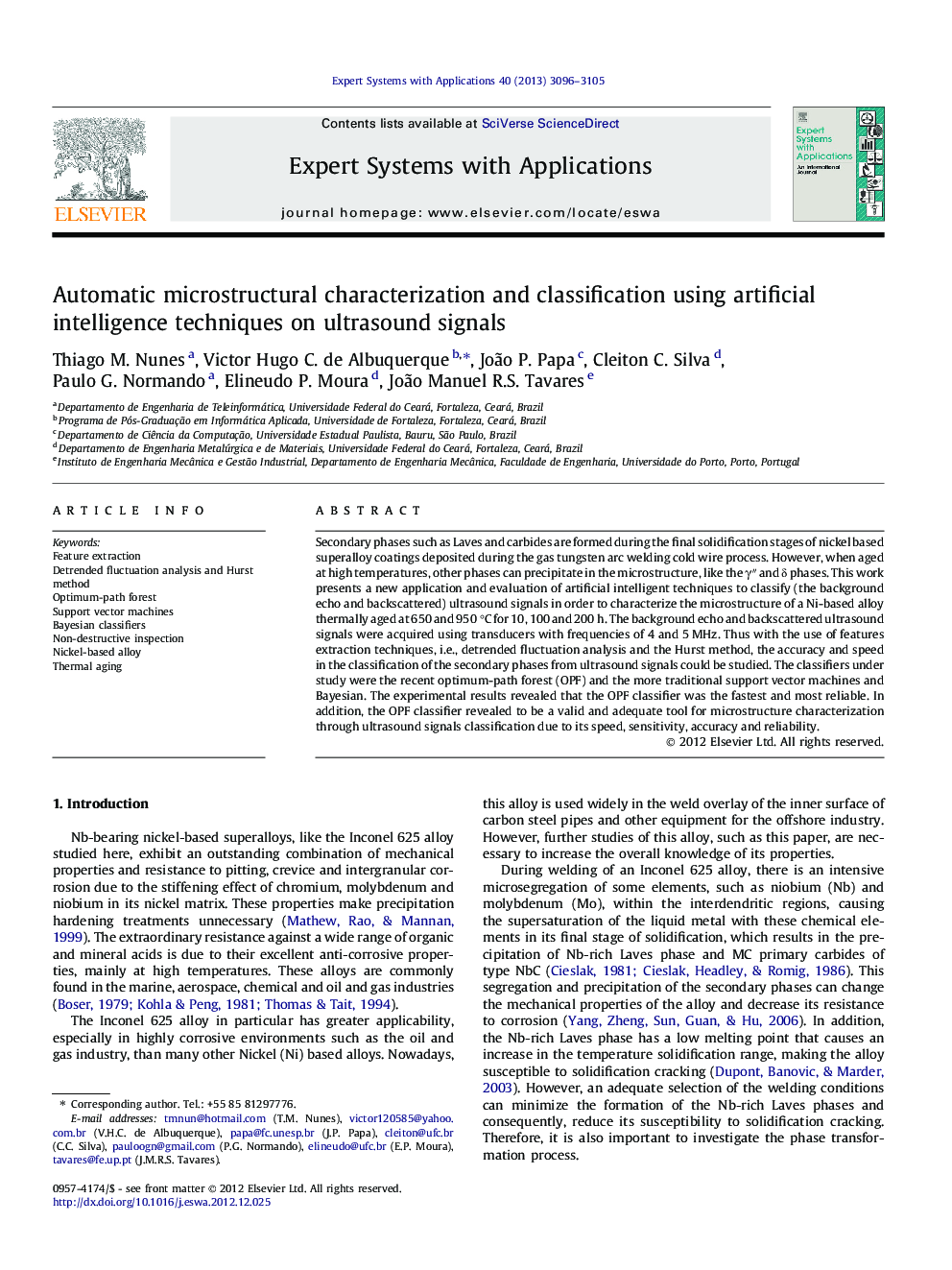
Secondary phases such as Laves and carbides are formed during the final solidification stages of nickel based superalloy coatings deposited during the gas tungsten arc welding cold wire process. However, when aged at high temperatures, other phases can precipitate in the microstructure, like the γ″ and δ phases. This work presents a new application and evaluation of artificial intelligent techniques to classify (the background echo and backscattered) ultrasound signals in order to characterize the microstructure of a Ni-based alloy thermally aged at 650 and 950 °C for 10, 100 and 200 h. The background echo and backscattered ultrasound signals were acquired using transducers with frequencies of 4 and 5 MHz. Thus with the use of features extraction techniques, i.e., detrended fluctuation analysis and the Hurst method, the accuracy and speed in the classification of the secondary phases from ultrasound signals could be studied. The classifiers under study were the recent optimum-path forest (OPF) and the more traditional support vector machines and Bayesian. The experimental results revealed that the OPF classifier was the fastest and most reliable. In addition, the OPF classifier revealed to be a valid and adequate tool for microstructure characterization through ultrasound signals classification due to its speed, sensitivity, accuracy and reliability.
► To monitor the kinetics of phase transformation from Ni-based alloy thermally aged and in the as-weld state.
► The ultrasound signals are preprocessed by detrended fluctuation analysis and the Hurst method.
► The speed and accuracy of the OPF (accuracy rate superior to 90%) used for first time in this domain.
► The OPF, SVMs and Bayesian artificial intelligence methods are analyzed to verify time and accuracy.
► To predict the right moment to carry out maintenance services, reducing costs and maintenance time.
Journal: Expert Systems with Applications - Volume 40, Issue 8, 15 June 2013, Pages 3096–3105