کد مقاله | کد نشریه | سال انتشار | مقاله انگلیسی | نسخه تمام متن |
---|---|---|---|---|
10368595 | 874919 | 2015 | 12 صفحه PDF | دانلود رایگان |
عنوان انگلیسی مقاله ISI
Unsupervised language model adaptation using LDA-based mixture models and latent semantic marginals
دانلود مقاله + سفارش ترجمه
دانلود مقاله ISI انگلیسی
رایگان برای ایرانیان
کلمات کلیدی
موضوعات مرتبط
مهندسی و علوم پایه
مهندسی کامپیوتر
پردازش سیگنال
پیش نمایش صفحه اول مقاله
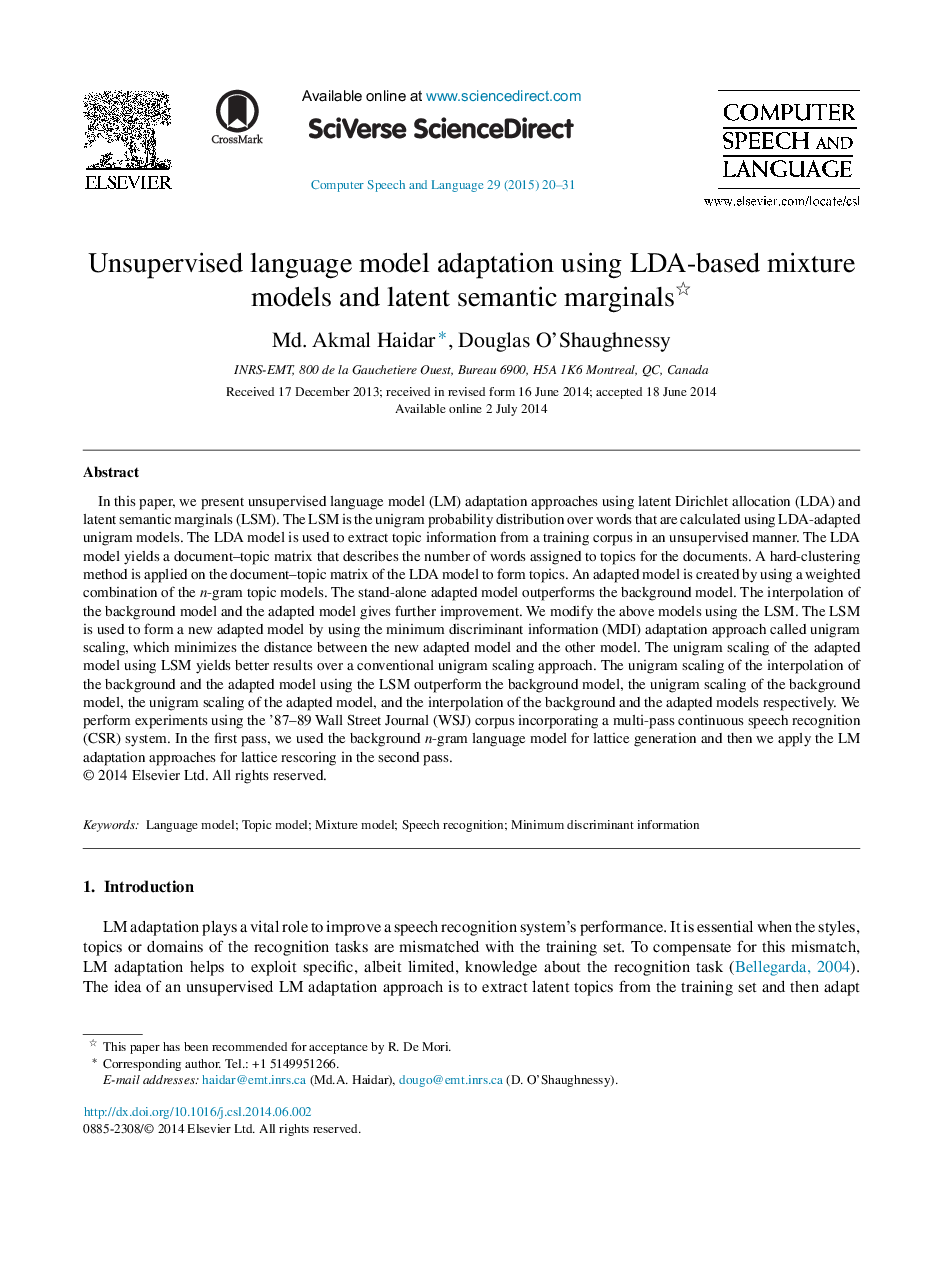
چکیده انگلیسی
In this paper, we present unsupervised language model (LM) adaptation approaches using latent Dirichlet allocation (LDA) and latent semantic marginals (LSM). The LSM is the unigram probability distribution over words that are calculated using LDA-adapted unigram models. The LDA model is used to extract topic information from a training corpus in an unsupervised manner. The LDA model yields a document-topic matrix that describes the number of words assigned to topics for the documents. A hard-clustering method is applied on the document-topic matrix of the LDA model to form topics. An adapted model is created by using a weighted combination of the n-gram topic models. The stand-alone adapted model outperforms the background model. The interpolation of the background model and the adapted model gives further improvement. We modify the above models using the LSM. The LSM is used to form a new adapted model by using the minimum discriminant information (MDI) adaptation approach called unigram scaling, which minimizes the distance between the new adapted model and the other model. The unigram scaling of the adapted model using LSM yields better results over a conventional unigram scaling approach. The unigram scaling of the interpolation of the background and the adapted model using the LSM outperform the background model, the unigram scaling of the background model, the unigram scaling of the adapted model, and the interpolation of the background and the adapted models respectively. We perform experiments using the '87-89 Wall Street Journal (WSJ) corpus incorporating a multi-pass continuous speech recognition (CSR) system. In the first pass, we used the background n-gram language model for lattice generation and then we apply the LM adaptation approaches for lattice rescoring in the second pass.
ناشر
Database: Elsevier - ScienceDirect (ساینس دایرکت)
Journal: Computer Speech & Language - Volume 29, Issue 1, January 2015, Pages 20-31
Journal: Computer Speech & Language - Volume 29, Issue 1, January 2015, Pages 20-31
نویسندگان
Md. Akmal Haidar, Douglas O'Shaughnessy,