کد مقاله | کد نشریه | سال انتشار | مقاله انگلیسی | نسخه تمام متن |
---|---|---|---|---|
415867 | 681247 | 2012 | 20 صفحه PDF | دانلود رایگان |
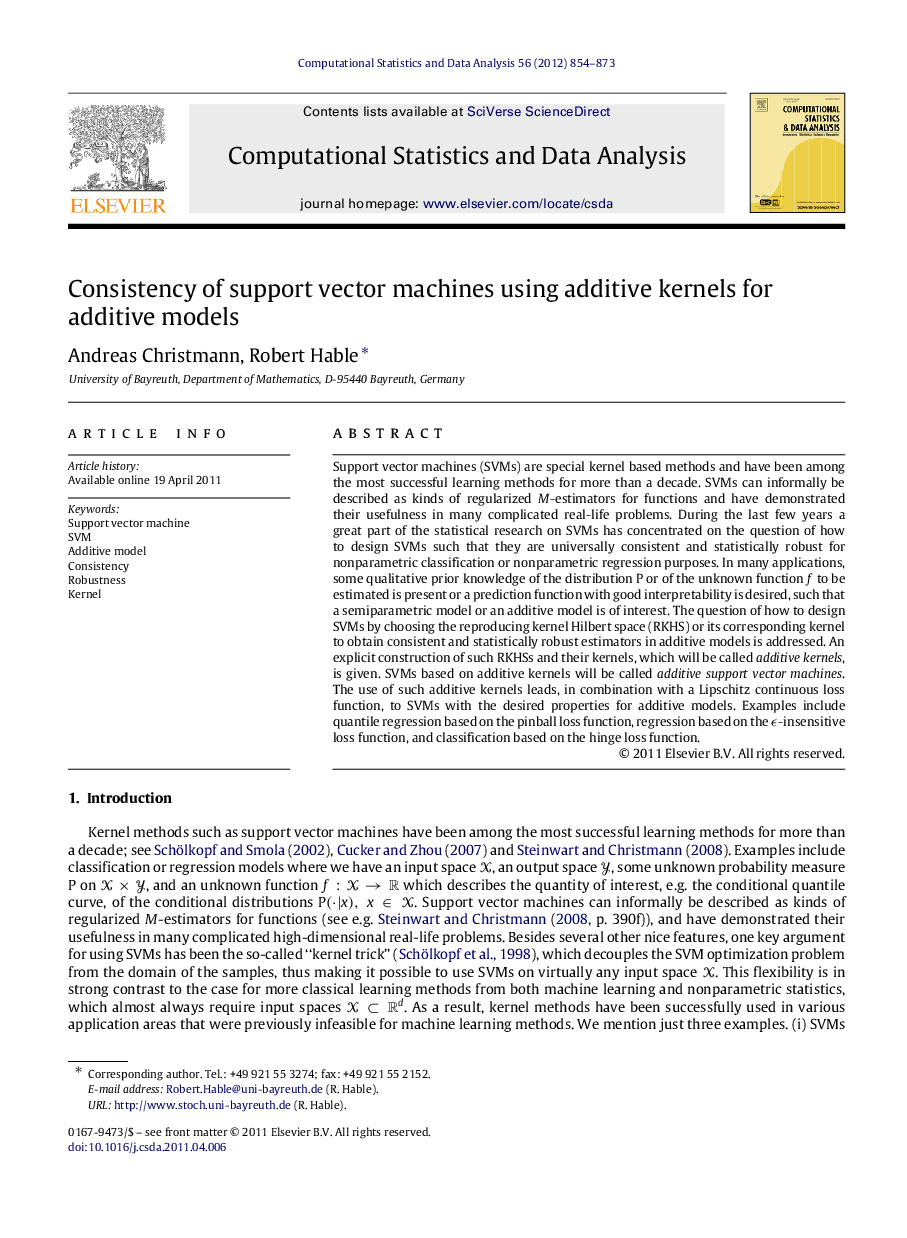
Support vector machines (SVMs) are special kernel based methods and have been among the most successful learning methods for more than a decade. SVMs can informally be described as kinds of regularized MM-estimators for functions and have demonstrated their usefulness in many complicated real-life problems. During the last few years a great part of the statistical research on SVMs has concentrated on the question of how to design SVMs such that they are universally consistent and statistically robust for nonparametric classification or nonparametric regression purposes. In many applications, some qualitative prior knowledge of the distribution P or of the unknown function ff to be estimated is present or a prediction function with good interpretability is desired, such that a semiparametric model or an additive model is of interest. The question of how to design SVMs by choosing the reproducing kernel Hilbert space (RKHS) or its corresponding kernel to obtain consistent and statistically robust estimators in additive models is addressed. An explicit construction of such RKHSs and their kernels, which will be called additive kernels, is given. SVMs based on additive kernels will be called additive support vector machines . The use of such additive kernels leads, in combination with a Lipschitz continuous loss function, to SVMs with the desired properties for additive models. Examples include quantile regression based on the pinball loss function, regression based on the ϵϵ-insensitive loss function, and classification based on the hinge loss function.
Journal: Computational Statistics & Data Analysis - Volume 56, Issue 4, 1 April 2012, Pages 854–873