کد مقاله | کد نشریه | سال انتشار | مقاله انگلیسی | نسخه تمام متن |
---|---|---|---|---|
10150999 | 1666104 | 2018 | 39 صفحه PDF | دانلود رایگان |
عنوان انگلیسی مقاله ISI
Rough possibilistic C-means clustering based on multigranulation approximation regions and shadowed sets
دانلود مقاله + سفارش ترجمه
دانلود مقاله ISI انگلیسی
رایگان برای ایرانیان
کلمات کلیدی
موضوعات مرتبط
مهندسی و علوم پایه
مهندسی کامپیوتر
هوش مصنوعی
پیش نمایش صفحه اول مقاله
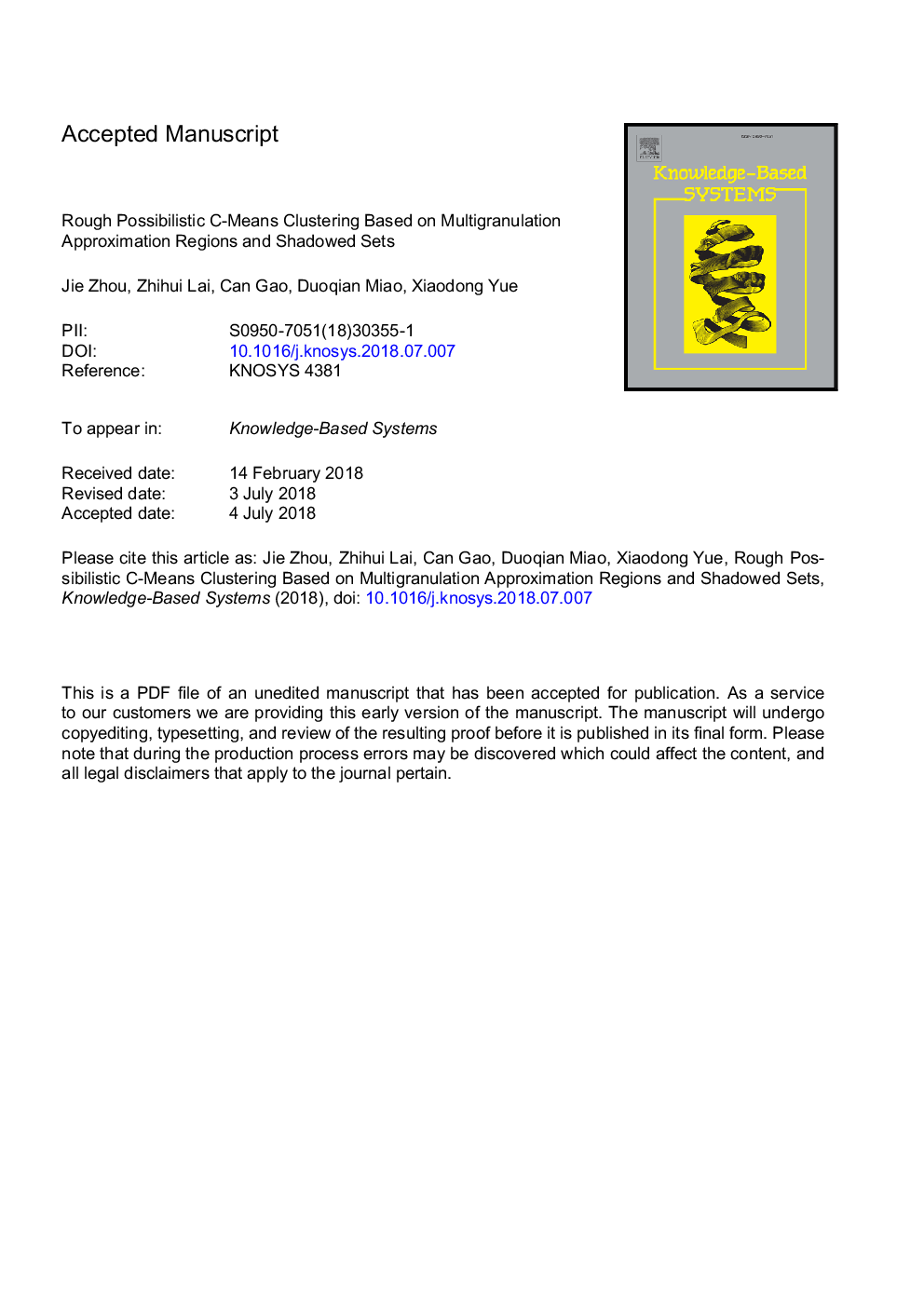
چکیده انگلیسی
The management of uncertain information in a data set is crucial for clustering models. In this study, we present a rough possibilistic C-means clustering approach based on multigranulation approximation regions and shadowed sets, which can handle the uncertainties implicated in data and generated by the model parameters simultaneously. In particular, all patterns are first partitioned into three approximation regions with respect to a fixed cluster according to their possibilistic membership degrees based on shadowed set theory, which can help capture the natural topology of the data, especially when dealing with outliers and noisy data. The multigranulation approximation regions of each cluster can then be formed under a series of fuzzifier values, where the uncertainty caused by a specific fuzzifier value can be detected based on variations in the approximation regions with different levels of granularity. We also introduce a framework for updating prototypes based on ensemble strategies to attenuate the distortions due to iteration during clustering procedures. Finally, an adaptive mechanism is developed for dynamically adjusting scale parameters based on the notion of the maximal compatible regions of clusters. By integrating various granular computing techniques, i.e., rough sets, fuzzy sets, shadowed sets, and the notion of multigranulation, the uncertainties implicated in data and produced by model parameters can be adequately addressed, and the possibilistic membership values involved make the method sufficiently robust to deal with noisy environments. The improved performance of the proposed approach was demonstrated in experiments based on the comparisons with other available fuzzy and possibilistic clustering methods.
ناشر
Database: Elsevier - ScienceDirect (ساینس دایرکت)
Journal: Knowledge-Based Systems - Volume 160, 15 November 2018, Pages 144-166
Journal: Knowledge-Based Systems - Volume 160, 15 November 2018, Pages 144-166
نویسندگان
Jie Zhou, Zhihui Lai, Can Gao, Duoqian Miao, Xiaodong Yue,