کد مقاله | کد نشریه | سال انتشار | مقاله انگلیسی | نسخه تمام متن |
---|---|---|---|---|
10361302 | 870182 | 2005 | 14 صفحه PDF | دانلود رایگان |
عنوان انگلیسی مقاله ISI
Classification methodologies of multilayer perceptrons with sigmoid activation functions
دانلود مقاله + سفارش ترجمه
دانلود مقاله ISI انگلیسی
رایگان برای ایرانیان
کلمات کلیدی
موضوعات مرتبط
مهندسی و علوم پایه
مهندسی کامپیوتر
چشم انداز کامپیوتر و تشخیص الگو
پیش نمایش صفحه اول مقاله
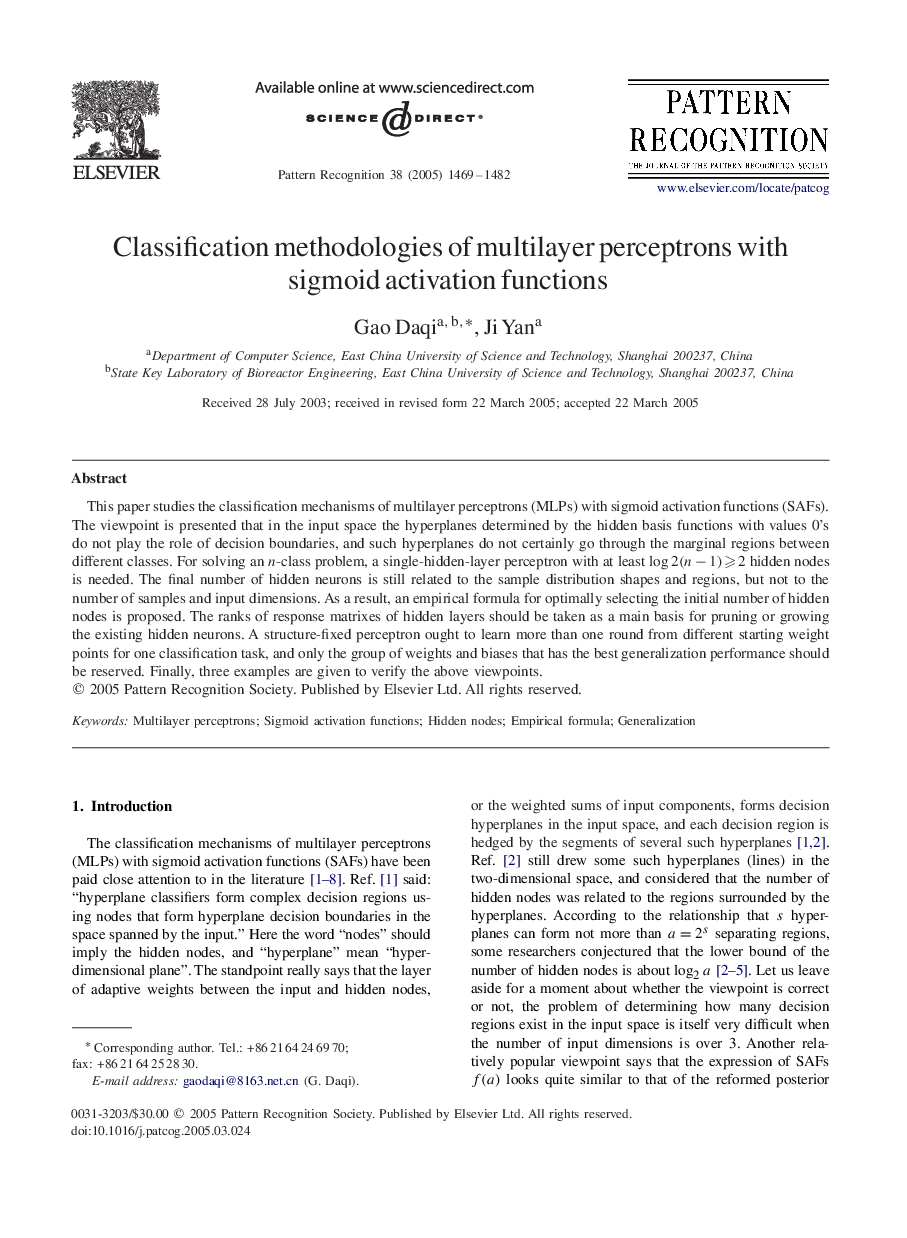
چکیده انگلیسی
This paper studies the classification mechanisms of multilayer perceptrons (MLPs) with sigmoid activation functions (SAFs). The viewpoint is presented that in the input space the hyperplanes determined by the hidden basis functions with values 0's do not play the role of decision boundaries, and such hyperplanes do not certainly go through the marginal regions between different classes. For solving an n-class problem, a single-hidden-layer perceptron with at least log2(n-1)⩾2 hidden nodes is needed. The final number of hidden neurons is still related to the sample distribution shapes and regions, but not to the number of samples and input dimensions. As a result, an empirical formula for optimally selecting the initial number of hidden nodes is proposed. The ranks of response matrixes of hidden layers should be taken as a main basis for pruning or growing the existing hidden neurons. A structure-fixed perceptron ought to learn more than one round from different starting weight points for one classification task, and only the group of weights and biases that has the best generalization performance should be reserved. Finally, three examples are given to verify the above viewpoints.
ناشر
Database: Elsevier - ScienceDirect (ساینس دایرکت)
Journal: Pattern Recognition - Volume 38, Issue 10, October 2005, Pages 1469-1482
Journal: Pattern Recognition - Volume 38, Issue 10, October 2005, Pages 1469-1482
نویسندگان
Gao Daqi, Ji Yan,