کد مقاله | کد نشریه | سال انتشار | مقاله انگلیسی | نسخه تمام متن |
---|---|---|---|---|
494890 | 862809 | 2016 | 14 صفحه PDF | دانلود رایگان |
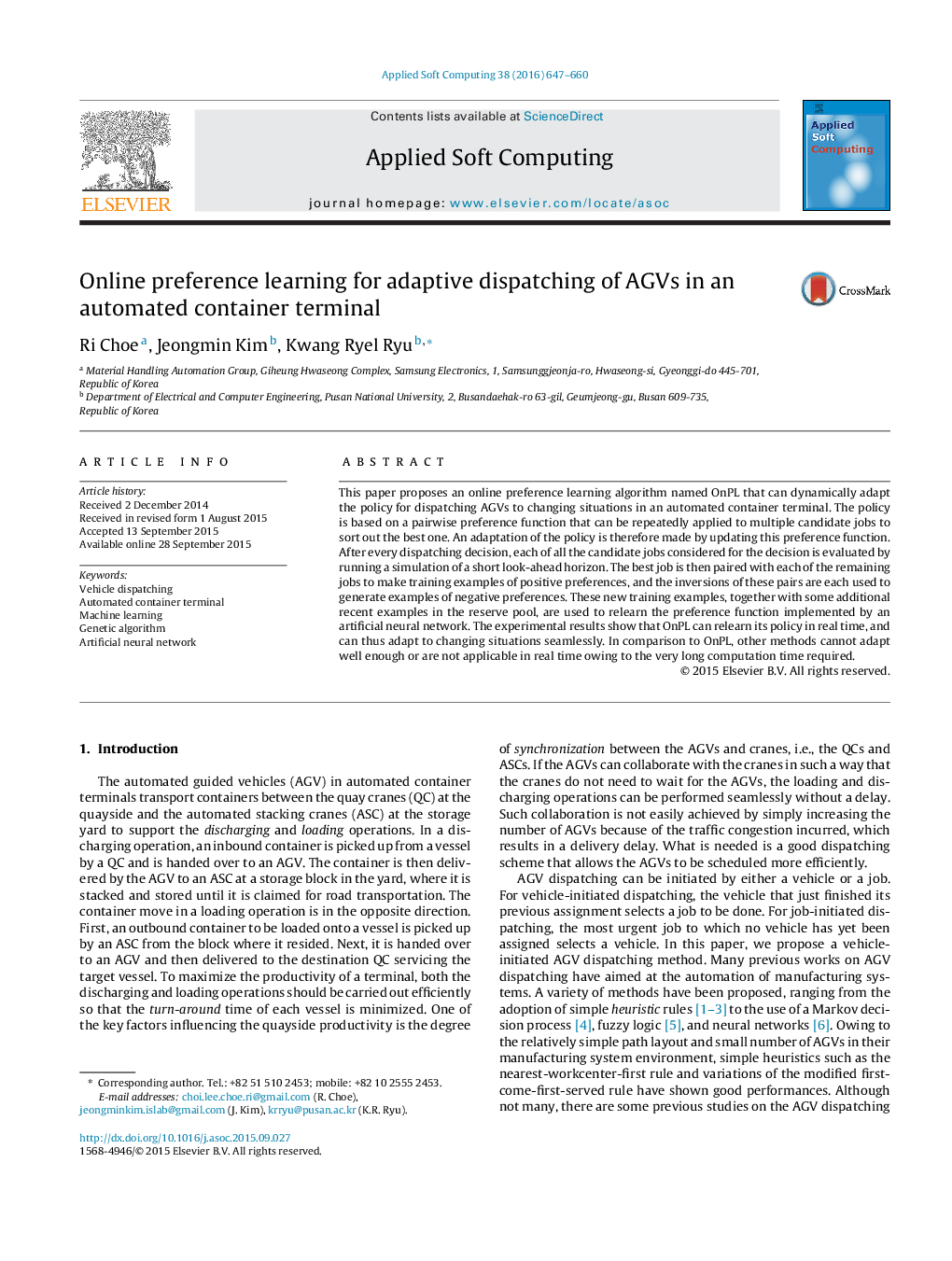
• AGV dispatching can be done by using a policy recommending the best job assignment.
• The policy proposed in this paper is based on a pairwise preference function.
• The policy can be adapted by having the preference function updated through learning.
• The pairwise preference function is implemented as a neural network.
This paper proposes an online preference learning algorithm named OnPL that can dynamically adapt the policy for dispatching AGVs to changing situations in an automated container terminal. The policy is based on a pairwise preference function that can be repeatedly applied to multiple candidate jobs to sort out the best one. An adaptation of the policy is therefore made by updating this preference function. After every dispatching decision, each of all the candidate jobs considered for the decision is evaluated by running a simulation of a short look-ahead horizon. The best job is then paired with each of the remaining jobs to make training examples of positive preferences, and the inversions of these pairs are each used to generate examples of negative preferences. These new training examples, together with some additional recent examples in the reserve pool, are used to relearn the preference function implemented by an artificial neural network. The experimental results show that OnPL can relearn its policy in real time, and can thus adapt to changing situations seamlessly. In comparison to OnPL, other methods cannot adapt well enough or are not applicable in real time owing to the very long computation time required.
Figure optionsDownload as PowerPoint slide
Journal: Applied Soft Computing - Volume 38, January 2016, Pages 647–660