کد مقاله | کد نشریه | سال انتشار | مقاله انگلیسی | نسخه تمام متن |
---|---|---|---|---|
6412895 | 1629931 | 2014 | 8 صفحه PDF | دانلود رایگان |
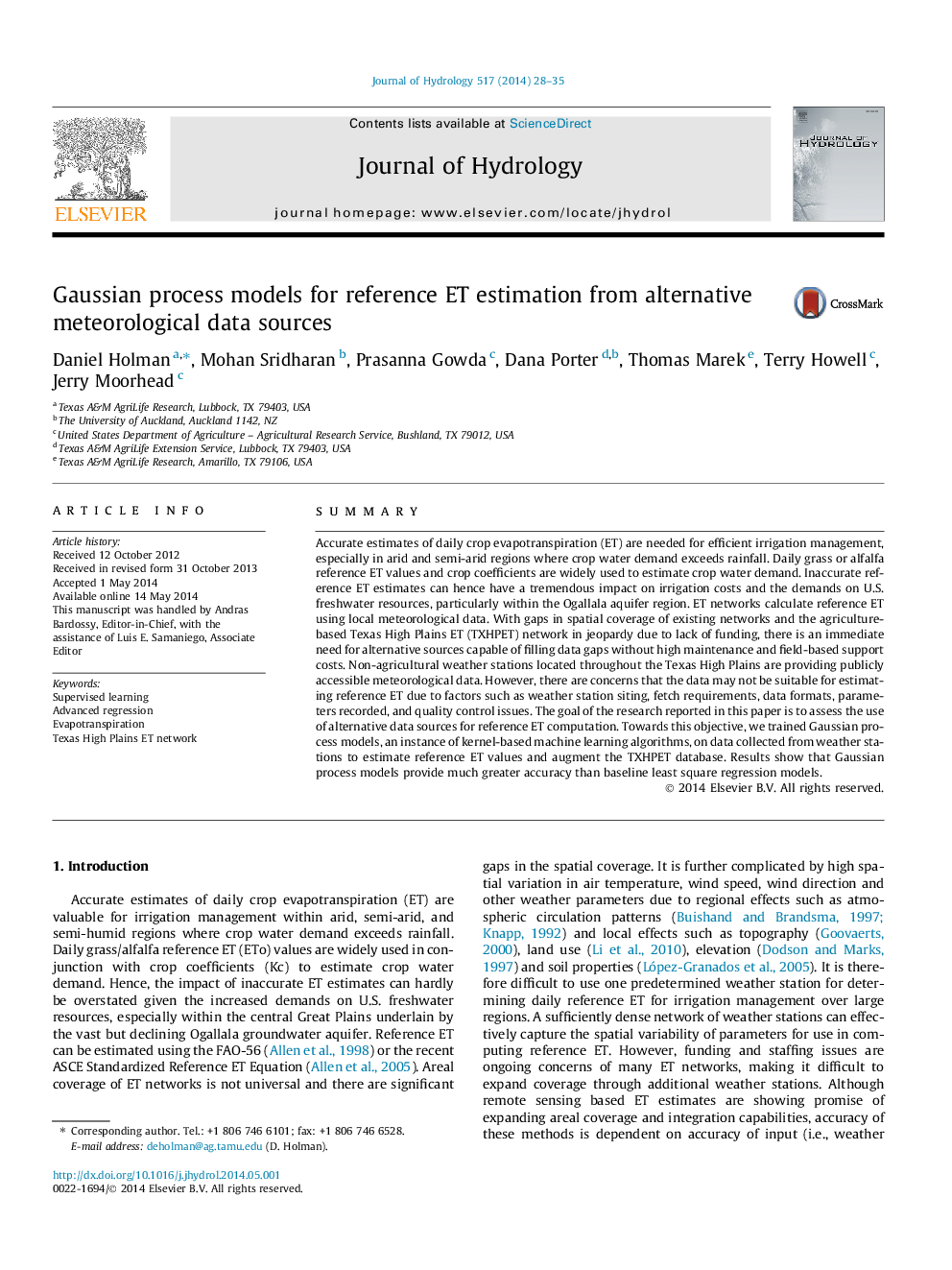
- Accurate estimates of daily ref. ET are needed for efficient irrigation management.
- Climate data from non-ET stations can be used to fill ET for sparse ET-based network.
- GP models performed better than OLS regression models for daily ref. ET estimates.
SummaryAccurate estimates of daily crop evapotranspiration (ET) are needed for efficient irrigation management, especially in arid and semi-arid regions where crop water demand exceeds rainfall. Daily grass or alfalfa reference ET values and crop coefficients are widely used to estimate crop water demand. Inaccurate reference ET estimates can hence have a tremendous impact on irrigation costs and the demands on U.S. freshwater resources, particularly within the Ogallala aquifer region. ET networks calculate reference ET using local meteorological data. With gaps in spatial coverage of existing networks and the agriculture-based Texas High Plains ET (TXHPET) network in jeopardy due to lack of funding, there is an immediate need for alternative sources capable of filling data gaps without high maintenance and field-based support costs. Non-agricultural weather stations located throughout the Texas High Plains are providing publicly accessible meteorological data. However, there are concerns that the data may not be suitable for estimating reference ET due to factors such as weather station siting, fetch requirements, data formats, parameters recorded, and quality control issues. The goal of the research reported in this paper is to assess the use of alternative data sources for reference ET computation. Towards this objective, we trained Gaussian process models, an instance of kernel-based machine learning algorithms, on data collected from weather stations to estimate reference ET values and augment the TXHPET database. Results show that Gaussian process models provide much greater accuracy than baseline least square regression models.
Journal: Journal of Hydrology - Volume 517, 19 September 2014, Pages 28-35