کد مقاله | کد نشریه | سال انتشار | مقاله انگلیسی | نسخه تمام متن |
---|---|---|---|---|
5771172 | 1629902 | 2017 | 14 صفحه PDF | دانلود رایگان |
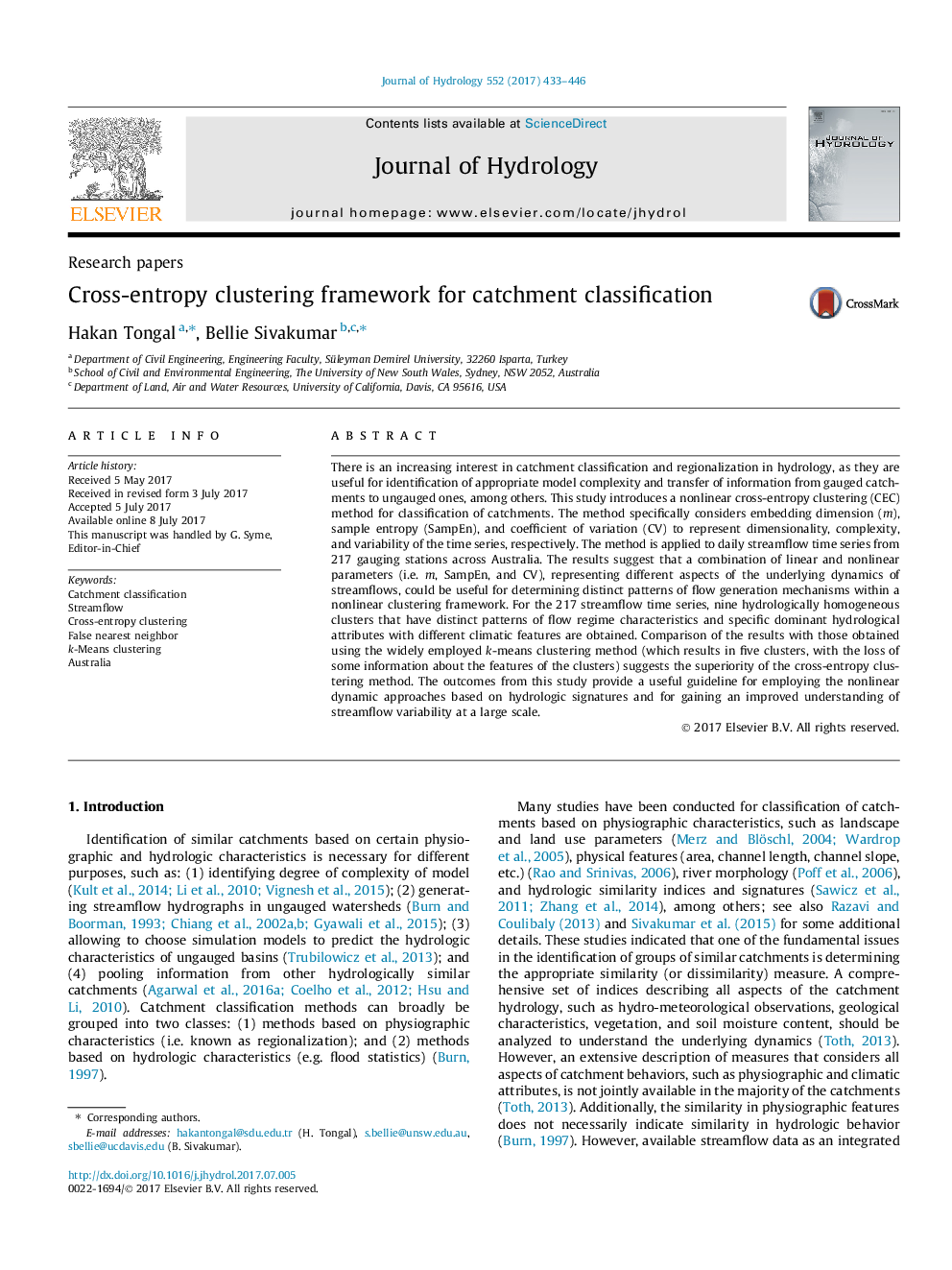
- Cross-entropy clustering method is proposed for catchment classification.
- It considers system dimensionality, complexity, and variability as key properties.
- The method is applied to streamflow data from 217 gauging stations across Australia.
- Nine clusters with distinct patterns of hydro-climatic features are found.
- The cross-entropy clustering is found superior to the k-means clustering method.
There is an increasing interest in catchment classification and regionalization in hydrology, as they are useful for identification of appropriate model complexity and transfer of information from gauged catchments to ungauged ones, among others. This study introduces a nonlinear cross-entropy clustering (CEC) method for classification of catchments. The method specifically considers embedding dimension (m), sample entropy (SampEn), and coefficient of variation (CV) to represent dimensionality, complexity, and variability of the time series, respectively. The method is applied to daily streamflow time series from 217 gauging stations across Australia. The results suggest that a combination of linear and nonlinear parameters (i.e. m, SampEn, and CV), representing different aspects of the underlying dynamics of streamflows, could be useful for determining distinct patterns of flow generation mechanisms within a nonlinear clustering framework. For the 217 streamflow time series, nine hydrologically homogeneous clusters that have distinct patterns of flow regime characteristics and specific dominant hydrological attributes with different climatic features are obtained. Comparison of the results with those obtained using the widely employed k-means clustering method (which results in five clusters, with the loss of some information about the features of the clusters) suggests the superiority of the cross-entropy clustering method. The outcomes from this study provide a useful guideline for employing the nonlinear dynamic approaches based on hydrologic signatures and for gaining an improved understanding of streamflow variability at a large scale.
Journal: Journal of Hydrology - Volume 552, September 2017, Pages 433-446