کد مقاله | کد نشریه | سال انتشار | مقاله انگلیسی | نسخه تمام متن |
---|---|---|---|---|
4949167 | 1440038 | 2018 | 17 صفحه PDF | دانلود رایگان |
عنوان انگلیسی مقاله ISI
Accelerating pseudo-marginal MCMC using Gaussian processes
دانلود مقاله + سفارش ترجمه
دانلود مقاله ISI انگلیسی
رایگان برای ایرانیان
کلمات کلیدی
موضوعات مرتبط
مهندسی و علوم پایه
مهندسی کامپیوتر
نظریه محاسباتی و ریاضیات
پیش نمایش صفحه اول مقاله
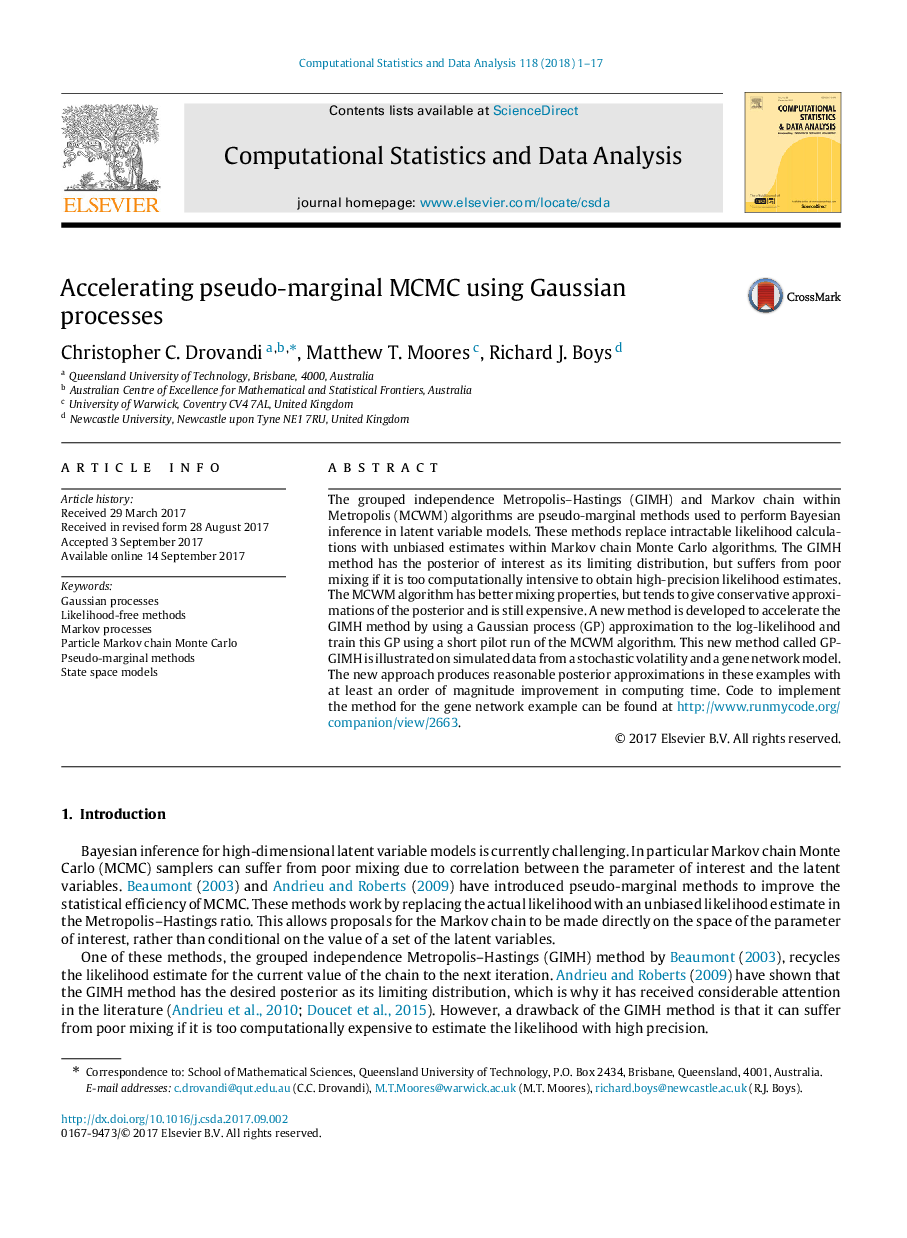
چکیده انگلیسی
The grouped independence Metropolis-Hastings (GIMH) and Markov chain within Metropolis (MCWM) algorithms are pseudo-marginal methods used to perform Bayesian inference in latent variable models. These methods replace intractable likelihood calculations with unbiased estimates within Markov chain Monte Carlo algorithms. The GIMH method has the posterior of interest as its limiting distribution, but suffers from poor mixing if it is too computationally intensive to obtain high-precision likelihood estimates. The MCWM algorithm has better mixing properties, but tends to give conservative approximations of the posterior and is still expensive. A new method is developed to accelerate the GIMH method by using a Gaussian process (GP) approximation to the log-likelihood and train this GP using a short pilot run of the MCWM algorithm. This new method called GP-GIMH is illustrated on simulated data from a stochastic volatility and a gene network model. The new approach produces reasonable posterior approximations in these examples with at least an order of magnitude improvement in computing time. Code to implement the method for the gene network example can be found at http://www.runmycode.org/companion/view/2663.
ناشر
Database: Elsevier - ScienceDirect (ساینس دایرکت)
Journal: Computational Statistics & Data Analysis - Volume 118, February 2018, Pages 1-17
Journal: Computational Statistics & Data Analysis - Volume 118, February 2018, Pages 1-17
نویسندگان
Christopher C. Drovandi, Matthew T. Moores, Richard J. Boys,