کد مقاله | کد نشریه | سال انتشار | مقاله انگلیسی | نسخه تمام متن |
---|---|---|---|---|
10326109 | 677486 | 2005 | 9 صفحه PDF | دانلود رایگان |
عنوان انگلیسی مقاله ISI
Complex-valued neural networks for nonlinear complex principal component analysis
دانلود مقاله + سفارش ترجمه
دانلود مقاله ISI انگلیسی
رایگان برای ایرانیان
موضوعات مرتبط
مهندسی و علوم پایه
مهندسی کامپیوتر
هوش مصنوعی
پیش نمایش صفحه اول مقاله
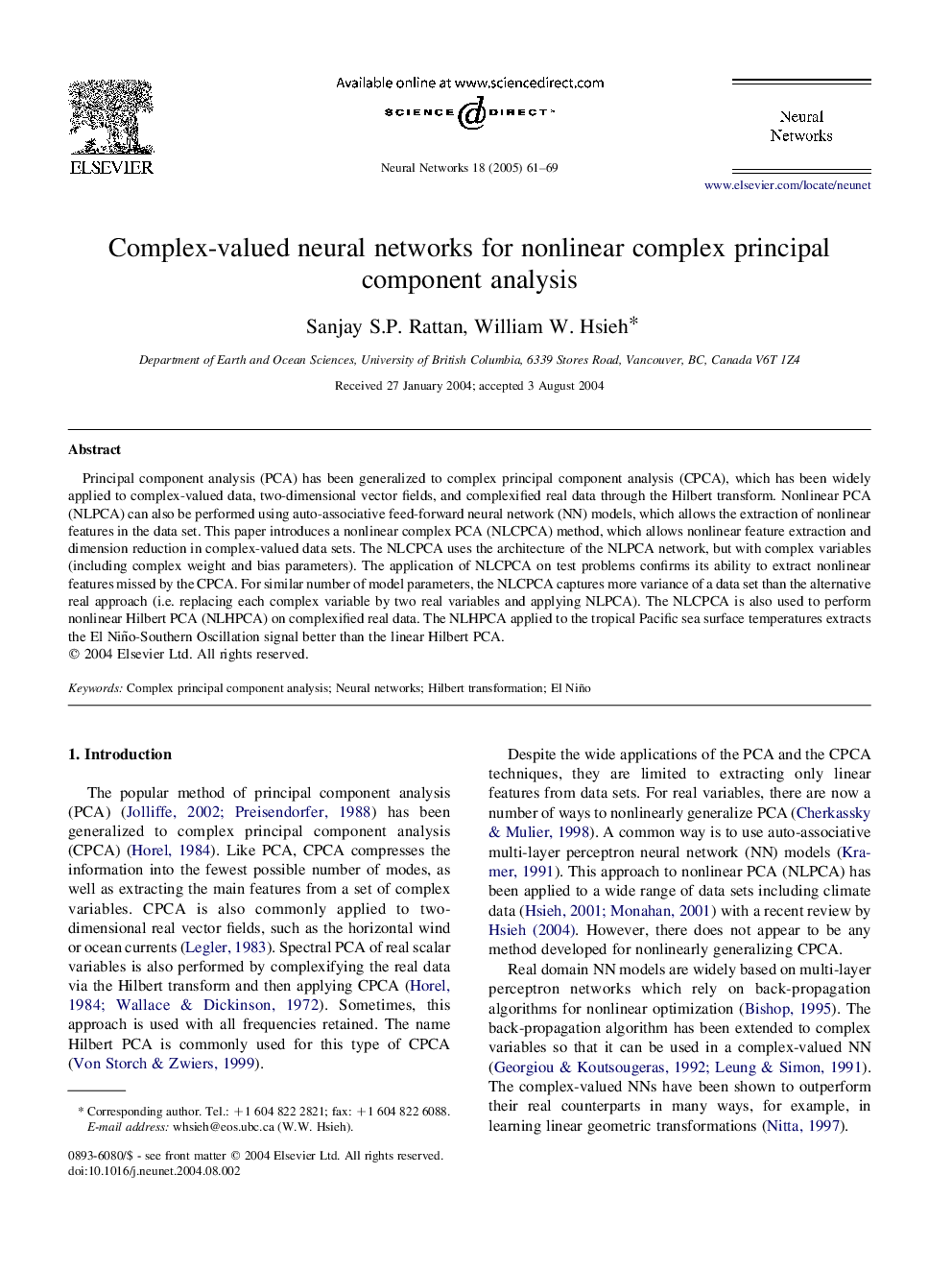
چکیده انگلیسی
Principal component analysis (PCA) has been generalized to complex principal component analysis (CPCA), which has been widely applied to complex-valued data, two-dimensional vector fields, and complexified real data through the Hilbert transform. Nonlinear PCA (NLPCA) can also be performed using auto-associative feed-forward neural network (NN) models, which allows the extraction of nonlinear features in the data set. This paper introduces a nonlinear complex PCA (NLCPCA) method, which allows nonlinear feature extraction and dimension reduction in complex-valued data sets. The NLCPCA uses the architecture of the NLPCA network, but with complex variables (including complex weight and bias parameters). The application of NLCPCA on test problems confirms its ability to extract nonlinear features missed by the CPCA. For similar number of model parameters, the NLCPCA captures more variance of a data set than the alternative real approach (i.e. replacing each complex variable by two real variables and applying NLPCA). The NLCPCA is also used to perform nonlinear Hilbert PCA (NLHPCA) on complexified real data. The NLHPCA applied to the tropical Pacific sea surface temperatures extracts the El Niño-Southern Oscillation signal better than the linear Hilbert PCA.
ناشر
Database: Elsevier - ScienceDirect (ساینس دایرکت)
Journal: Neural Networks - Volume 18, Issue 1, January 2005, Pages 61-69
Journal: Neural Networks - Volume 18, Issue 1, January 2005, Pages 61-69
نویسندگان
Sanjay S.P. Rattan, William W. Hsieh,