کد مقاله | کد نشریه | سال انتشار | مقاله انگلیسی | نسخه تمام متن |
---|---|---|---|---|
4953322 | 1443004 | 2018 | 13 صفحه PDF | دانلود رایگان |
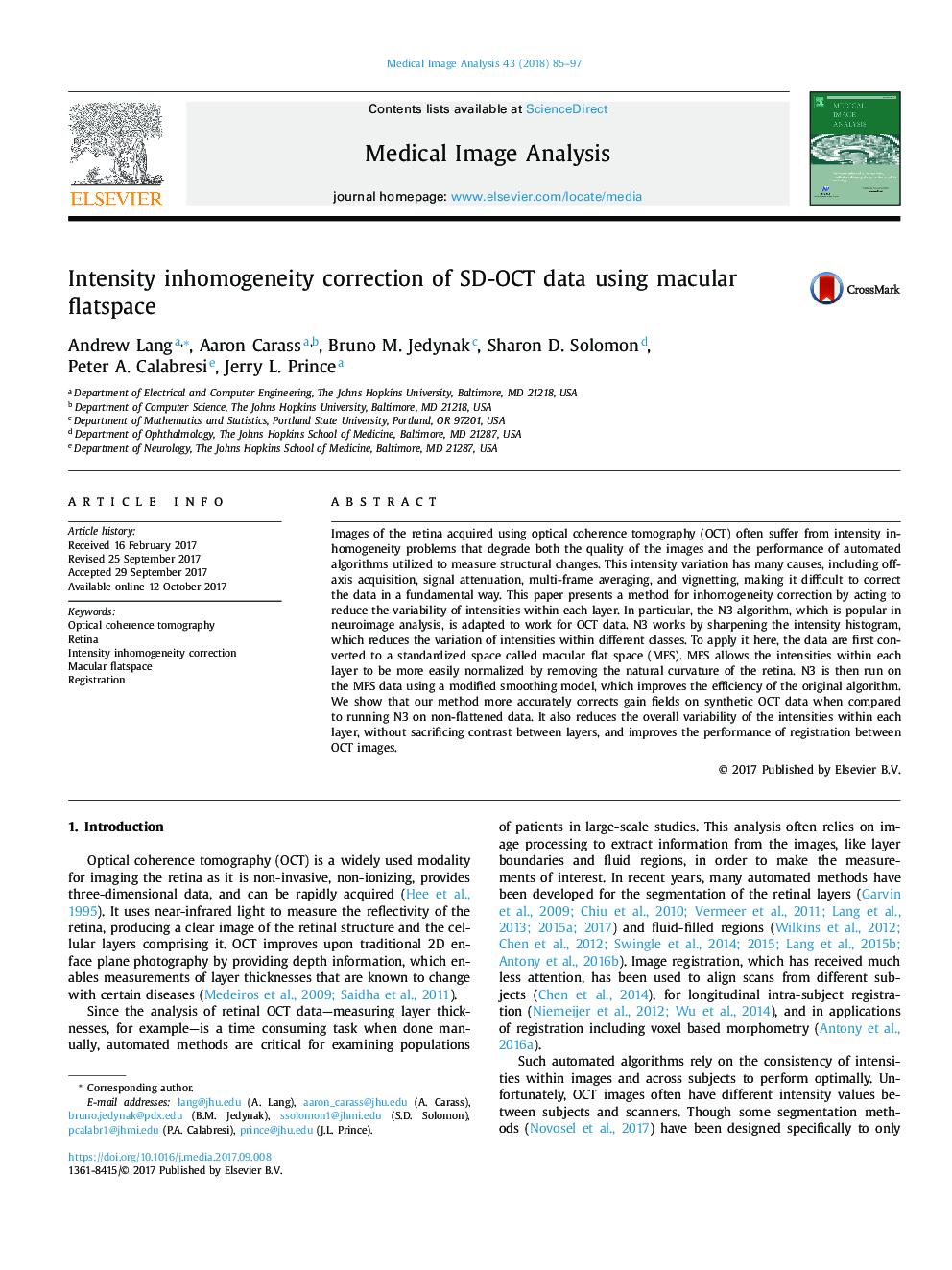
- Optical coherence tomography (OCT) intensity inhomogeneity correction based on image characteristics, which outperforms the physics based models.
- Introduction of macular flat space as a unique computational domain for processing retinal images.
- Improved the performance of the N3 inhomogeneity correction software, which is critically important in neuroimaging.
- A framework for applying synthetic gainfields to OCT images for validating inhomogeneity correction.
- First OCT demonstration of improved registration performance after application of inhomogeneity correction.
Images of the retina acquired using optical coherence tomography (OCT) often suffer from intensity inhomogeneity problems that degrade both the quality of the images and the performance of automated algorithms utilized to measure structural changes. This intensity variation has many causes, including off-axis acquisition, signal attenuation, multi-frame averaging, and vignetting, making it difficult to correct the data in a fundamental way. This paper presents a method for inhomogeneity correction by acting to reduce the variability of intensities within each layer. In particular, the N3 algorithm, which is popular in neuroimage analysis, is adapted to work for OCT data. N3 works by sharpening the intensity histogram, which reduces the variation of intensities within different classes. To apply it here, the data are first converted to a standardized space called macular flat space (MFS). MFS allows the intensities within each layer to be more easily normalized by removing the natural curvature of the retina. N3 is then run on the MFS data using a modified smoothing model, which improves the efficiency of the original algorithm. We show that our method more accurately corrects gain fields on synthetic OCT data when compared to running N3 on non-flattened data. It also reduces the overall variability of the intensities within each layer, without sacrificing contrast between layers, and improves the performance of registration between OCT images.
Journal: Medical Image Analysis - Volume 43, January 2018, Pages 85-97