کد مقاله | کد نشریه | سال انتشار | مقاله انگلیسی | نسخه تمام متن |
---|---|---|---|---|
4950260 | 1364283 | 2018 | 18 صفحه PDF | دانلود رایگان |
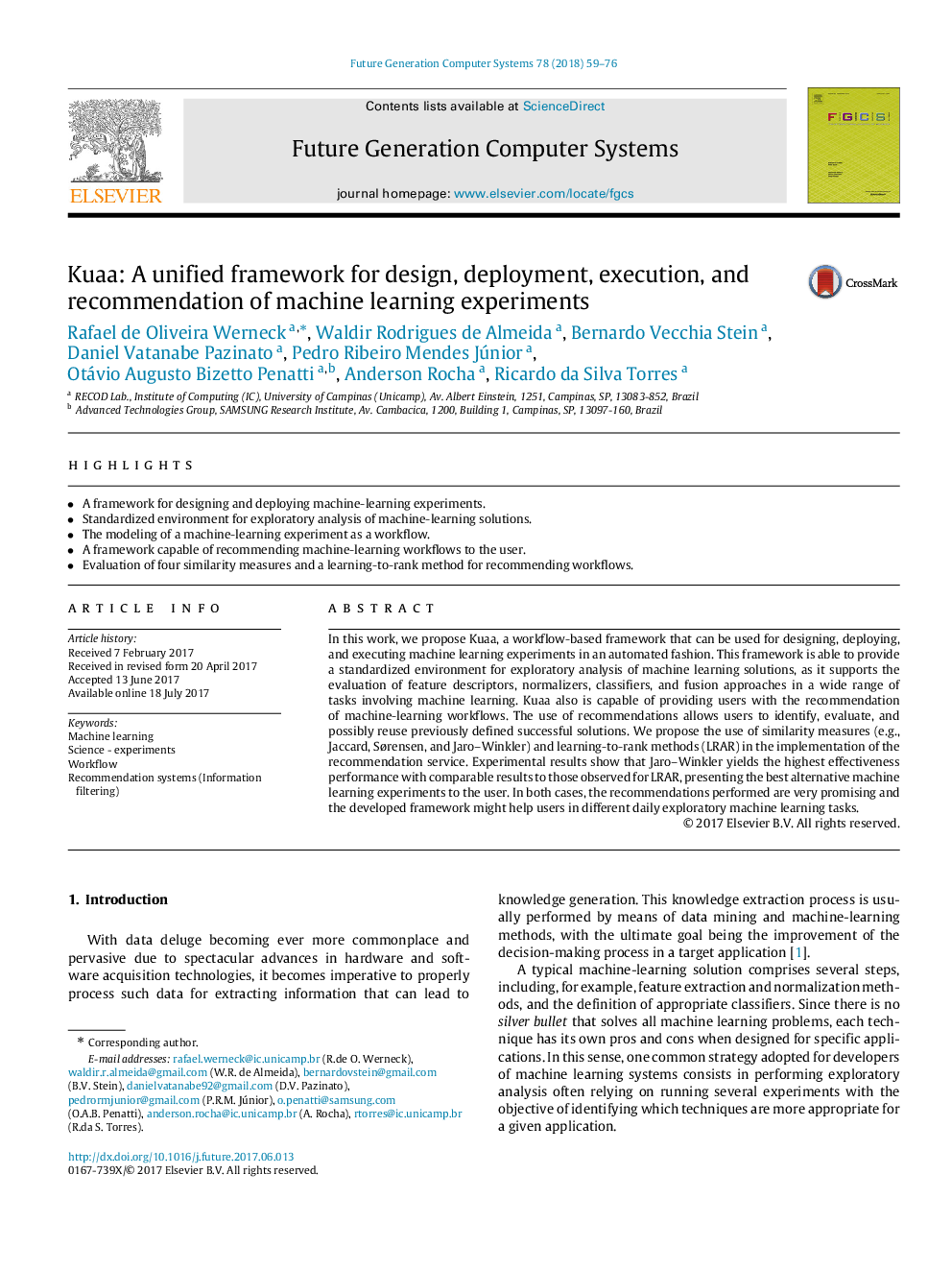
- A framework for designing and deploying machine-learning experiments.
- Standardized environment for exploratory analysis of machine-learning solutions.
- The modeling of a machine-learning experiment as a workflow.
- A framework capable of recommending machine-learning workflows to the user.
- Evaluation of four similarity measures and a learning-to-rank method for recommending workflows.
In this work, we propose Kuaa, a workflow-based framework that can be used for designing, deploying, and executing machine learning experiments in an automated fashion. This framework is able to provide a standardized environment for exploratory analysis of machine learning solutions, as it supports the evaluation of feature descriptors, normalizers, classifiers, and fusion approaches in a wide range of tasks involving machine learning. Kuaa also is capable of providing users with the recommendation of machine-learning workflows. The use of recommendations allows users to identify, evaluate, and possibly reuse previously defined successful solutions. We propose the use of similarity measures (e.g., Jaccard, Sørensen, and Jaro-Winkler) and learning-to-rank methods (LRAR) in the implementation of the recommendation service. Experimental results show that Jaro-Winkler yields the highest effectiveness performance with comparable results to those observed for LRAR, presenting the best alternative machine learning experiments to the user. In both cases, the recommendations performed are very promising and the developed framework might help users in different daily exploratory machine learning tasks.
Journal: Future Generation Computer Systems - Volume 78, Part 1, January 2018, Pages 59-76