کد مقاله | کد نشریه | سال انتشار | مقاله انگلیسی | نسخه تمام متن |
---|---|---|---|---|
11031706 | 1645932 | 2019 | 17 صفحه PDF | دانلود رایگان |
عنوان انگلیسی مقاله ISI
Quality variable prediction for chemical processes based on semisupervised Dirichlet process mixture of Gaussians
دانلود مقاله + سفارش ترجمه
دانلود مقاله ISI انگلیسی
رایگان برای ایرانیان
کلمات کلیدی
موضوعات مرتبط
مهندسی و علوم پایه
مهندسی شیمی
مهندسی شیمی (عمومی)
پیش نمایش صفحه اول مقاله
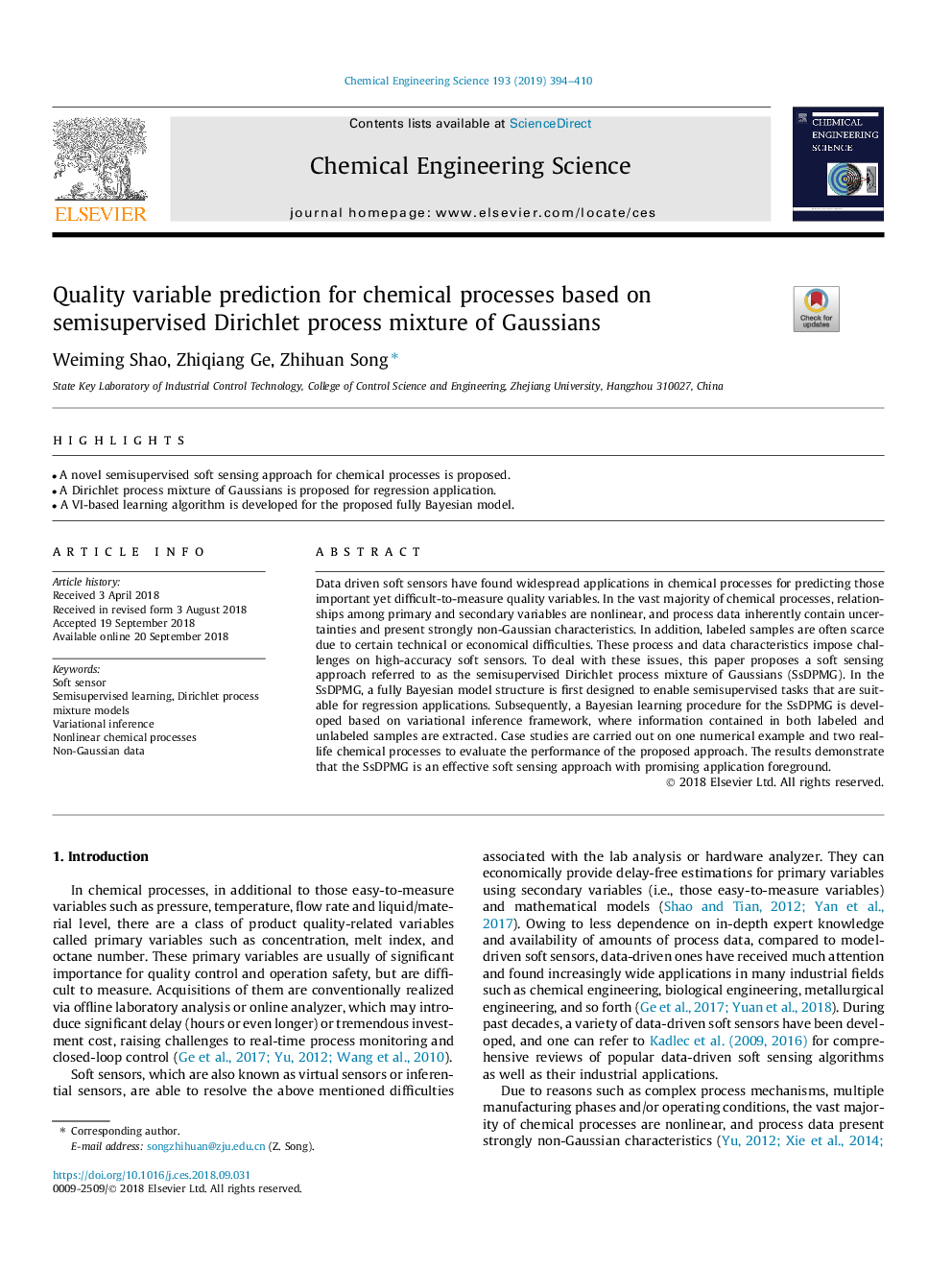
چکیده انگلیسی
Data driven soft sensors have found widespread applications in chemical processes for predicting those important yet difficult-to-measure quality variables. In the vast majority of chemical processes, relationships among primary and secondary variables are nonlinear, and process data inherently contain uncertainties and present strongly non-Gaussian characteristics. In addition, labeled samples are often scarce due to certain technical or economical difficulties. These process and data characteristics impose challenges on high-accuracy soft sensors. To deal with these issues, this paper proposes a soft sensing approach referred to as the semisupervised Dirichlet process mixture of Gaussians (SsDPMG). In the SsDPMG, a fully Bayesian model structure is first designed to enable semisupervised tasks that are suitable for regression applications. Subsequently, a Bayesian learning procedure for the SsDPMG is developed based on variational inference framework, where information contained in both labeled and unlabeled samples are extracted. Case studies are carried out on one numerical example and two real-life chemical processes to evaluate the performance of the proposed approach. The results demonstrate that the SsDPMG is an effective soft sensing approach with promising application foreground.
ناشر
Database: Elsevier - ScienceDirect (ساینس دایرکت)
Journal: Chemical Engineering Science - Volume 193, 16 January 2019, Pages 394-410
Journal: Chemical Engineering Science - Volume 193, 16 January 2019, Pages 394-410
نویسندگان
Weiming Shao, Zhiqiang Ge, Zhihuan Song,