کد مقاله | کد نشریه | سال انتشار | مقاله انگلیسی | نسخه تمام متن |
---|---|---|---|---|
559100 | 875052 | 2010 | 15 صفحه PDF | دانلود رایگان |
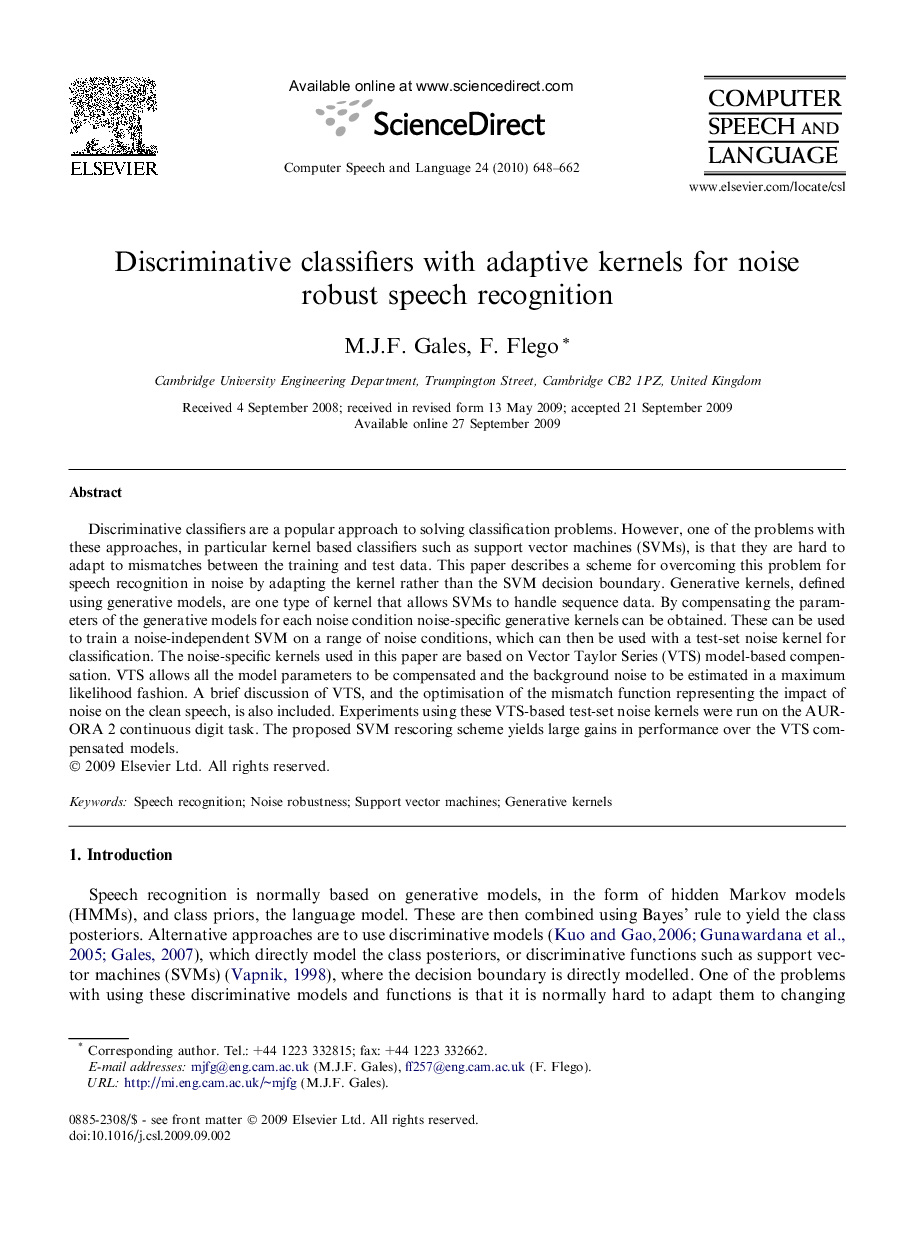
Discriminative classifiers are a popular approach to solving classification problems. However, one of the problems with these approaches, in particular kernel based classifiers such as support vector machines (SVMs), is that they are hard to adapt to mismatches between the training and test data. This paper describes a scheme for overcoming this problem for speech recognition in noise by adapting the kernel rather than the SVM decision boundary. Generative kernels, defined using generative models, are one type of kernel that allows SVMs to handle sequence data. By compensating the parameters of the generative models for each noise condition noise-specific generative kernels can be obtained. These can be used to train a noise-independent SVM on a range of noise conditions, which can then be used with a test-set noise kernel for classification. The noise-specific kernels used in this paper are based on Vector Taylor Series (VTS) model-based compensation. VTS allows all the model parameters to be compensated and the background noise to be estimated in a maximum likelihood fashion. A brief discussion of VTS, and the optimisation of the mismatch function representing the impact of noise on the clean speech, is also included. Experiments using these VTS-based test-set noise kernels were run on the AURORA 2 continuous digit task. The proposed SVM rescoring scheme yields large gains in performance over the VTS compensated models.
Journal: Computer Speech & Language - Volume 24, Issue 4, October 2010, Pages 648–662