کد مقاله | کد نشریه | سال انتشار | مقاله انگلیسی | نسخه تمام متن |
---|---|---|---|---|
530737 | 869785 | 2008 | 10 صفحه PDF | دانلود رایگان |
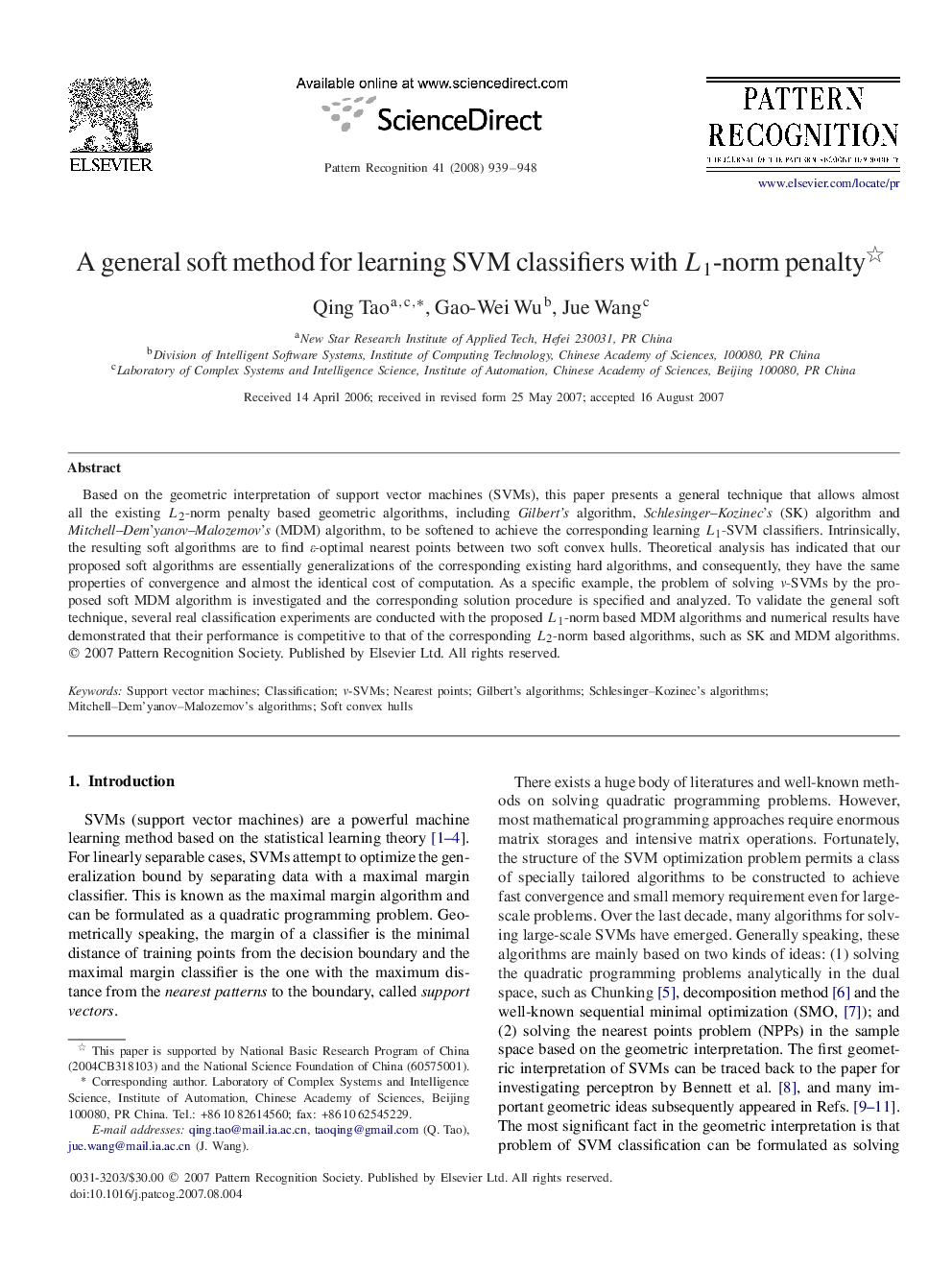
Based on the geometric interpretation of support vector machines (SVMs), this paper presents a general technique that allows almost all the existing L2L2-norm penalty based geometric algorithms, including Gilbert's algorithm, Schlesinger–Kozinec’s (SK) algorithm and Mitchell–Dem’yanov–Malozemov’s (MDM) algorithm, to be softened to achieve the corresponding learning L1L1-SVM classifiers. Intrinsically, the resulting soft algorithms are to find εε-optimal nearest points between two soft convex hulls. Theoretical analysis has indicated that our proposed soft algorithms are essentially generalizations of the corresponding existing hard algorithms, and consequently, they have the same properties of convergence and almost the identical cost of computation. As a specific example, the problem of solving νν-SVMs by the proposed soft MDM algorithm is investigated and the corresponding solution procedure is specified and analyzed. To validate the general soft technique, several real classification experiments are conducted with the proposed L1L1-norm based MDM algorithms and numerical results have demonstrated that their performance is competitive to that of the corresponding L2L2-norm based algorithms, such as SK and MDM algorithms.
Journal: Pattern Recognition - Volume 41, Issue 3, March 2008, Pages 939–948