کد مقاله | کد نشریه | سال انتشار | مقاله انگلیسی | نسخه تمام متن |
---|---|---|---|---|
394860 | 665913 | 2008 | 18 صفحه PDF | دانلود رایگان |
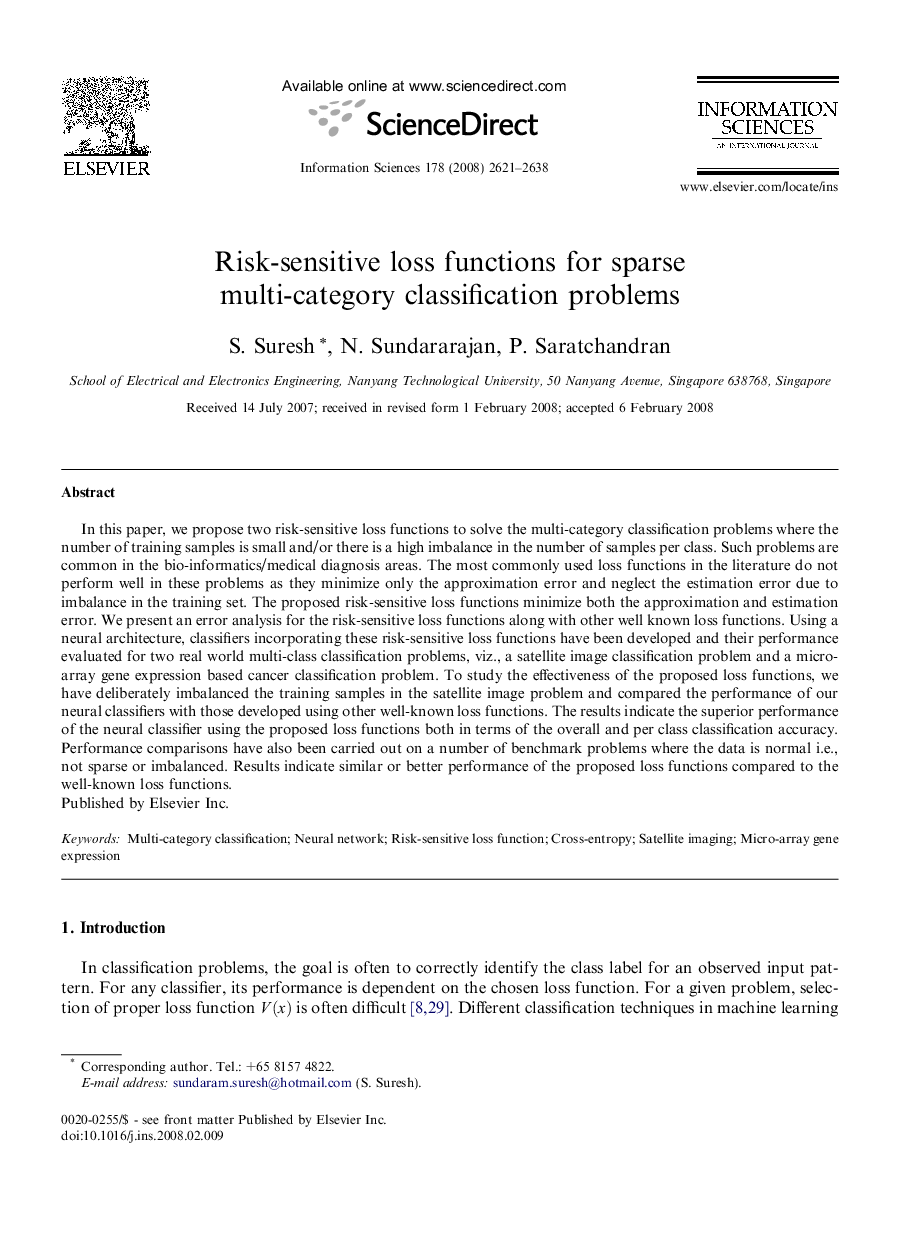
In this paper, we propose two risk-sensitive loss functions to solve the multi-category classification problems where the number of training samples is small and/or there is a high imbalance in the number of samples per class. Such problems are common in the bio-informatics/medical diagnosis areas. The most commonly used loss functions in the literature do not perform well in these problems as they minimize only the approximation error and neglect the estimation error due to imbalance in the training set. The proposed risk-sensitive loss functions minimize both the approximation and estimation error. We present an error analysis for the risk-sensitive loss functions along with other well known loss functions. Using a neural architecture, classifiers incorporating these risk-sensitive loss functions have been developed and their performance evaluated for two real world multi-class classification problems, viz., a satellite image classification problem and a micro-array gene expression based cancer classification problem. To study the effectiveness of the proposed loss functions, we have deliberately imbalanced the training samples in the satellite image problem and compared the performance of our neural classifiers with those developed using other well-known loss functions. The results indicate the superior performance of the neural classifier using the proposed loss functions both in terms of the overall and per class classification accuracy. Performance comparisons have also been carried out on a number of benchmark problems where the data is normal i.e., not sparse or imbalanced. Results indicate similar or better performance of the proposed loss functions compared to the well-known loss functions.
Journal: Information Sciences - Volume 178, Issue 12, 15 June 2008, Pages 2621–2638