کد مقاله | کد نشریه | سال انتشار | مقاله انگلیسی | نسخه تمام متن |
---|---|---|---|---|
10344598 | 697864 | 2012 | 10 صفحه PDF | دانلود رایگان |
عنوان انگلیسی مقاله ISI
Bagging, bumping, multiview, and active learning for record linkage with empirical results on patient identity data
دانلود مقاله + سفارش ترجمه
دانلود مقاله ISI انگلیسی
رایگان برای ایرانیان
کلمات کلیدی
موضوعات مرتبط
مهندسی و علوم پایه
مهندسی کامپیوتر
علوم کامپیوتر (عمومی)
پیش نمایش صفحه اول مقاله
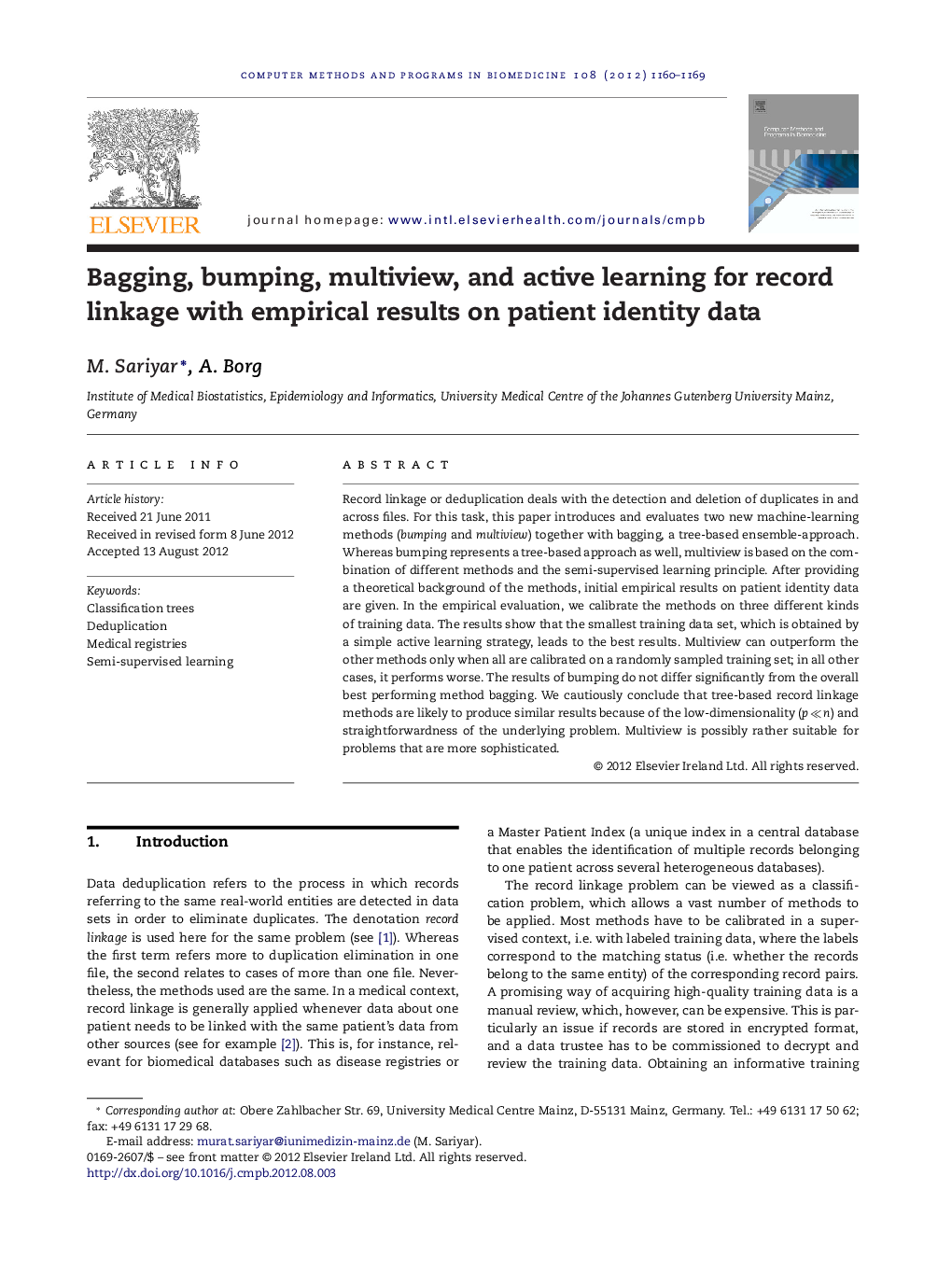
چکیده انگلیسی
Record linkage or deduplication deals with the detection and deletion of duplicates in and across files. For this task, this paper introduces and evaluates two new machine-learning methods (bumping and multiview) together with bagging, a tree-based ensemble-approach. Whereas bumping represents a tree-based approach as well, multiview is based on the combination of different methods and the semi-supervised learning principle. After providing a theoretical background of the methods, initial empirical results on patient identity data are given. In the empirical evaluation, we calibrate the methods on three different kinds of training data. The results show that the smallest training data set, which is obtained by a simple active learning strategy, leads to the best results. Multiview can outperform the other methods only when all are calibrated on a randomly sampled training set; in all other cases, it performs worse. The results of bumping do not differ significantly from the overall best performing method bagging. We cautiously conclude that tree-based record linkage methods are likely to produce similar results because of the low-dimensionality (p âªÂ n) and straightforwardness of the underlying problem. Multiview is possibly rather suitable for problems that are more sophisticated.
ناشر
Database: Elsevier - ScienceDirect (ساینس دایرکت)
Journal: Computer Methods and Programs in Biomedicine - Volume 108, Issue 3, December 2012, Pages 1160-1169
Journal: Computer Methods and Programs in Biomedicine - Volume 108, Issue 3, December 2012, Pages 1160-1169
نویسندگان
M. Sariyar, A. Borg,