کد مقاله | کد نشریه | سال انتشار | مقاله انگلیسی | نسخه تمام متن |
---|---|---|---|---|
1132254 | 955767 | 2011 | 14 صفحه PDF | دانلود رایگان |
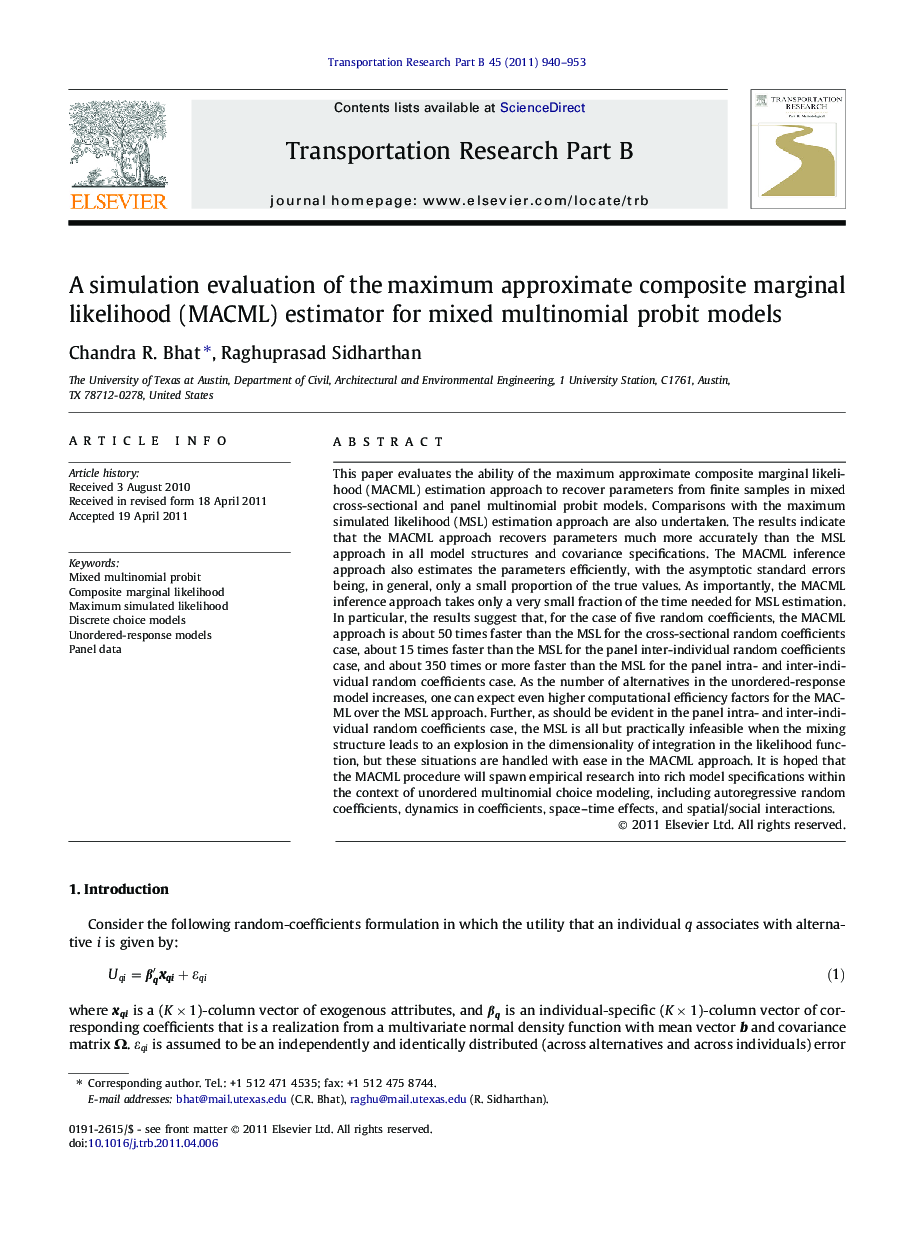
This paper evaluates the ability of the maximum approximate composite marginal likelihood (MACML) estimation approach to recover parameters from finite samples in mixed cross-sectional and panel multinomial probit models. Comparisons with the maximum simulated likelihood (MSL) estimation approach are also undertaken. The results indicate that the MACML approach recovers parameters much more accurately than the MSL approach in all model structures and covariance specifications. The MACML inference approach also estimates the parameters efficiently, with the asymptotic standard errors being, in general, only a small proportion of the true values. As importantly, the MACML inference approach takes only a very small fraction of the time needed for MSL estimation. In particular, the results suggest that, for the case of five random coefficients, the MACML approach is about 50 times faster than the MSL for the cross-sectional random coefficients case, about 15 times faster than the MSL for the panel inter-individual random coefficients case, and about 350 times or more faster than the MSL for the panel intra- and inter-individual random coefficients case. As the number of alternatives in the unordered-response model increases, one can expect even higher computational efficiency factors for the MACML over the MSL approach. Further, as should be evident in the panel intra- and inter-individual random coefficients case, the MSL is all but practically infeasible when the mixing structure leads to an explosion in the dimensionality of integration in the likelihood function, but these situations are handled with ease in the MACML approach. It is hoped that the MACML procedure will spawn empirical research into rich model specifications within the context of unordered multinomial choice modeling, including autoregressive random coefficients, dynamics in coefficients, space–time effects, and spatial/social interactions.
► The MACML estimator recovers parameters well in multinomial probit model structures.
► The MACML estimator is very fast relative to the MSL estimator.
► Rich model specifications can be easily estimated using the MACML approach.
Journal: Transportation Research Part B: Methodological - Volume 45, Issue 7, August 2011, Pages 940–953