کد مقاله | کد نشریه | سال انتشار | مقاله انگلیسی | نسخه تمام متن |
---|---|---|---|---|
4580045 | 1630149 | 2007 | 13 صفحه PDF | دانلود رایگان |
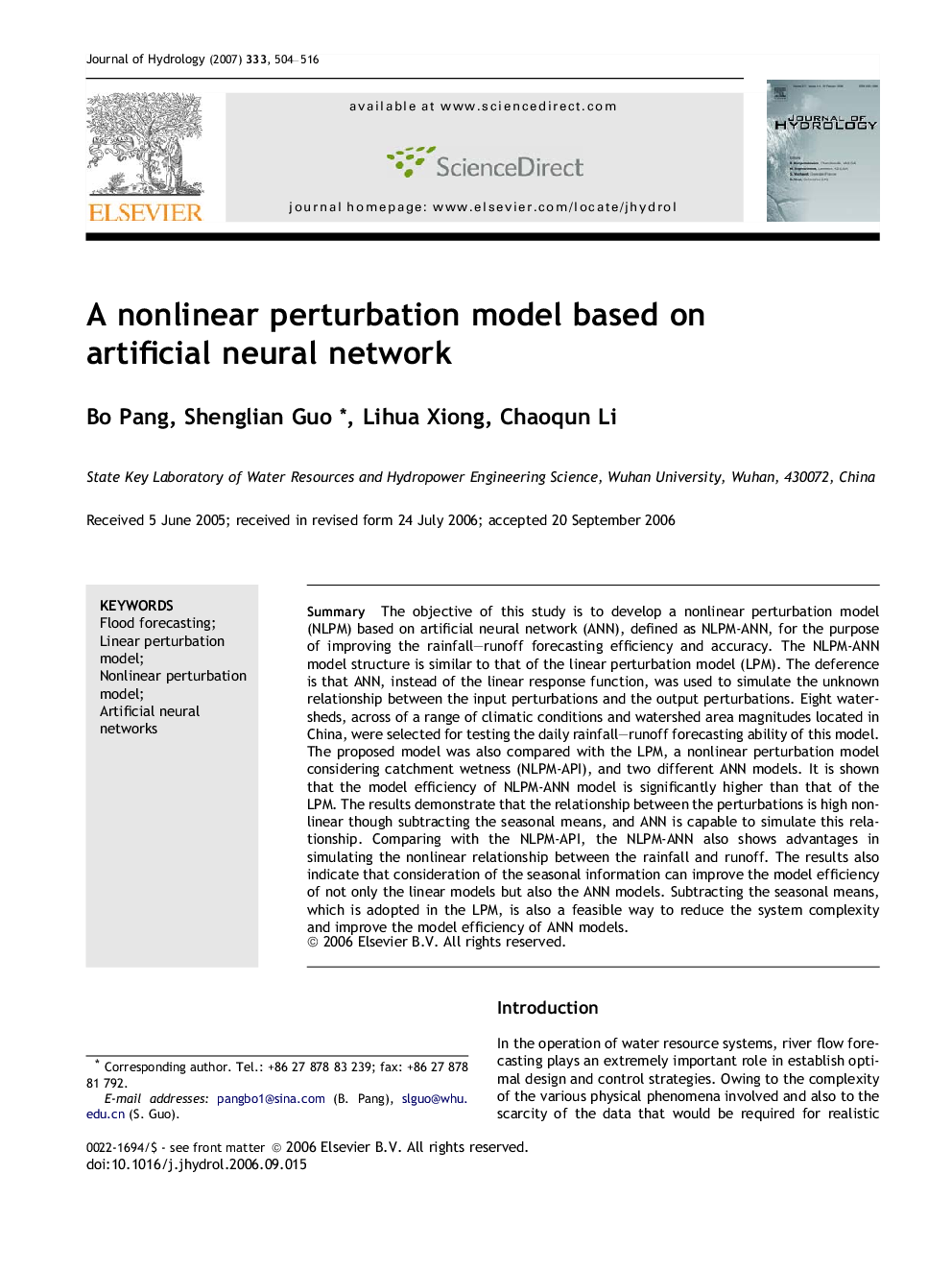
SummaryThe objective of this study is to develop a nonlinear perturbation model (NLPM) based on artificial neural network (ANN), defined as NLPM-ANN, for the purpose of improving the rainfall–runoff forecasting efficiency and accuracy. The NLPM-ANN model structure is similar to that of the linear perturbation model (LPM). The deference is that ANN, instead of the linear response function, was used to simulate the unknown relationship between the input perturbations and the output perturbations. Eight watersheds, across of a range of climatic conditions and watershed area magnitudes located in China, were selected for testing the daily rainfall–runoff forecasting ability of this model. The proposed model was also compared with the LPM, a nonlinear perturbation model considering catchment wetness (NLPM-API), and two different ANN models. It is shown that the model efficiency of NLPM-ANN model is significantly higher than that of the LPM. The results demonstrate that the relationship between the perturbations is high nonlinear though subtracting the seasonal means, and ANN is capable to simulate this relationship. Comparing with the NLPM-API, the NLPM-ANN also shows advantages in simulating the nonlinear relationship between the rainfall and runoff. The results also indicate that consideration of the seasonal information can improve the model efficiency of not only the linear models but also the ANN models. Subtracting the seasonal means, which is adopted in the LPM, is also a feasible way to reduce the system complexity and improve the model efficiency of ANN models.
Journal: Journal of Hydrology - Volume 333, Issues 2–4, 15 February 2007, Pages 504–516