کد مقاله | کد نشریه | سال انتشار | مقاله انگلیسی | نسخه تمام متن |
---|---|---|---|---|
4576048 | 1629940 | 2014 | 17 صفحه PDF | دانلود رایگان |
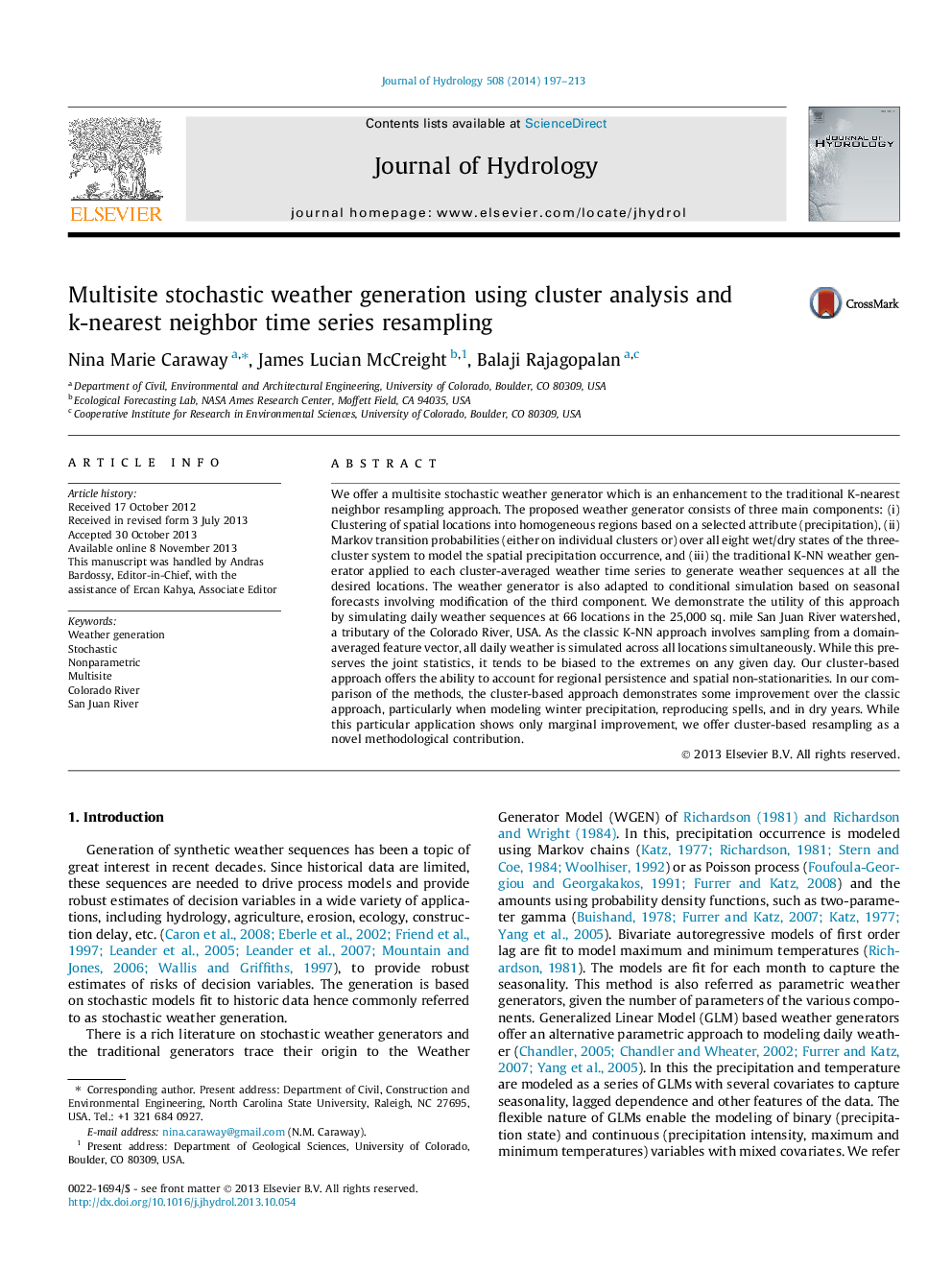
• We develop a multisite, stochastic weather generator for hydrologic simulation.
• Our approach combines precipitation-based clustering and Markov Chain states.
• Simulation achieved through K-NN resampling based on Markov states.
• Has option of simulation conditioned on seasonal probabilistic climate forecasts.
• We simulate 66 locations in the 25,000 sq. mile San Juan River watershed of Colorado River Basin, USA.
We offer a multisite stochastic weather generator which is an enhancement to the traditional K-nearest neighbor resampling approach. The proposed weather generator consists of three main components: (i) Clustering of spatial locations into homogeneous regions based on a selected attribute (precipitation), (ii) Markov transition probabilities (either on individual clusters or) over all eight wet/dry states of the three-cluster system to model the spatial precipitation occurrence, and (iii) the traditional K-NN weather generator applied to each cluster-averaged weather time series to generate weather sequences at all the desired locations. The weather generator is also adapted to conditional simulation based on seasonal forecasts involving modification of the third component. We demonstrate the utility of this approach by simulating daily weather sequences at 66 locations in the 25,000 sq. mile San Juan River watershed, a tributary of the Colorado River, USA. As the classic K-NN approach involves sampling from a domain-averaged feature vector, all daily weather is simulated across all locations simultaneously. While this preserves the joint statistics, it tends to be biased to the extremes on any given day. Our cluster-based approach offers the ability to account for regional persistence and spatial non-stationarities. In our comparison of the methods, the cluster-based approach demonstrates some improvement over the classic approach, particularly when modeling winter precipitation, reproducing spells, and in dry years. While this particular application shows only marginal improvement, we offer cluster-based resampling as a novel methodological contribution.
Journal: Journal of Hydrology - Volume 508, 16 January 2014, Pages 197–213