کد مقاله | کد نشریه | سال انتشار | مقاله انگلیسی | نسخه تمام متن |
---|---|---|---|---|
6949560 | 1451276 | 2014 | 12 صفحه PDF | دانلود رایگان |
عنوان انگلیسی مقاله ISI
Evaluating the performance of a new classifier - the GP-OAD: A comparison with existing methods for classifying rock type and mineralogy from hyperspectral imagery
دانلود مقاله + سفارش ترجمه
دانلود مقاله ISI انگلیسی
رایگان برای ایرانیان
کلمات کلیدی
موضوعات مرتبط
مهندسی و علوم پایه
مهندسی کامپیوتر
سیستم های اطلاعاتی
پیش نمایش صفحه اول مقاله
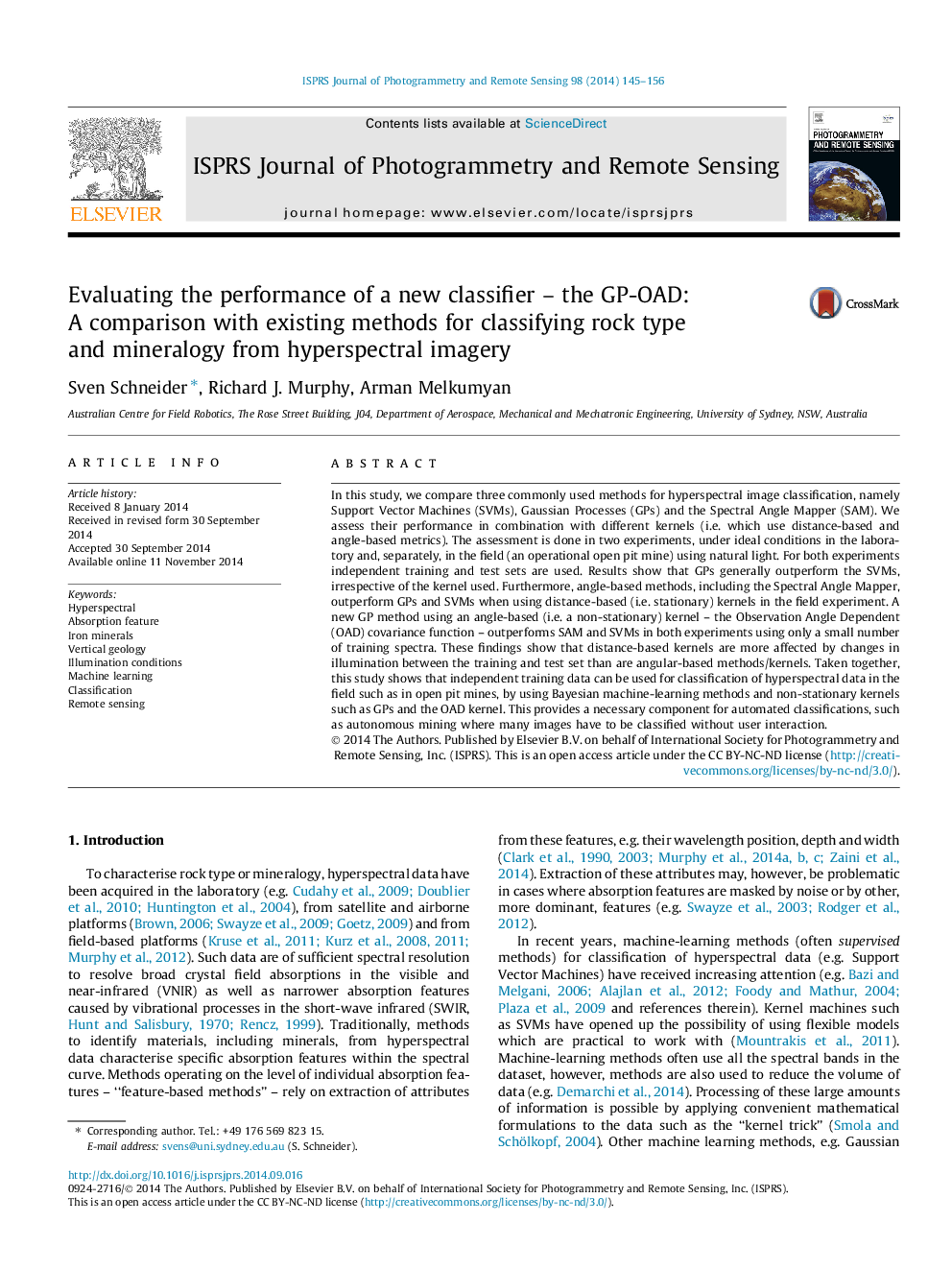
چکیده انگلیسی
In this study, we compare three commonly used methods for hyperspectral image classification, namely Support Vector Machines (SVMs), Gaussian Processes (GPs) and the Spectral Angle Mapper (SAM). We assess their performance in combination with different kernels (i.e. which use distance-based and angle-based metrics). The assessment is done in two experiments, under ideal conditions in the laboratory and, separately, in the field (an operational open pit mine) using natural light. For both experiments independent training and test sets are used. Results show that GPs generally outperform the SVMs, irrespective of the kernel used. Furthermore, angle-based methods, including the Spectral Angle Mapper, outperform GPs and SVMs when using distance-based (i.e. stationary) kernels in the field experiment. A new GP method using an angle-based (i.e. a non-stationary) kernel - the Observation Angle Dependent (OAD) covariance function - outperforms SAM and SVMs in both experiments using only a small number of training spectra. These findings show that distance-based kernels are more affected by changes in illumination between the training and test set than are angular-based methods/kernels. Taken together, this study shows that independent training data can be used for classification of hyperspectral data in the field such as in open pit mines, by using Bayesian machine-learning methods and non-stationary kernels such as GPs and the OAD kernel. This provides a necessary component for automated classifications, such as autonomous mining where many images have to be classified without user interaction.
ناشر
Database: Elsevier - ScienceDirect (ساینس دایرکت)
Journal: ISPRS Journal of Photogrammetry and Remote Sensing - Volume 98, December 2014, Pages 145-156
Journal: ISPRS Journal of Photogrammetry and Remote Sensing - Volume 98, December 2014, Pages 145-156
نویسندگان
Sven Schneider, Richard J. Murphy, Arman Melkumyan,