کد مقاله | کد نشریه | سال انتشار | مقاله انگلیسی | نسخه تمام متن |
---|---|---|---|---|
532856 | 870007 | 2007 | 8 صفحه PDF | دانلود رایگان |
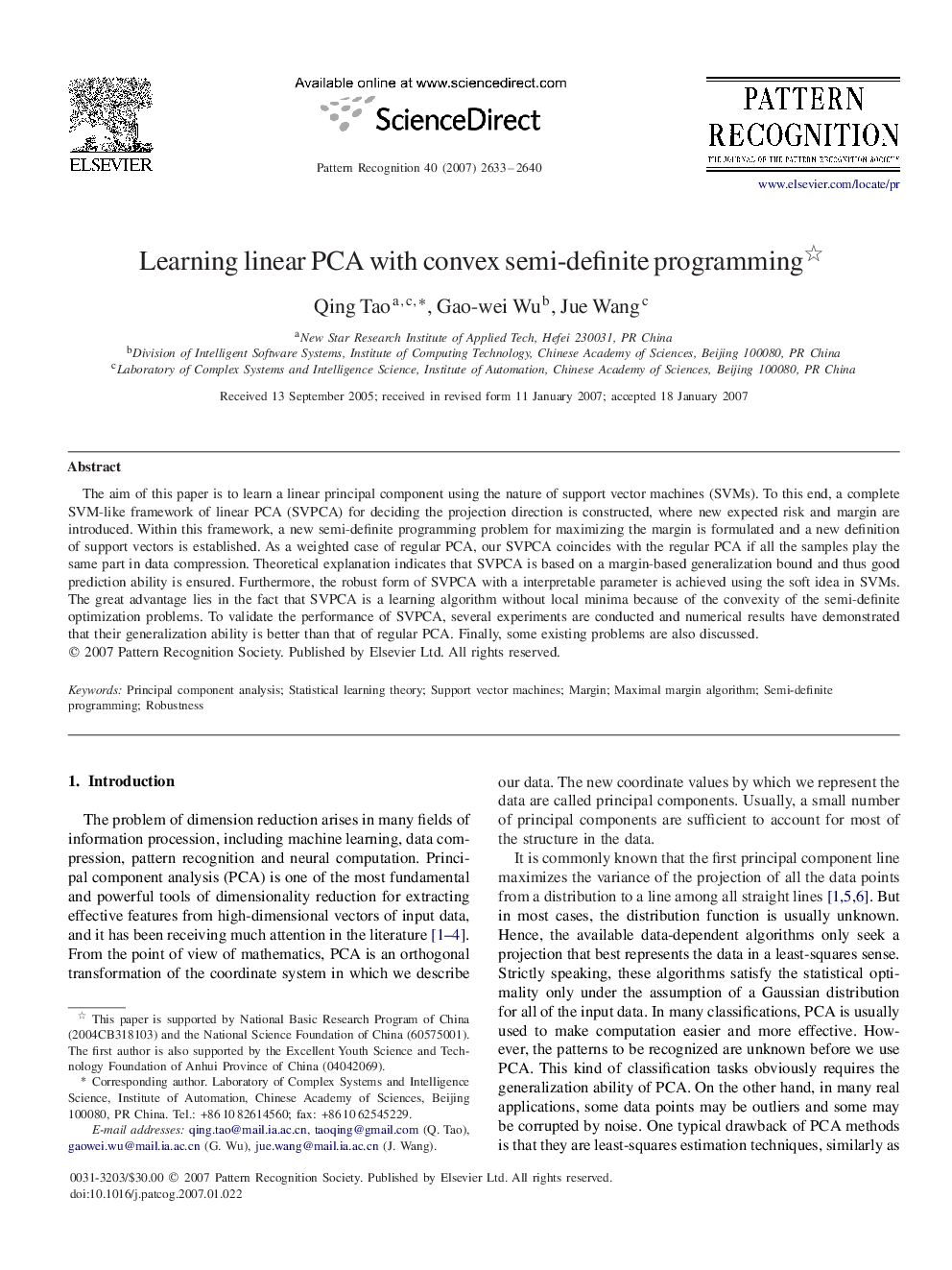
The aim of this paper is to learn a linear principal component using the nature of support vector machines (SVMs). To this end, a complete SVM-like framework of linear PCA (SVPCA) for deciding the projection direction is constructed, where new expected risk and margin are introduced. Within this framework, a new semi-definite programming problem for maximizing the margin is formulated and a new definition of support vectors is established. As a weighted case of regular PCA, our SVPCA coincides with the regular PCA if all the samples play the same part in data compression. Theoretical explanation indicates that SVPCA is based on a margin-based generalization bound and thus good prediction ability is ensured. Furthermore, the robust form of SVPCA with a interpretable parameter is achieved using the soft idea in SVMs. The great advantage lies in the fact that SVPCA is a learning algorithm without local minima because of the convexity of the semi-definite optimization problems. To validate the performance of SVPCA, several experiments are conducted and numerical results have demonstrated that their generalization ability is better than that of regular PCA. Finally, some existing problems are also discussed.
Journal: Pattern Recognition - Volume 40, Issue 10, October 2007, Pages 2633–2640