کد مقاله | کد نشریه | سال انتشار | مقاله انگلیسی | نسخه تمام متن |
---|---|---|---|---|
11030065 | 1646392 | 2019 | 12 صفحه PDF | دانلود رایگان |
عنوان انگلیسی مقاله ISI
Efficient algorithms for graph regularized PLSA for probabilistic topic modeling
دانلود مقاله + سفارش ترجمه
دانلود مقاله ISI انگلیسی
رایگان برای ایرانیان
کلمات کلیدی
موضوعات مرتبط
مهندسی و علوم پایه
مهندسی کامپیوتر
چشم انداز کامپیوتر و تشخیص الگو
پیش نمایش صفحه اول مقاله
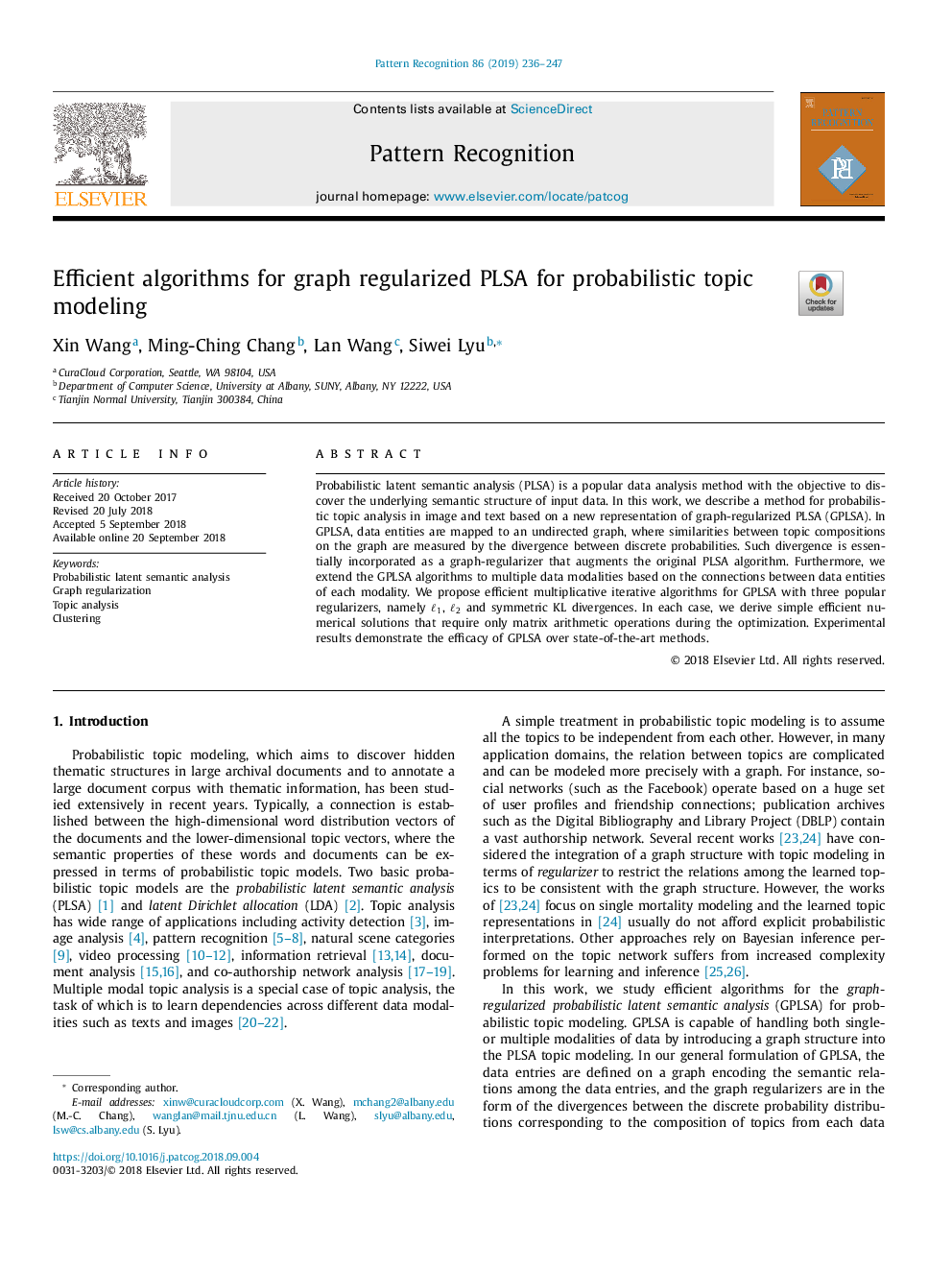
چکیده انگلیسی
Probabilistic latent semantic analysis (PLSA) is a popular data analysis method with the objective to discover the underlying semantic structure of input data. In this work, we describe a method for probabilistic topic analysis in image and text based on a new representation of graph-regularized PLSA (GPLSA). In GPLSA, data entities are mapped to an undirected graph, where similarities between topic compositions on the graph are measured by the divergence between discrete probabilities. Such divergence is essentially incorporated as a graph-regularizer that augments the original PLSA algorithm. Furthermore, we extend the GPLSA algorithms to multiple data modalities based on the connections between data entities of each modality. We propose efficient multiplicative iterative algorithms for GPLSA with three popular regularizers, namely â1, â2 and symmetric KL divergences. In each case, we derive simple efficient numerical solutions that require only matrix arithmetic operations during the optimization. Experimental results demonstrate the efficacy of GPLSA over state-of-the-art methods.
ناشر
Database: Elsevier - ScienceDirect (ساینس دایرکت)
Journal: Pattern Recognition - Volume 86, February 2019, Pages 236-247
Journal: Pattern Recognition - Volume 86, February 2019, Pages 236-247
نویسندگان
Xin Wang, Ming-Ching Chang, Lan Wang, Siwei Lyu,