کد مقاله | کد نشریه | سال انتشار | مقاله انگلیسی | نسخه تمام متن |
---|---|---|---|---|
1153113 | 1489811 | 2016 | 17 صفحه PDF | دانلود رایگان |
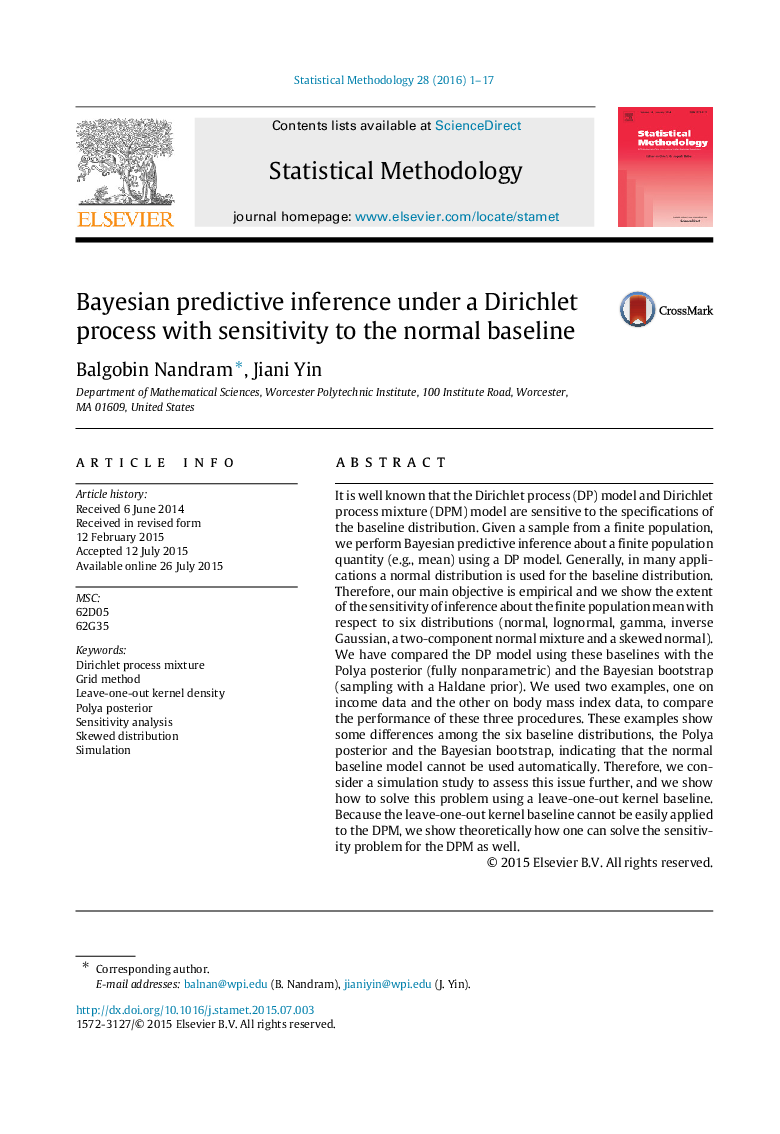
It is well known that the Dirichlet process (DP) model and Dirichlet process mixture (DPM) model are sensitive to the specifications of the baseline distribution. Given a sample from a finite population, we perform Bayesian predictive inference about a finite population quantity (e.g., mean) using a DP model. Generally, in many applications a normal distribution is used for the baseline distribution. Therefore, our main objective is empirical and we show the extent of the sensitivity of inference about the finite population mean with respect to six distributions (normal, lognormal, gamma, inverse Gaussian, a two-component normal mixture and a skewed normal). We have compared the DP model using these baselines with the Polya posterior (fully nonparametric) and the Bayesian bootstrap (sampling with a Haldane prior). We used two examples, one on income data and the other on body mass index data, to compare the performance of these three procedures. These examples show some differences among the six baseline distributions, the Polya posterior and the Bayesian bootstrap, indicating that the normal baseline model cannot be used automatically. Therefore, we consider a simulation study to assess this issue further, and we show how to solve this problem using a leave-one-out kernel baseline. Because the leave-one-out kernel baseline cannot be easily applied to the DPM, we show theoretically how one can solve the sensitivity problem for the DPM as well.
Journal: Statistical Methodology - Volume 28, January 2016, Pages 1–17