کد مقاله | کد نشریه | سال انتشار | مقاله انگلیسی | نسخه تمام متن |
---|---|---|---|---|
410353 | 679137 | 2013 | 9 صفحه PDF | دانلود رایگان |
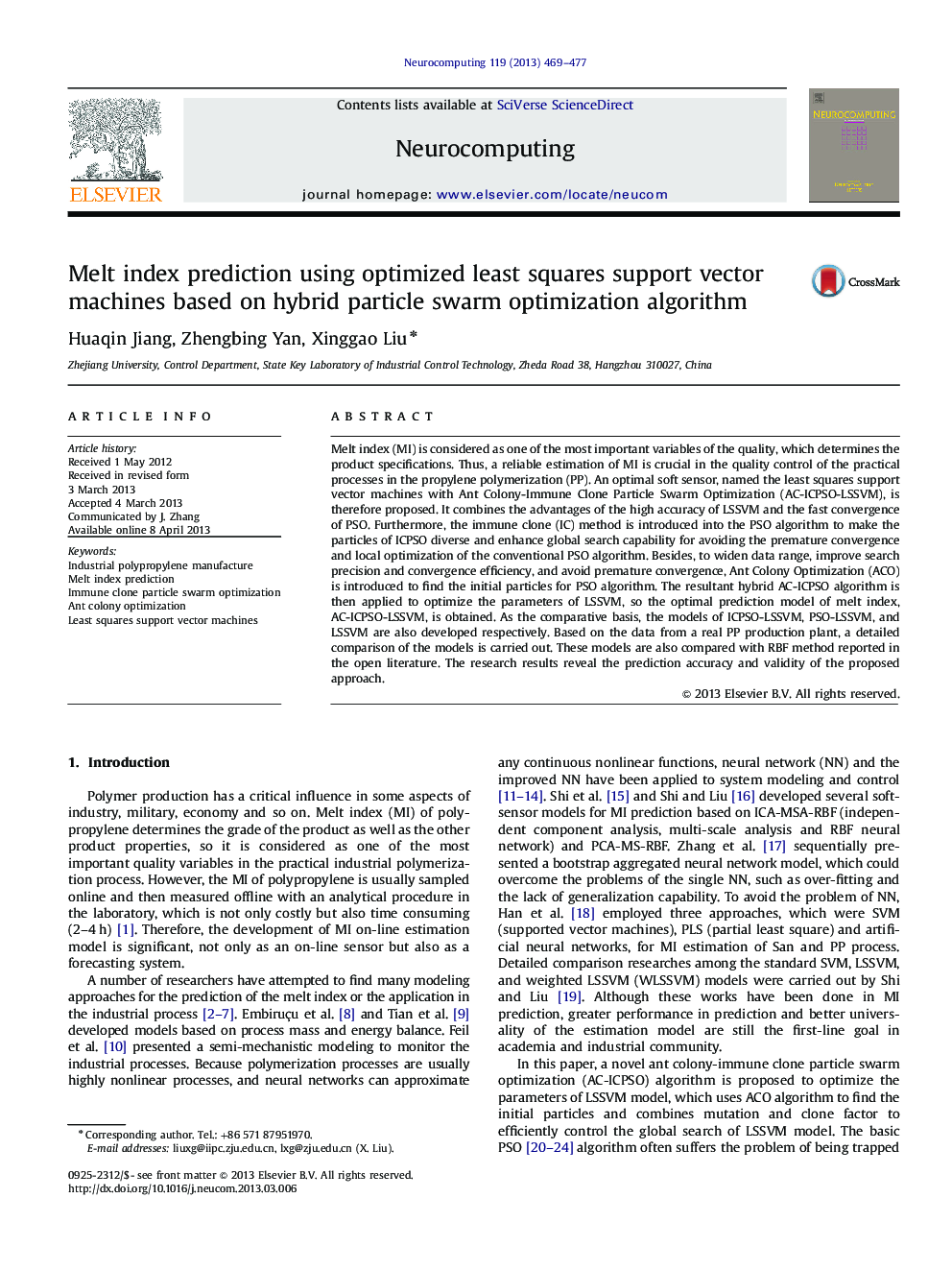
Melt index (MI) is considered as one of the most important variables of the quality, which determines the product specifications. Thus, a reliable estimation of MI is crucial in the quality control of the practical processes in the propylene polymerization (PP). An optimal soft sensor, named the least squares support vector machines with Ant Colony-Immune Clone Particle Swarm Optimization (AC-ICPSO-LSSVM), is therefore proposed. It combines the advantages of the high accuracy of LSSVM and the fast convergence of PSO. Furthermore, the immune clone (IC) method is introduced into the PSO algorithm to make the particles of ICPSO diverse and enhance global search capability for avoiding the premature convergence and local optimization of the conventional PSO algorithm. Besides, to widen data range, improve search precision and convergence efficiency, and avoid premature convergence, Ant Colony Optimization (ACO) is introduced to find the initial particles for PSO algorithm. The resultant hybrid AC-ICPSO algorithm is then applied to optimize the parameters of LSSVM, so the optimal prediction model of melt index, AC-ICPSO-LSSVM, is obtained. As the comparative basis, the models of ICPSO-LSSVM, PSO-LSSVM, and LSSVM are also developed respectively. Based on the data from a real PP production plant, a detailed comparison of the models is carried out. These models are also compared with RBF method reported in the open literature. The research results reveal the prediction accuracy and validity of the proposed approach.
Journal: Neurocomputing - Volume 119, 7 November 2013, Pages 469–477