کد مقاله | کد نشریه | سال انتشار | مقاله انگلیسی | نسخه تمام متن |
---|---|---|---|---|
5856507 | 1131976 | 2015 | 6 صفحه PDF | دانلود رایگان |
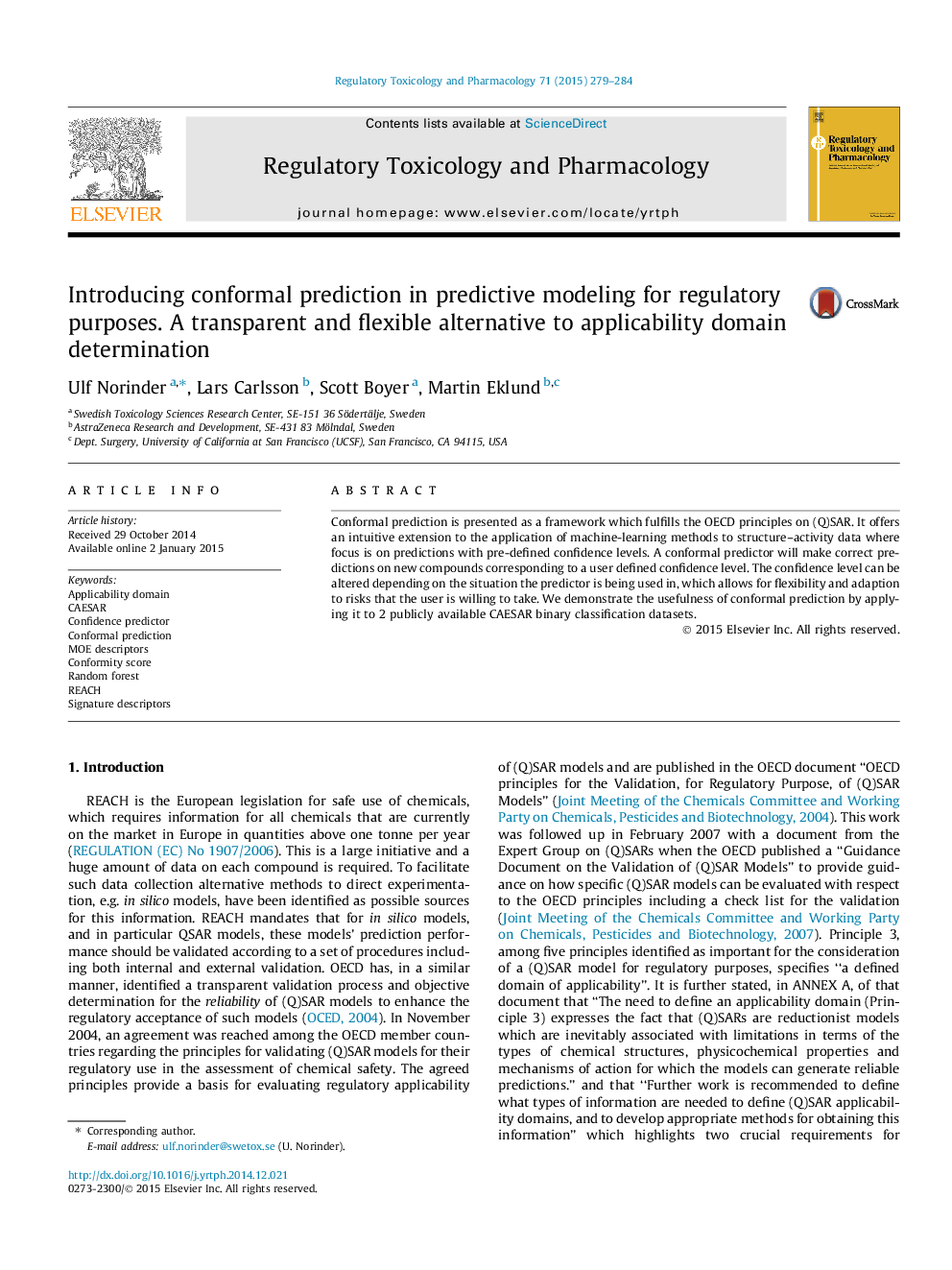
- CAESAR datasets were studied using random forest with MOE and signature descriptors.
- Binary QSAR classification models were constructed using conformal prediction.
- Resulting models are predictive and valid in conformal prediction terms.
- Conformal prediction represents an intuitive extension for reliability estimation.
- The confidence level can be altered depending on acceptable risks.
Conformal prediction is presented as a framework which fulfills the OECD principles on (Q)SAR. It offers an intuitive extension to the application of machine-learning methods to structure-activity data where focus is on predictions with pre-defined confidence levels. A conformal predictor will make correct predictions on new compounds corresponding to a user defined confidence level. The confidence level can be altered depending on the situation the predictor is being used in, which allows for flexibility and adaption to risks that the user is willing to take. We demonstrate the usefulness of conformal prediction by applying it to 2 publicly available CAESAR binary classification datasets.
Journal: Regulatory Toxicology and Pharmacology - Volume 71, Issue 2, March 2015, Pages 279-284